Scale-Guided Adaptive Sampling for General Object Detection Algorithms
2022 IEEE 8th International Conference on Computer and Communications (ICCC)(2022)
摘要
In object detection task based on deep learning, how to solve the imbalance of positive and negative samples has always been a difficult problem due to the enormous number of candidate anchor boxes. The traditional method uniformly processes the anchor boxes on feature maps of different scales to distinct positive and negative samples, ignoring the fact that different scale feature maps have different characteristics. For an object of a certain size, if anchors being assigned for regression are on the a too high-level feature map, it will lose a lot of detailed information; on the contrary, if the regressed anchors are assigned on a too low-level feature map, it is not enough to correctly detect due to the loss of semantic information. Based on this, this paper proposes a scale-guided adaptive sampling strategy for general object detection. The core idea is to assign positive samples on the feature map of the appropriate scale for each detected object. Specifically, two main parts of improvement are proposed. One is scale-oriented adaptive positive sample selection strategy(SASS). In this part, a global threshold used to classify positive samples is calculated according to the mean value of the anchors laid on the feature map of each scale, instead of using a fixed value directly. In addition, in order to avoid the problem of inaccurate object feature extraction caused by discarding feature maps, this paper also proposes a multi-scale feature fusion method(MFF) based on the attention mechanism, which further optimizes the scale-oriented automatic feature proposed in this paper. We verify the effectiveness of the proposed method on popular PASCAL VOC dataset, and our method achieves significant performance improvement compared to existing object detection algorithms.
更多查看译文
关键词
object detection,deep learning,multi-scale feature fusion,adaptive positive sample selection
AI 理解论文
溯源树
样例
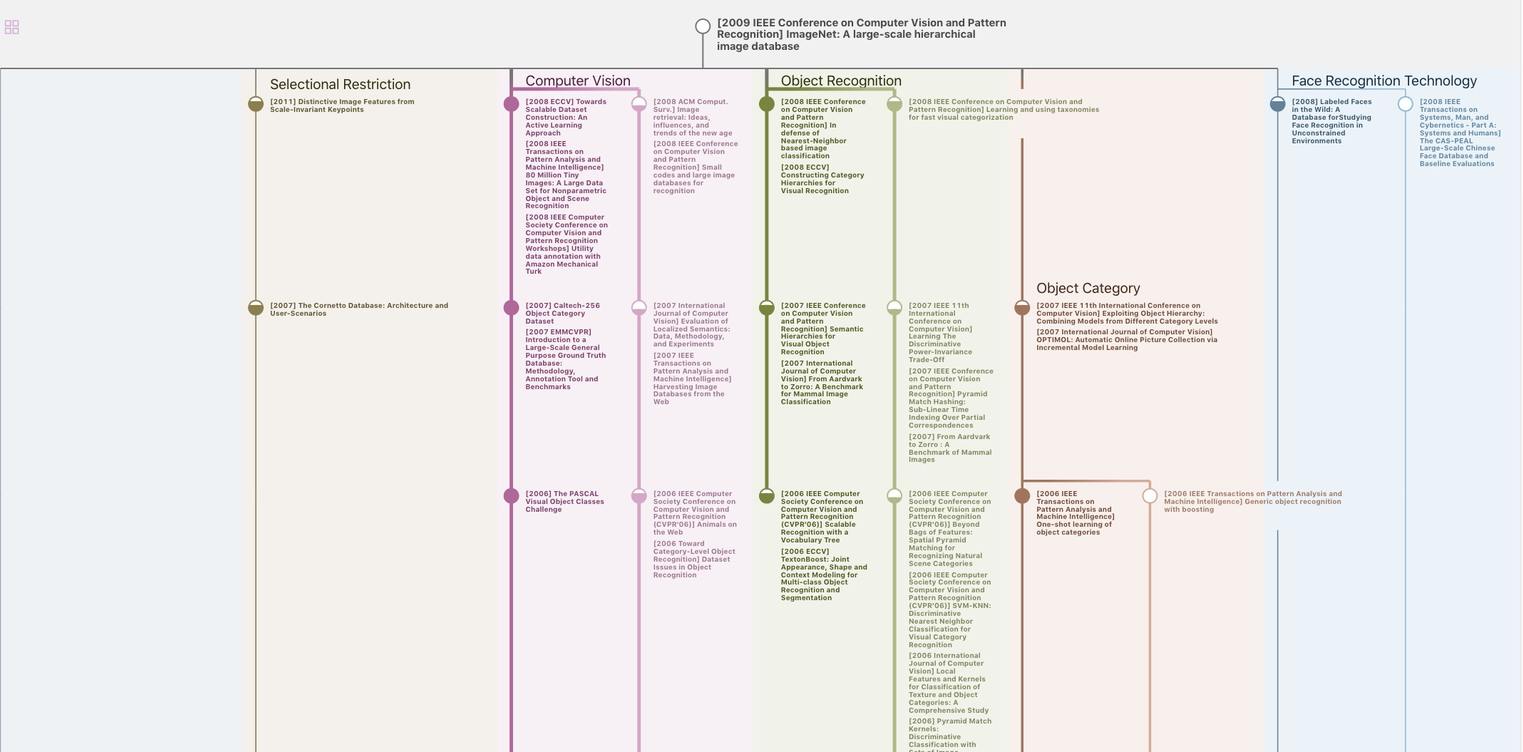
生成溯源树,研究论文发展脉络
Chat Paper
正在生成论文摘要