Rotation-robust neighbor-based subcategory centroid alignment for corss-domain scene classification of areial images
IGARSS 2023 - 2023 IEEE INTERNATIONAL GEOSCIENCE AND REMOTE SENSING SYMPOSIUM(2023)
摘要
Semi-supervised domain adaptation methods can decrease the cost in producing training samples for scene classification. But they may deliver unsatisfactory performances because of the feature distribution bias between the previously labeled data and new aerial images caused by different imaging conditions. In order to address this problem, an original semi-supervised, rotation-robust domain adaptation (SRDA) framework is proposed to decrease the effect of feature distribution bias from the aspect of reducing the effect of rotation variance on representing scenes in aerial images and decreasing the influence of intra-class diversity on land-cover classification. The SRDA framework and some state-of-the-art domain adaptation methods are experimented on the UC Merced dataset to prove its superiority. The experimental results show the superiority of the proposed SRDA method over most of the previous domain adaptation approaches by at least 2% in overall accuracy.
更多查看译文
关键词
Semi-supervised domain adaptation,scene classification,feature distribution bias,rotation variance,intra-class diversity
AI 理解论文
溯源树
样例
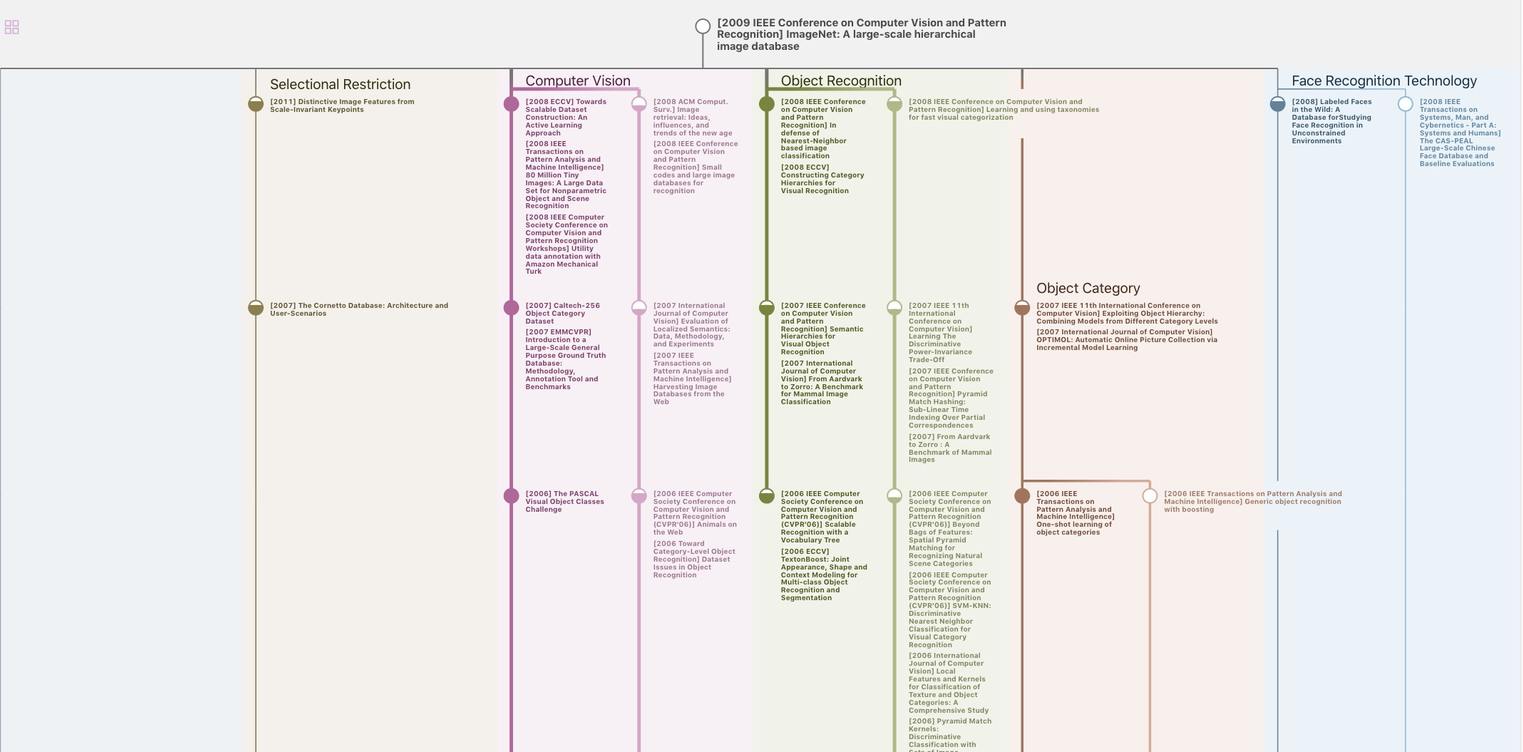
生成溯源树,研究论文发展脉络
Chat Paper
正在生成论文摘要