Data Augmentation of Insulator Aerial Images for Intelligent Inspection of Electric Transmission Systems
2022 IEEE 6th Conference on Energy Internet and Energy System Integration (EI2)(2022)
摘要
Insulators on the electric power transmission lines are prone to defects due to outdoor exposure that can degrade the system performance or even lead to power outage. The existing solutions of defect diagnosis based on vision-based supervised deep learning algorithms suffer from the lack of defect samples. This work develops an efficient data augmentation method for aerial insulator images to promote the detection model performance. The insulator and background are separated firstly and the perspective transformation or random flip is adopted for the foreground and random erasing is used for the background in the augmentation process, respectively. In addition, the noises and weather features are incorporated for the overall image to simulate different photography scenarios. The proposed data augmentation solution is assessed through experiments using the CNN-based training models. The numerical results confirm that the proposed solution enables performance improvements for a range of models in terms of accuracy, recall and F2 score. Besides, the Grad-CAM visualization method is also used and demonstrates the superiority of models trained based on the augmented dataset for insulator defect diagnosis tasks.
更多查看译文
关键词
Data Augmentation,Insulator,CNN,Defect Diagnosis
AI 理解论文
溯源树
样例
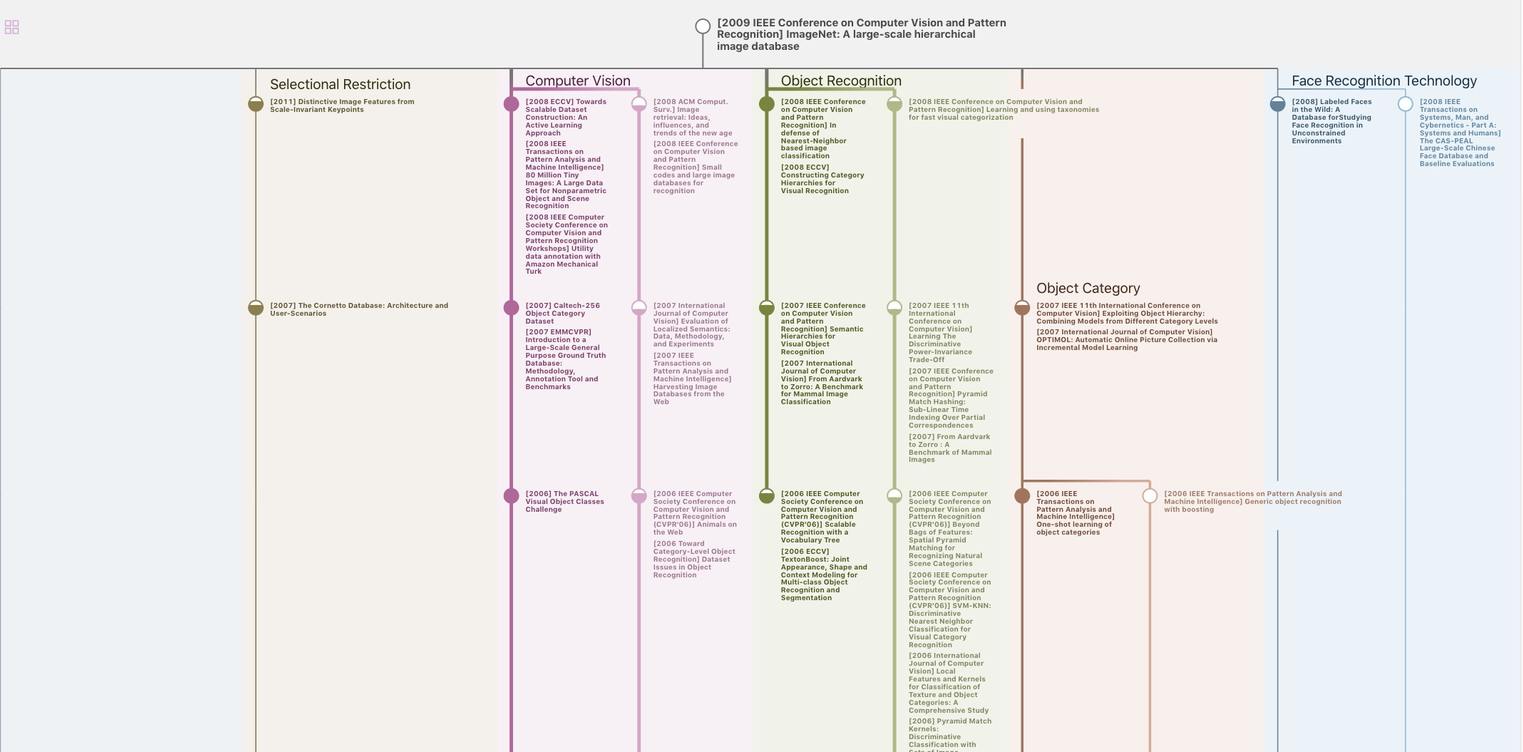
生成溯源树,研究论文发展脉络
Chat Paper
正在生成论文摘要