A $1.5\mu\mathrm{W}$ End-to-End Keyword Spotting SoC with Content-Adaptive Frame Sub-Sampling and Fast-Settling Analog Frontend
2023 IEEE International Solid-State Circuits Conference (ISSCC)(2023)
摘要
Keyword spotting (KWS) has become essential as a wake-up mechanism for edge loT devices. While recent advances in deep learning have improved KWS accuracy [1], reducing system power consumption remains a challenge. A typical KWS signal chain consists of an analog frontend (AFE), feature extractor (FE), and neural network classifier (NN). To reduce total KWS power, all three blocks must be carefully co-optimized. Recent KWS work reported 0.51
$\mu \mathrm{W}$
consumption for the FE and NN, but it only supports two keywords and lacks an AFE, whose power often dominates [2]. A KWS system including an AFE was also proposed but consumes
$16\mu \mathrm{W}$
[3]. This work proposes a fully integrated keyword spotting system that employs the skip RNN algorithm [4] to simultaneously reduce the power consumption of the AFE, FE, and NN by adaptively sub-sampling (i.e., skipping) input frames based on the signal content. The skip RNN continually decides whether the RNN state is to be updated or skipped for one or more 16ms-long frames based on its input content history, reducing NN operation and hence power. We further propose a scheme to use the NN skip decision to dynamically turn off the AFE and FE, which dominate the KWS power (combined
$> 65{\%}$
) for one or more consecutive frames, achieving
$3\times$
power reduction. The proposed AFE features a programmable switched capacitor resistor and two-step switching frequency control, demonstrating less than 1ms settling time. The FE and NN employ computational sprinting with efficient scheduling to reduce their operation time and static current. The proposed KWS system consumes
$1.5\mu \mathrm{W}$
(reduced from
$4.47\mu \mathrm{W}$
with an average of 76% frame skipping) in 28nm CMOS, while achieving 92.8% accuracy on five-word GSCD KWS.
更多查看译文
关键词
AFE,analog frontend,CMOS,consecutive frames,content-adaptive frame sub-sampling,deep learning,edge loT devices,end-to-end keyword spotting SoC,fast-settling analog frontend,fast-settling analog,feature extractor,five-word GSCD KWS,fully integrated keyword spotting system,input content history,input frames,KWS accuracy,KWS system,neural network classifier,NN skip decision,power 0.51 muW,power 1.5 muW,power 16 muW,power 4.47 muW,programmable switched capacitor resistor,RNN state,signal content,size 28.0 nm,skip RNN,system power consumption,two-step switching frequency control,typical KWS signal chain,wake-up mechanism
AI 理解论文
溯源树
样例
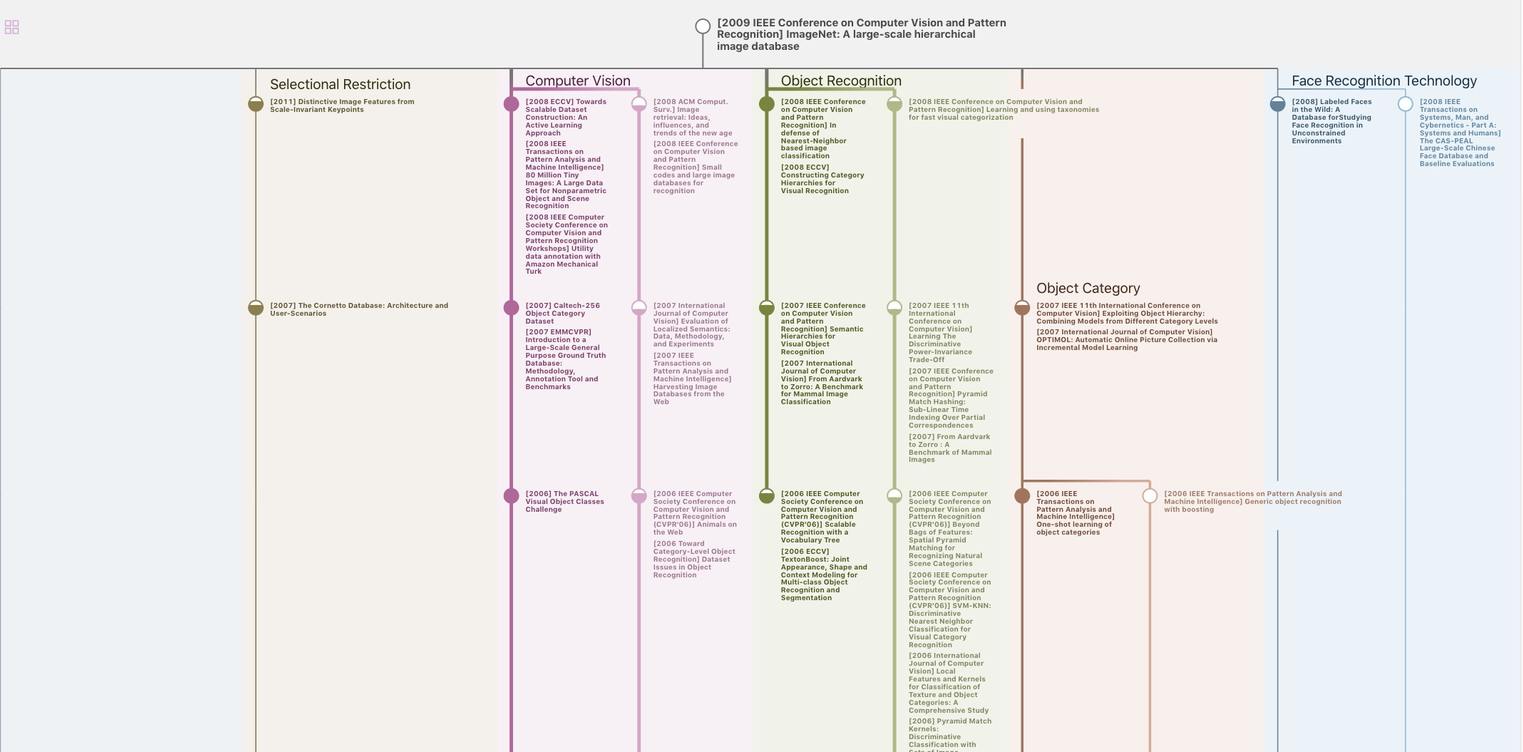
生成溯源树,研究论文发展脉络
Chat Paper
正在生成论文摘要