Prediction of Heart Disease Using Machine Learning
2022 International Conference on Recent Trends in Microelectronics, Automation, Computing and Communications Systems (ICMACC)(2022)
摘要
Heart disease is defined as any interruption in the usual activity of the heart. Heart disease was expected to kill millions of people every year, accounting for 32% of all deaths worldwide. To lower the death rate, it is important to detect heart diseases in their beginning phases so that the patient can start the treatment as soon as possible. For detecting heart disease, machine learning is an effective system to be applied. The primary goal of this paper is to attempt to predict heart disease, analyzing different attributes at an early stage to avoid disastrous consequences. Four machine learning models comprise the entire system: Logistic regression model, Decision Tree model, Random Forest model, and XGBoost Classifier model. In this system, there is a dataset of 1025 UCI data collected from Kaggle. It consists of 13 attributes in total. The dataset is evaluated and used in a variety of machine learning models. It has been divided into two segments for training and testing respectively. Then, the model has been trained using the training dataset. It is then evaluated on a test dataset. Different accuracies can be found for different models. Comparing the results, the Random Forest model and XGBoost model show 92.20% and 95.61% accuracy consecutively which are better than the Logistic Regression model and Decision Tree model holding 87.32% and 86.83% accuracy for predicting heart disease. As the Random Forest model shows good accuracy, a mobile application is developed based on this model. Thus, the study will benefit individuals by predicting whether a patient has heart problems or not.
更多查看译文
关键词
Machine learning,Heart Disease,Logistic regression model,Decision Tree model,Random Forest model,XGBosst Classifier model. Mobile application
AI 理解论文
溯源树
样例
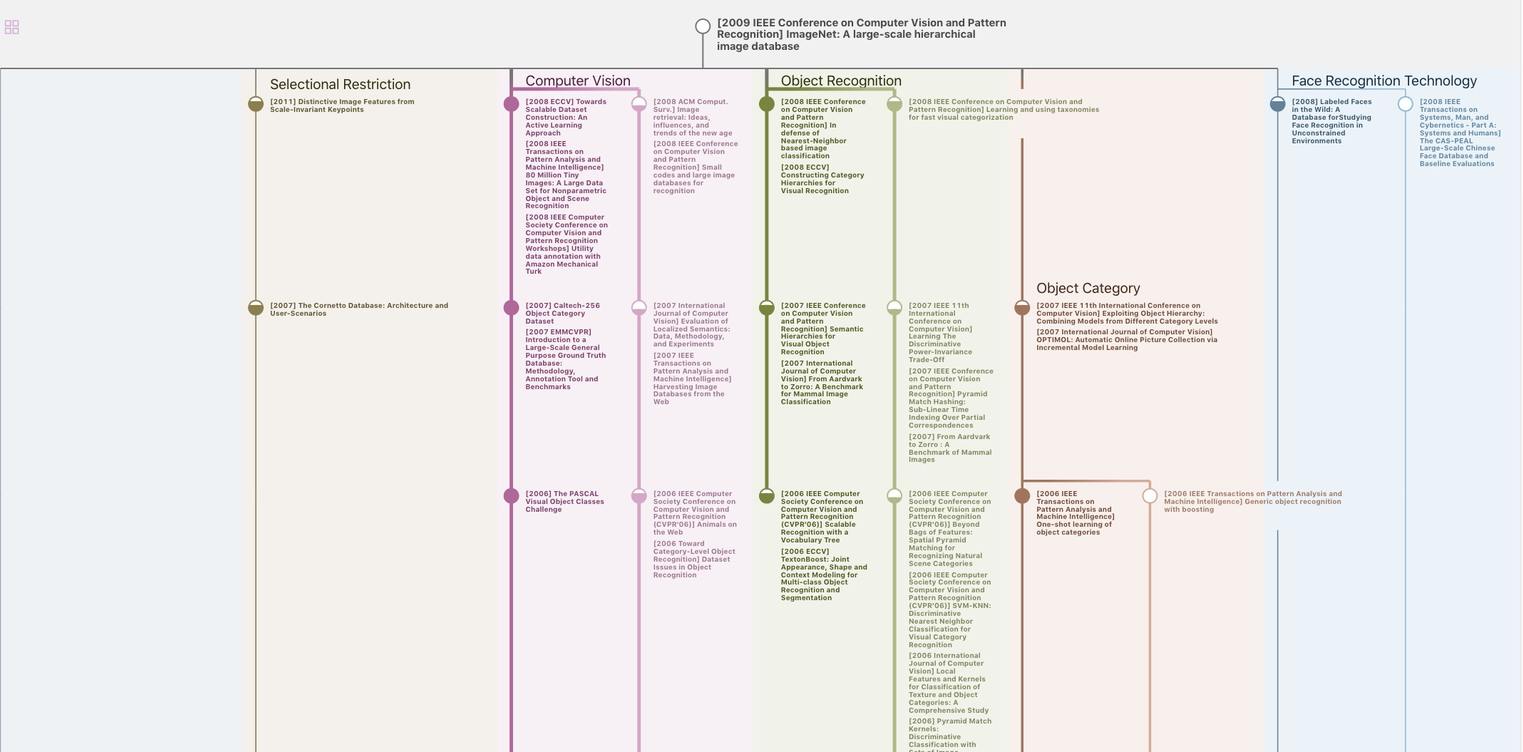
生成溯源树,研究论文发展脉络
Chat Paper
正在生成论文摘要