On Learning-based Model for Dynamic Granular Prediction of Power Outages under Extreme Events
2023 IEEE PES INNOVATIVE SMART GRID TECHNOLOGIES LATIN AMERICA, ISGT-LA(2023)
摘要
As the trend of climate change continues, an increase in the severity of extreme weather events is expected, posing a significant threat to the electric power infrastructure. The efficiency of service restoration efforts can be enhanced by having access to a highly granular outage forecasting tool with long lead times. In this study, we propose to develop and implement a multi-model framework as an operational tool that utilizes a dynamic, granular, multi-day outage forecasting model based on operational weather forecasts and detailed component outage information. To address the uneven distribution of different types of weather events and make better use of the timeseries data, a long-short-term-memory (LSTM)-based variational autoencoder (VAE) framework was developed to sample synthetic data and address data imbalance. With the balanced data, a prediction model was developed to estimate outages given a period of weather forecasts. The performance of the framework is demonstrated through several comparative studies.
更多查看译文
关键词
Variational autoencoder,outage prediction,recurrent neural networks,long-short-term-memory,weatherrelated outages
AI 理解论文
溯源树
样例
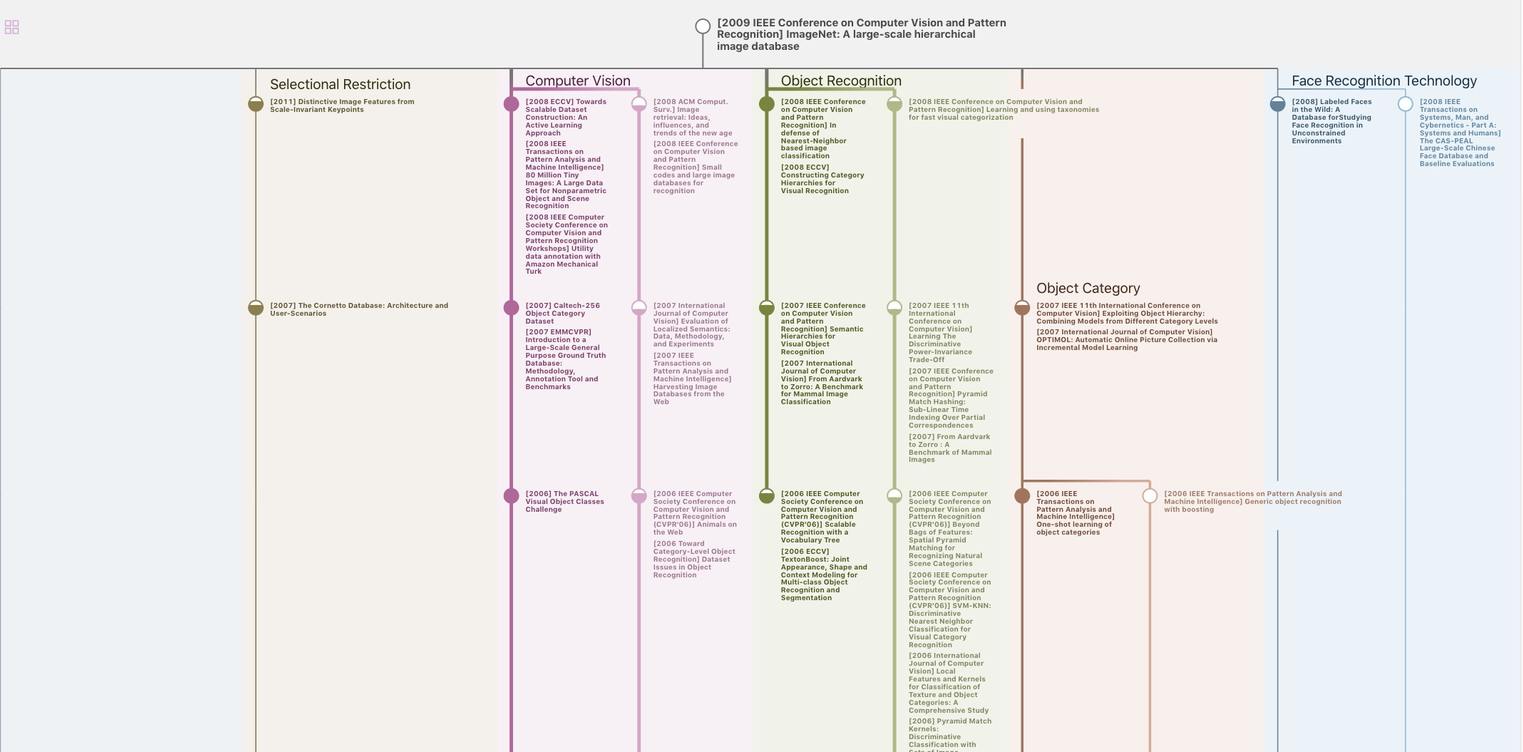
生成溯源树,研究论文发展脉络
Chat Paper
正在生成论文摘要