Multi-sensor Fusion Framework for Obstacle Avoidance via Deep Reinforcement Learning
2022 2nd International Conference on Electrical Engineering and Control Science (IC2ECS)(2022)
摘要
Deep reinforcement learning enables the agent to decide autonomously for obstacle avoidance, and the policy is learned by interacting with the environment without labelled data. However, the difference between simulation and reality affects the transferring of the policy. The data of lidar shows consistence between sim and real, but possesses limited filed of vision. And the data of camera have wide vertical view and rich features, but differs greatly between simulated and actual worlds. To solve the problem of avoiding irregular and dynamic obstacles, we propose a multi-sensor fusion framework for obstacle avoidance via deep reinforcement learning. After preprocessing the data from different sensors, we segment the color image from RGB camera and process the depth image according to the segmented image. We remove the traversable area from the depth image, assign the depth region labelled as person a coefficient of inflation and down sample the depth image to pseudo-lidar. Then we calibrate the data from lidar with pseudolidar and get calibrated lidar for subsequent policy network. To overcome the problem of avoiding moving obstacles, we integrate the Long Short Term Memory network into the Proximal Policy optimization network, and design corresponding training process. We train the network in simulation, and test it in both simulation and reality. The results demonstrate the effectiveness of our method.
更多查看译文
关键词
multi-sensor fusion,obstacle avoidance,deep reinforcement learning,sim-to-real
AI 理解论文
溯源树
样例
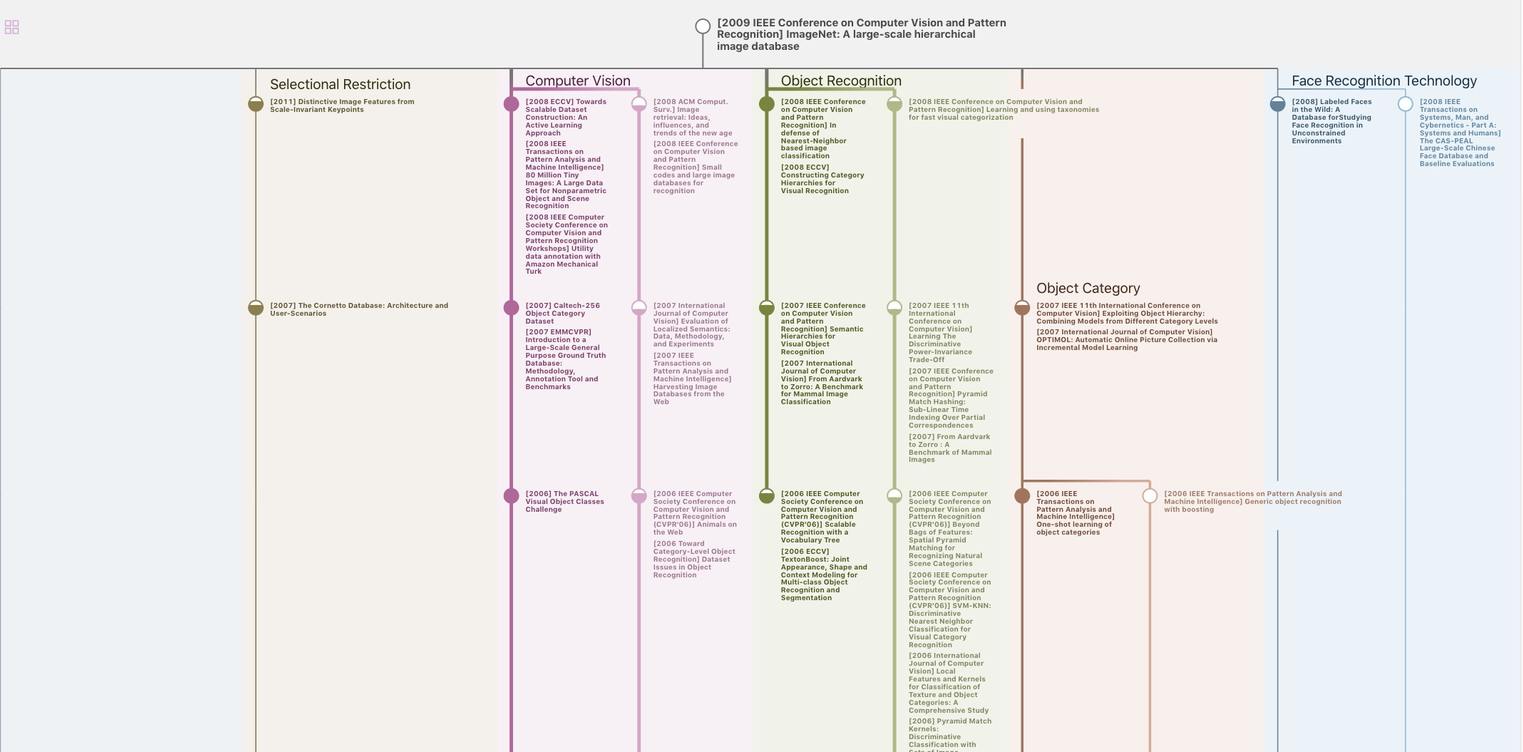
生成溯源树,研究论文发展脉络
Chat Paper
正在生成论文摘要