Machine Learning-based Dynamic Granular Electric Outage Forecasting
2023 Resilience Week (RWS)(2023)
摘要
As the trend in climate change continues, extreme weather events are expected to occur with increasing frequency and severity and pose a significant threat to the electric power infrastructure. Regardless of utilities' efforts in hardening the grid, damage to the utility assets such as overhead cables and distributed energy resources (DERs) that are particularly vulnerable to such events is unavoidable. Having a highly granular outage forecasting tool with a long lead time will be a great advantage for service restoration. In this study, we propose to develop and implement a multi-model framework as an operational tool based on a dynamic, granular, multi-day electric outage forecasting model using numerical weather forecasts and detailed component failure information. An innovative two-layered dynamic neural network and a sliding window are used to make better use of the available data. Case studies are performed to demonstrate the performance of the proposed framework.
更多查看译文
关键词
North American Mesoscale Forecast System (NAM),Global Forecast System (GFS),Grid outage forecasting,Numerical weather prediction,Long-short-term-memory,data standardization
AI 理解论文
溯源树
样例
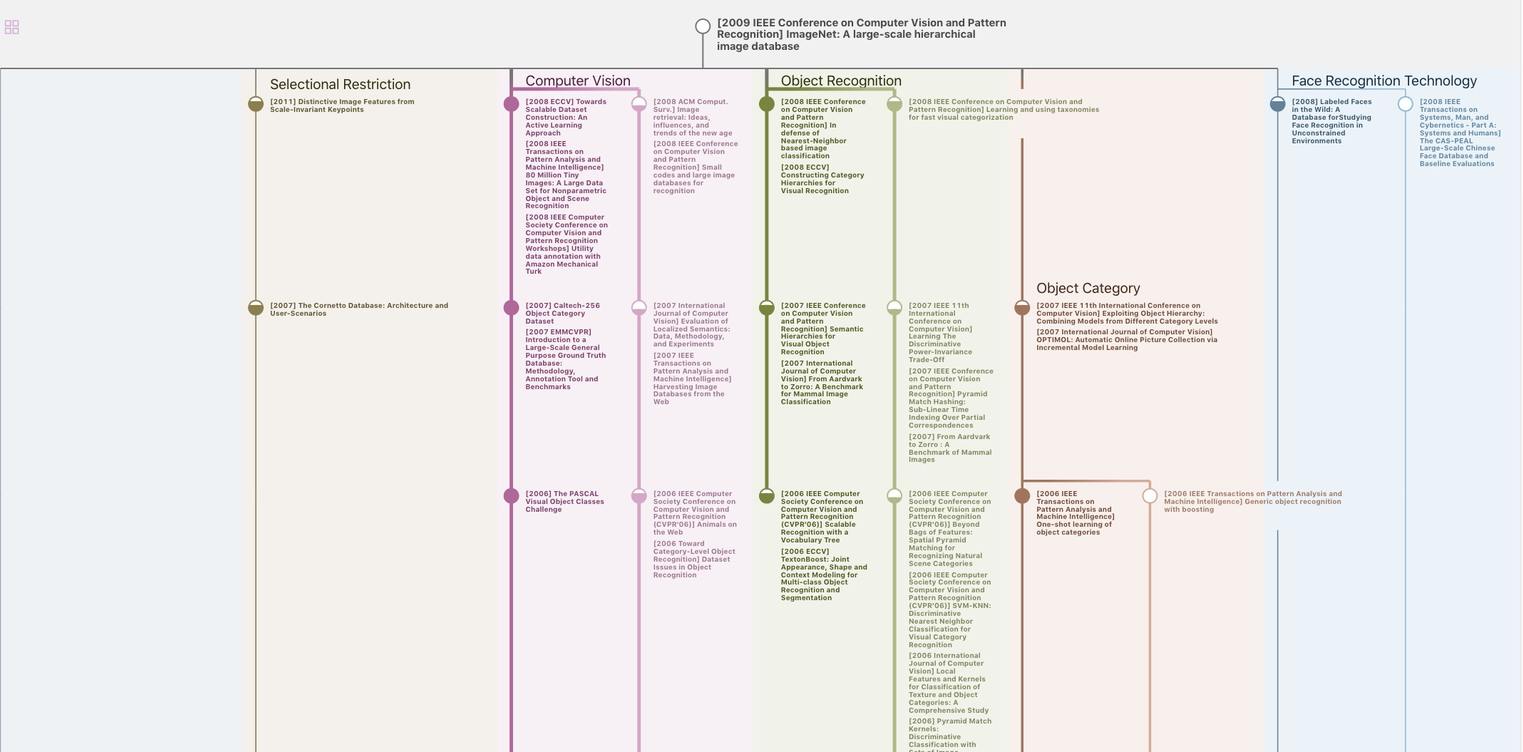
生成溯源树,研究论文发展脉络
Chat Paper
正在生成论文摘要