Fast machine learning simulator of at-sensor radiances for solar-induced fluorescence retrieval with desis and hyplant
IGARSS 2023 - 2023 IEEE INTERNATIONAL GEOSCIENCE AND REMOTE SENSING SYMPOSIUM(2023)
摘要
In many remote sensing applications the measured radiance needs to be corrected for atmospheric effects to study surface properties such as reflectance, temperature or emission features. The correction often applies radiative transfer to simulate atmospheric propagation, a time-consuming step usually done offline. In principle, an efficient machine learning (ML) model can accelerate the simulation step. This is the goal pursued here in the context of solar-induced fluorescence (SIF) emitted by vegetation around the O-2-A band using the spaceborne DESIS and airborne HyPlant spectrometers. We present an ML simulator of at-sensor radiances trained on synthetic spectra and describe its performance in detail. The simulator is fast and accurate, constituting a promising alternative to a full-fledged, lengthy radiative transfer code for SIF retrieval in the O-2-A band with DESIS and HyPlant.
更多查看译文
关键词
solar-induced fluorescence,hyperspectral sensors,radiative transfer,machine learning
AI 理解论文
溯源树
样例
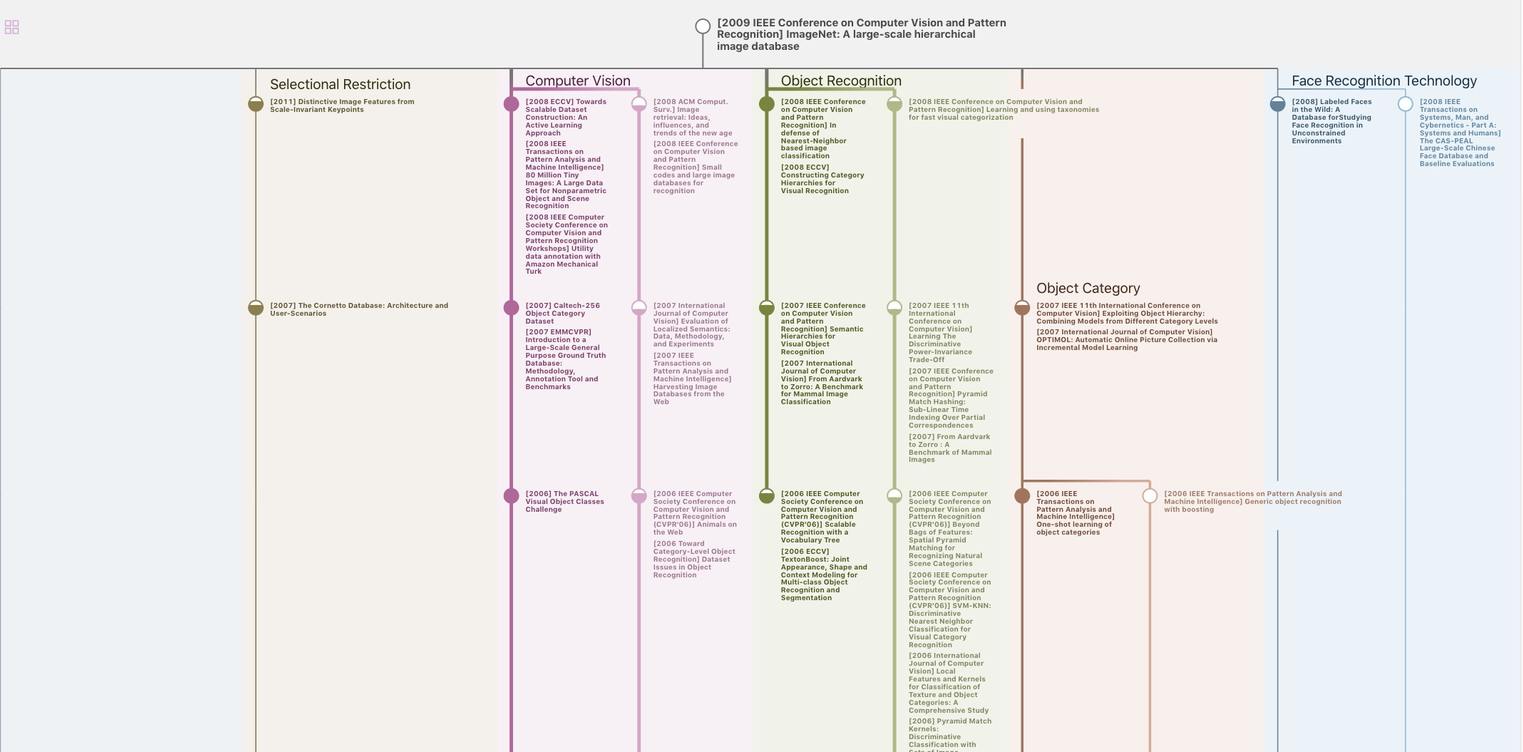
生成溯源树,研究论文发展脉络
Chat Paper
正在生成论文摘要