Spatio-temporal Analysis and Modeling of Coastal areas for Water Salinity Prediction
2023 IEEE International Students' Conference on Electrical, Electronics and Computer Science (SCEECS)(2023)
摘要
Salinity is an important parameter affecting the quality of water, and excessive amounts adversely affect vege-tation growth and aquatic organism populations. Natural factors like tidal waves, natural calamities etc., and man-made factors like unchecked disposal of industrial wastes, domestic/ urban sewage, and fish hatchery activities can cause significant increases in water salinity. In this article, an approach that utilizes multimodal data like Landsat 8 optical observations and the SMAP salinity data product for predicting water salinity indices in the coastal region is proposed. Machine Learning models such as K-nearest neighbor (KNN), Gradient Boost (GB), Extremely Randomized Tree (ERT), Random Forest Regression (RFR), Decision Tree (DT), Multiple Linear Regression (MLR), Lasso Regression (LR), and Ridge Regression (RR) are used for salinity prediction. Empirical experiments revealed that the ERT model outperformed other ML models, with a
$R^{2}$
of 0.92 and RMSE of 0.25 psu.
更多查看译文
关键词
Water salinity prediction,Remote sensing,Spatio-temporal analysis,Machine Learning,Landsat 8,SMAP
AI 理解论文
溯源树
样例
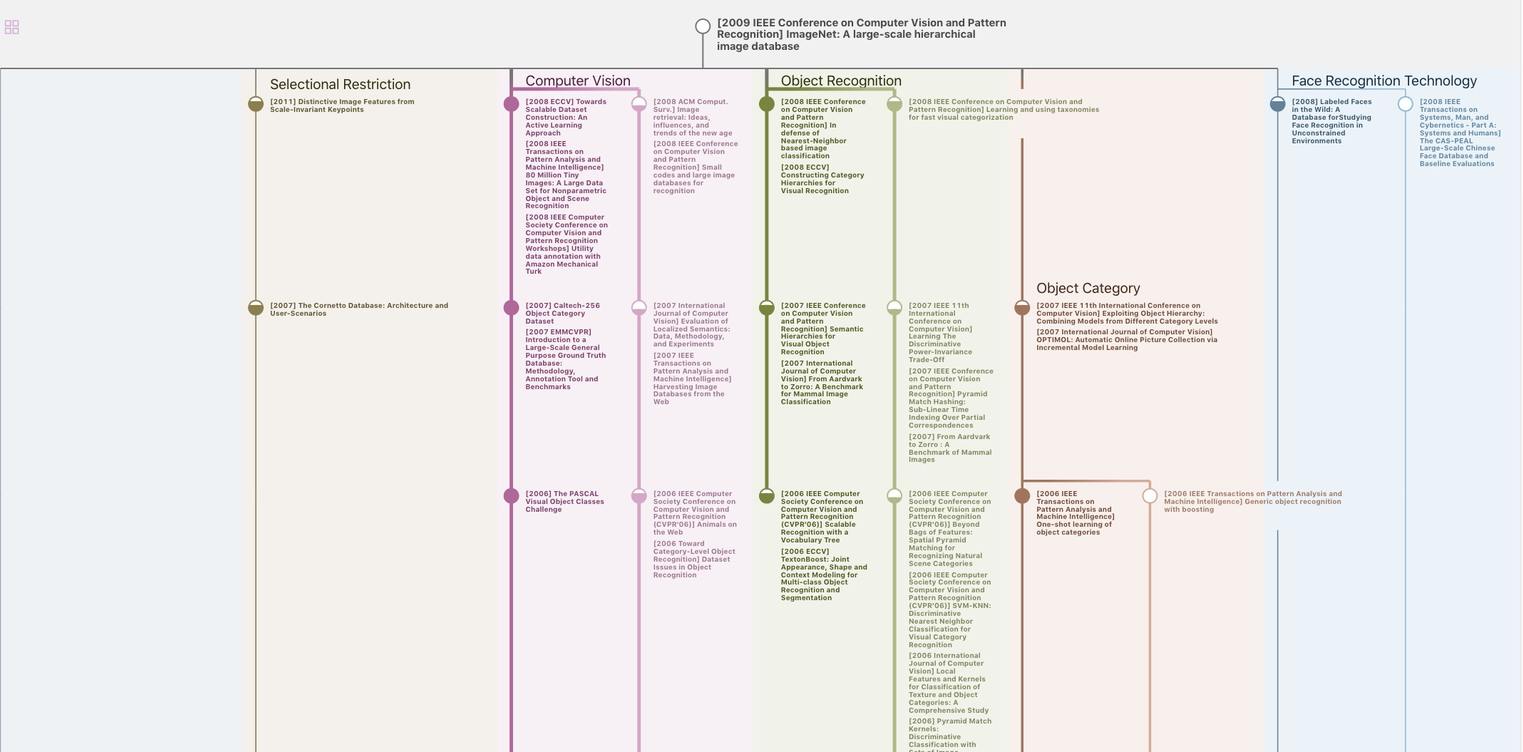
生成溯源树,研究论文发展脉络
Chat Paper
正在生成论文摘要