Cnn-mixer hierarchical spectral transformer for hyperspectral image classification
IGARSS 2023 - 2023 IEEE INTERNATIONAL GEOSCIENCE AND REMOTE SENSING SYMPOSIUM(2023)
摘要
Hyperspectral image (HSI) classification using vision Transformers is an area of active research in the field of remote sensing. However, vision Transformers suffer from lack of inductive bias inherent in convolutional neural networks (CNNs), which hinders their generalization ability when working with limited amounts of data. Furthermore, some research indicates that the competitive performance of vision Transformers primarily stems from the overall architecture, characterized by alternating residual layers of sequence mixers and multi-layer perceptron (MLP) blocks, rather than solely relying on the multi-head self-attention (MSA) mixer module. To this end, a novel CNN-mixer hierarchical spectral Transformer applied to hyperspectral image classification is proposed. To extract spectral features, a hierarchical spectral Transformer is designed to aggregate discriminative features from the spectral dimension. To remedy the lack of inductive bias inherent in vision Transformers, a simple CNN mixer is substituted for the MSA mixer to capture the local spatial features of each HSI window patch. Accordingly, the proposed network architecture leverages advantages of both CNNs and vision Transformers. Through extensive experiments, we find that the proposed method outperforms the state-of-the-art (SOTA) methods applied to the University of Houston, Botswana, and Salinas HSI datasets.
更多查看译文
关键词
hyperspectral image (HSI) classification,convolutional neural networks (CNNs),vision Transformer
AI 理解论文
溯源树
样例
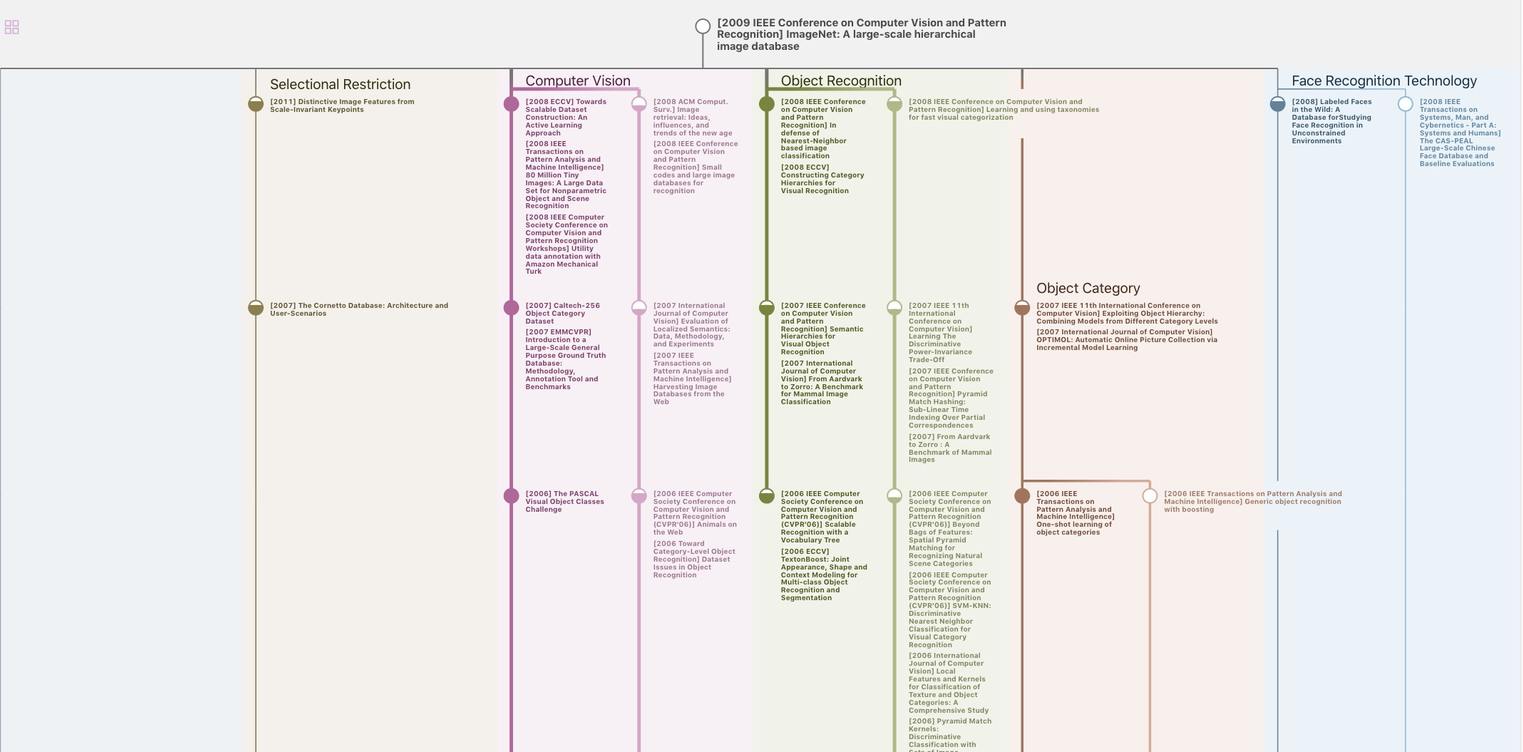
生成溯源树,研究论文发展脉络
Chat Paper
正在生成论文摘要