A Comparative Study of Deep Learning based MODWT-LSTM and Machine Learning Model to Predict Environmental Parameters of Polyhouse
2022 IEEE International Conference on Current Development in Engineering and Technology (CCET)(2022)
摘要
The accurate prediction of environmental parameters inside the polyhouse is prerequisite for reducing the potential yield and financial losses which might occur due to variations in weather conditions. A precise predictive model to forecast the internal climate of the polyhouse several hours beforehand on the basis of present and past weather conditions is needed for an hour. In this study, a new hybrid approach has been proposed which coupled artificial intelligence based deep learning model (Long-Short Term Memory (LSTM)) with Maximum Overlap Discrete Wavelet Transform (MODWT) to forecast the polyhouse environment. The wavelet transform method improved 10 to 20% accuracy of deep leaning model (LSTM) by filtering input data and extracting significant information. A comparative performance analysis of three machine learning models (MLP, SVM, GPR) with deep learning model (Long Short Term Memory (LSTM)) have been evaluated for predicting the temperature and relative humidity of polyhouse. This study also explores the ability of newly proposed hybrid model MODWT-LSTM and compares accuracy with LSTM. The findings of this study shows that MODWT-LSTM performs better than other models for predicting temperature (R
2
=0.95, RMSE=0.64°C) and relative humidity (R
2
=0.97, RMSE=2.43%) of polyhouse. The outcome of proposed work also states that deep learning can be applied more precisely for monitoring and controlling the environment of polyhouse.
更多查看译文
关键词
Deep Learning,Climatic Parameters,Polyhouse,Forecasting,Machine Learning
AI 理解论文
溯源树
样例
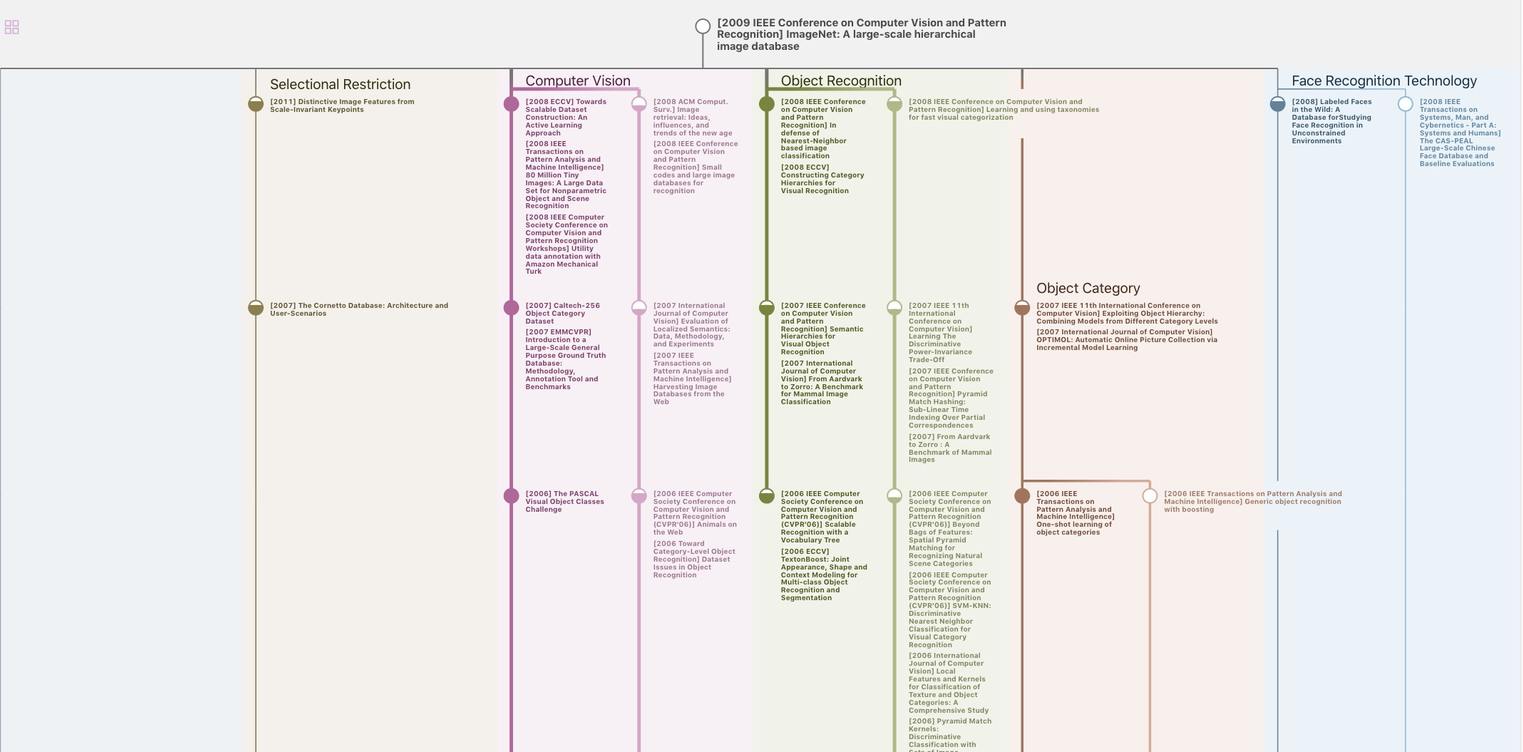
生成溯源树,研究论文发展脉络
Chat Paper
正在生成论文摘要