Answering Binary Causal Questions Using Role-Oriented Concept Embedding.
IEEE Transactions on Artificial Intelligence(2023)
Abstract
Answering binary causal questions is a challenging task, and it requires rich background knowledge to answer such questions. Extracting useful causal features from the background knowledge base and applying them effectively in a model is a crucial step to answering binary causal questions. The state-of-the-art approaches apply deep learning techniques to answer binary causal questions. In these approaches, candidate concepts are often embedded into vectors to model causal relationships among them. However, a concept may play the role of a cause in one question, but it could be an effect in another question. This aspect has not been extensively explored in existing approaches. Role-oriented causal concept embeddings are proposed in this article to model causality between concepts. We also propose leveraging semantic concept similarity to extract causal information from concepts. Finally, we develop a deep learning framework to answer binary causal questions. Our approach yields accuracy that is comparable to or better than the benchmark approaches.
MoreTranslated text
Key words
Causal focus (CF),causality,concept similarity,deep learning,role-oriented causal embedding
AI Read Science
Must-Reading Tree
Example
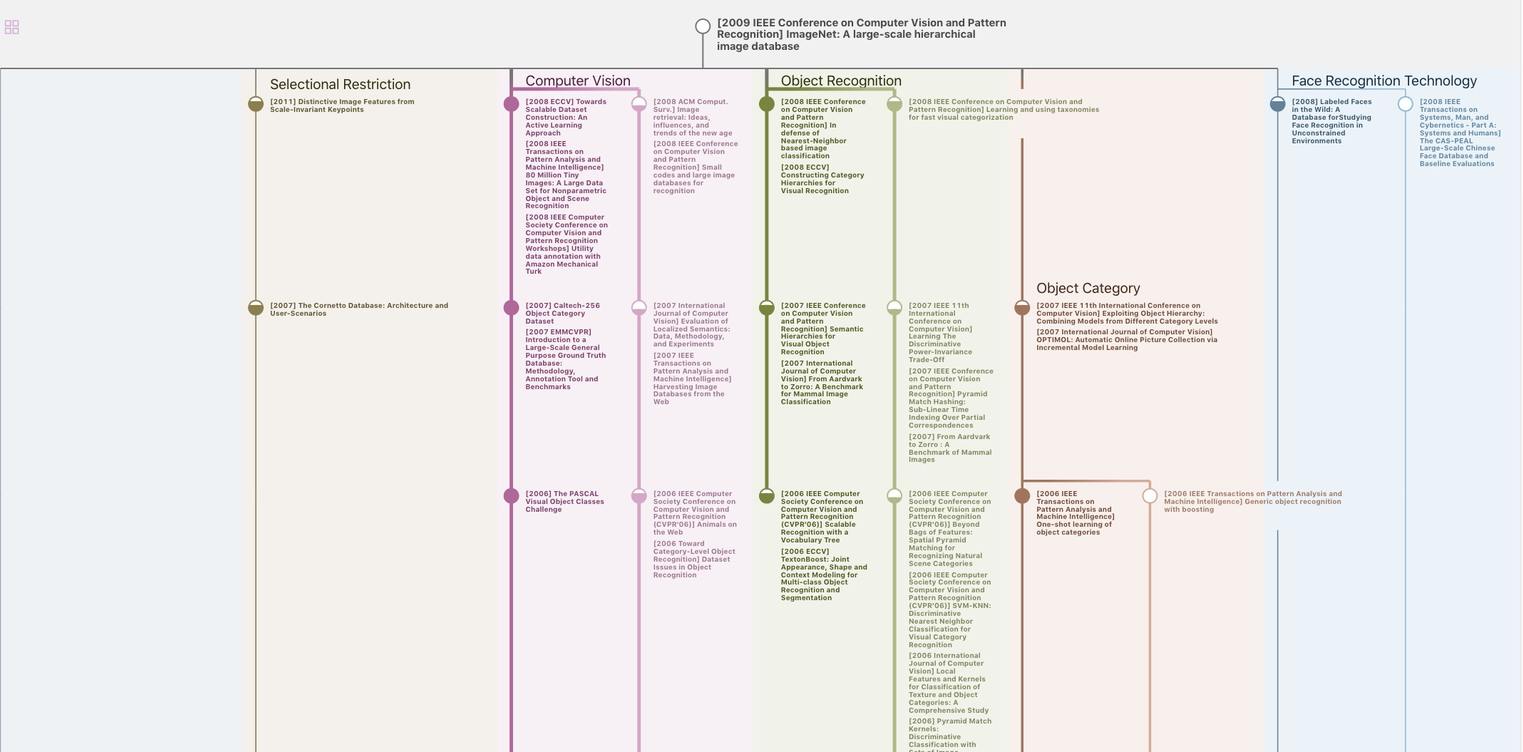
Generate MRT to find the research sequence of this paper
Chat Paper
Summary is being generated by the instructions you defined