Extracting Leaf Wetness Duration using Baseline Correction through Group-Sparse Total Variation Method for LW Sensor
2023 IEEE Applied Sensing Conference (APSCON)(2023)
Abstract
Today’s sensor-driven technological era has enabled modernization in the agriculture sector. Plant disease detection technologies play a pivotal role in considering crop loss due to fungal diseases, weeds, or pests. To detect plant-fungal disease, it is essential to measure the leaf wetness duration (LWD) on the leaf, for which leaf wetness sensors (LWS) are widely used. In this work, an inhouse, capacitive electronic leaf wetness sensor has been used along with interface electronics and deployed close to the mango plant. Further, the data are collected for about one week and applied Group-Sparsity Total Variation (GSTV) pre-processing data algorithms to extract the LWD information from the self-collected data. We found that GSTV outperforms extracting LWD when compared with the other data pre-processing methods such as TV, SavitzkyGolay Filter, 1-dimension median filter, and Wavelet-based considering the accuracy. To benchmark the measured LWD from the fabricated LWS, we also deployed commercially available Phytos-31 LWS. Field measurement results indicate that developed LWS measures LWD with a maximum discrepancy of about \pm 30 minutes compared with commercially available LWS.
MoreTranslated text
Key words
Group-Sparsity Total Vanation (GS-TV),Leaf wetness duration (LWD),Leaf wetness sensor (LWS),Plant disease detection
AI Read Science
Must-Reading Tree
Example
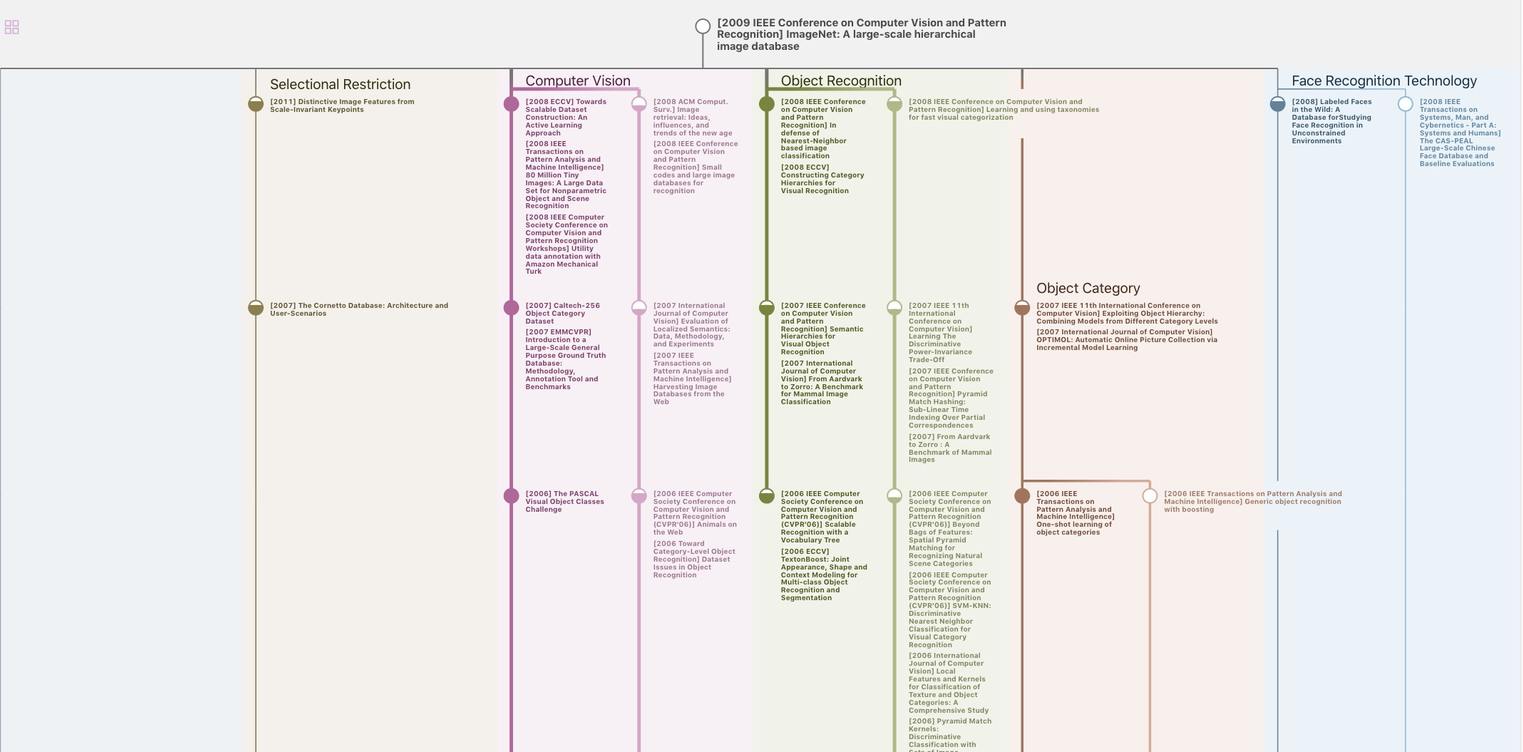
Generate MRT to find the research sequence of this paper
Chat Paper
Summary is being generated by the instructions you defined