A Configurable On-Chip Spike Encoding Network Based on Dual-Mode Integrate & Fire Neurons
2023 IEEE 6th Information Technology,Networking,Electronic and Automation Control Conference (ITNEC)(2023)
Abstract
For edge intelligence applications, this work proposes a tiny spike encoding network embedded with high-speed on-chip encoding capability, which applies the proposed dual-mode Integrate & Fire (IF) neuron model to support different coding schemes. The proposed encoding network was prototyped on a Zynq-7020 FPGA device, with an on-chip encoding speed as high as 2127 frame/s, while dissipating only 69 mW under a 250 MHz clock frequency. Our spiking neural network hardware encoder adopts an eight-core architecture for parallel computing to improve processing speed, supporting three well-known coding schemes, i.e. rate coding, burst coding and time-to-firstspike (TTFS) coding. To verify the performance of different coding schemes realized by our hardware encoder, the widely used MNIST and Fashion-MNIST datasets were selected as benchmark. After encoding, all spiking addressevent representation (AER) data were sent to a two-layer fully connected network with BP-STDP learning rule for training and inference, which was completed on PC software. Finally, three coding schemes (rate coding, burst coding and time-to-first-spike coding) all achieved comparably high classification accuracies on MNIST and Fashion-MNIST datasets (95.87%, 92.23% and 88.22% on MNIST, 83.79%, 82.71% and 73.79% on Fashion-MNIST, respectively).
MoreTranslated text
Key words
neuromorphic hardware,spike encoding network,configurable circuit,on-chip encoding
AI Read Science
Must-Reading Tree
Example
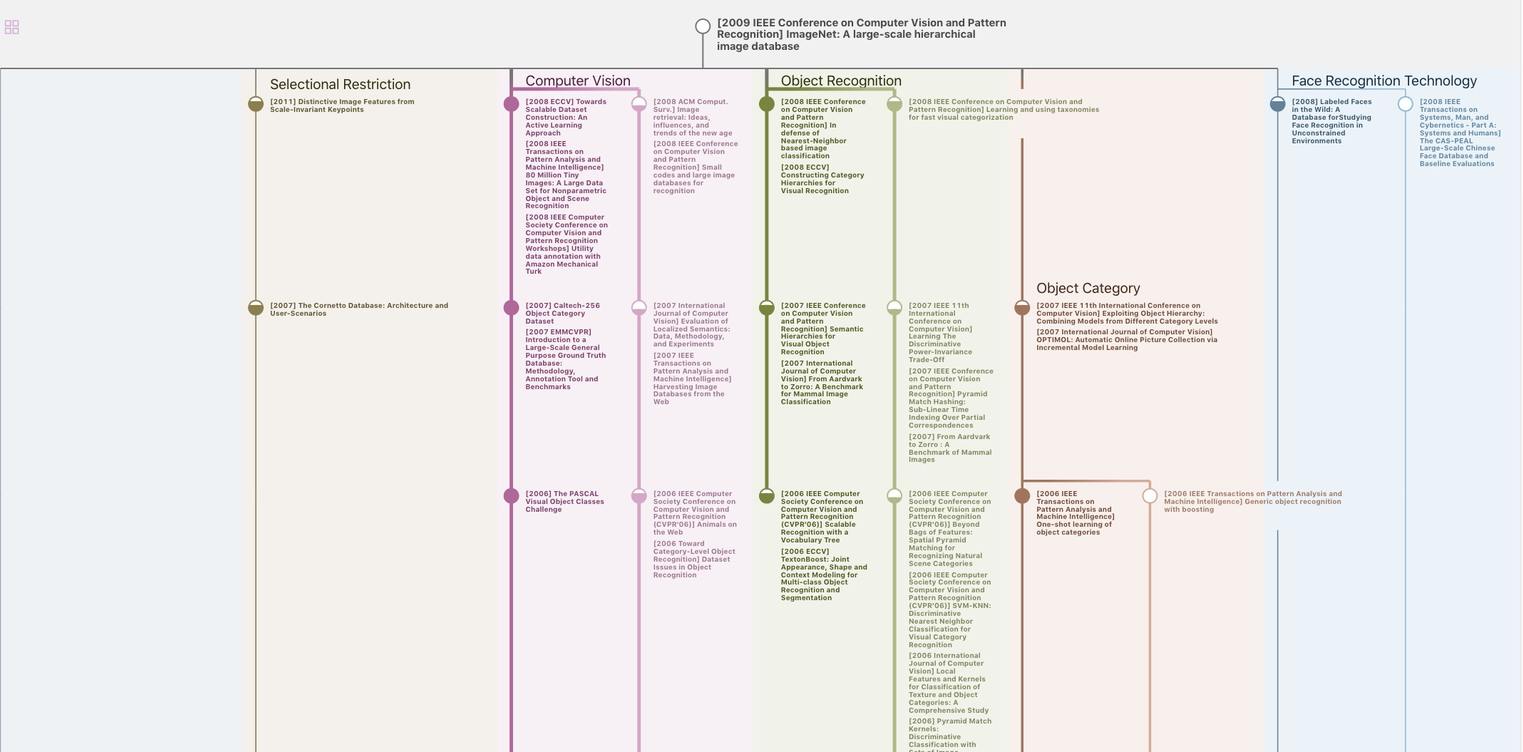
Generate MRT to find the research sequence of this paper
Chat Paper
Summary is being generated by the instructions you defined