A Perceptual Metric Prior on Deep Latent Space Improves Out-Of-Distribution Synthetic Aperture Sonar Image Classification
IGARSS 2023 - 2023 IEEE INTERNATIONAL GEOSCIENCE AND REMOTE SENSING SYMPOSIUM(2023)
摘要
Deep learning methods have achieved state-of-the-art performance on various machine learning benchmarks. However, when evaluated on data outside of the training-set distribution, performance can be mixed, even though this data is trivial for humans to discern. One reason for this gap is that deep network training is only tasked with solving a context-limited optimization problem. As long as the loss on the training data is minimized, any suitable set of features and decision boundary geometry are candidate solutions. This can result in the reliance on non-robust features that do not take into account the larger context, leading to poor performance on out-of-distribution (OOD) data that presents no trouble for humans. For example, training on a limited set of 2-dimensional image data makes it difficult to learn relationships that may be obvious in 3 dimensions. To partially mitigate this issue, we propose using a perceptual metric prior (PMP) to influence the latent manifold structure, mimicking the characteristics of human perception and improving OOD performance. Our method is demonstrated on a real-world synthetic aperture sonar (SAS) dataset, showing good performance on OOD imagery, even when only limited training data is available, as is often the case in SAS. Our proposal aims to address the under-specification issue by taking into account how inter-class samples relate to each other, encouraging the latent feature manifold to consider a larger context.
更多查看译文
AI 理解论文
溯源树
样例
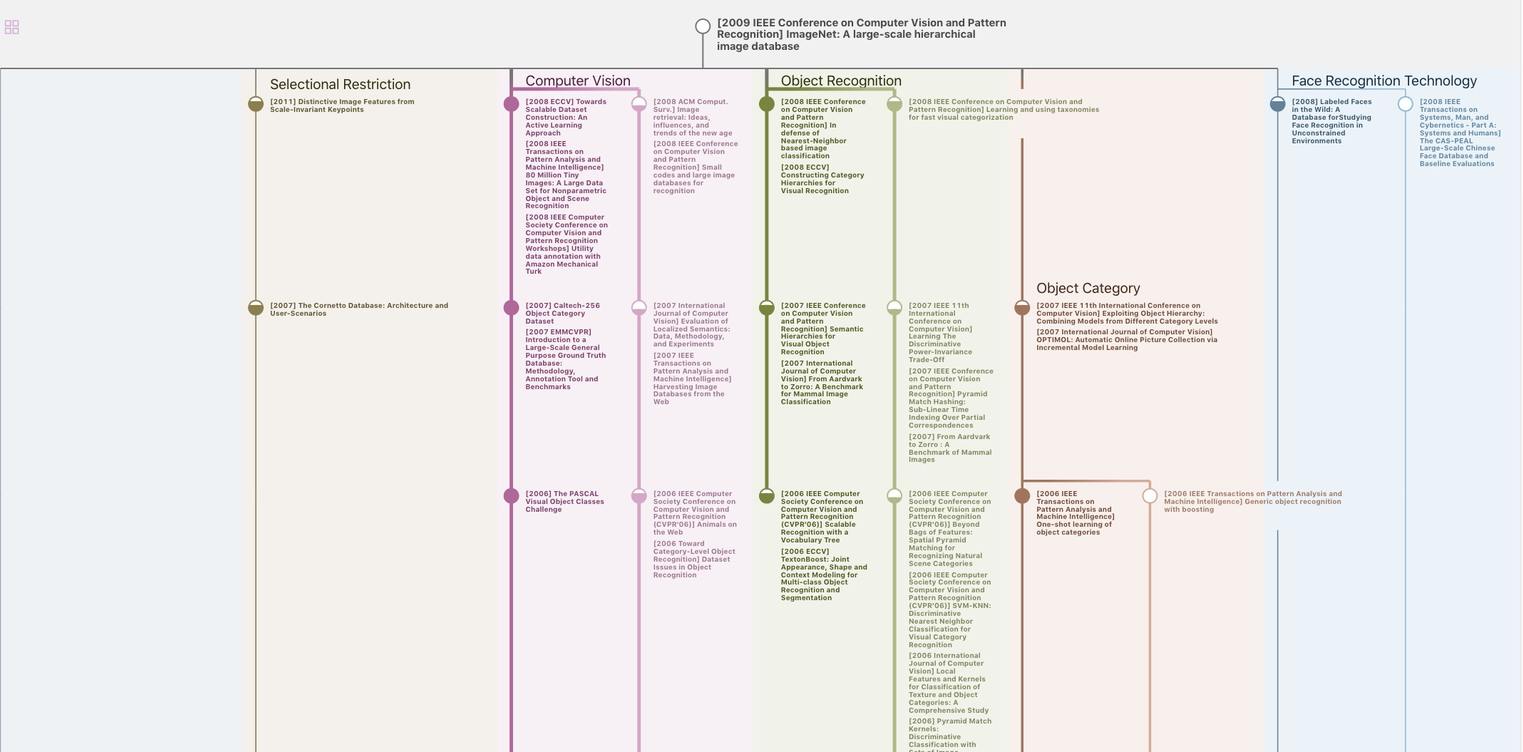
生成溯源树,研究论文发展脉络
Chat Paper
正在生成论文摘要