A module for enhancing accuracy of building damage detection by fusing features from pre and post disaster remote sensing images
IGARSS 2023 - 2023 IEEE INTERNATIONAL GEOSCIENCE AND REMOTE SENSING SYMPOSIUM(2023)
Abstract
In the aftermath of large-scale natural disasters, the accuracy of building damage detection (BDD) is of critical importance. Post-disaster high-resolution (post-HR) remote sensing imagery is fundamental for BDD; however, prompt acquisition of such imagery remains a significant challenge. To address this issue, we introduce a novel plug-and-play feature fusion (FF) module. This module, strategically situated between a pre-trained super-resolution (SR) model and a BDD model, ingeniously combines features from both pre-disaster high-resolution (pre-HR) and super-resolved post-disaster remote sensing imagery. The proposed approach is designed to maximize the utilization of pre-HR images, thereby enhancing BDD accuracy. Experimental validation confirms that this improvement in accuracy is attributable to the pragmatic extraction of features from pre-HR imagery, not just an increase in model complexity. Consequently, our approach holds substantial promise for real-world post-disaster scenarios and lays a solid foundation for future BDD research, demonstrating potential improvements in both efficacy and practicality.
MoreTranslated text
Key words
Super-resolution,building damage,xBD dataset,feature extraction,deep learning
AI Read Science
Must-Reading Tree
Example
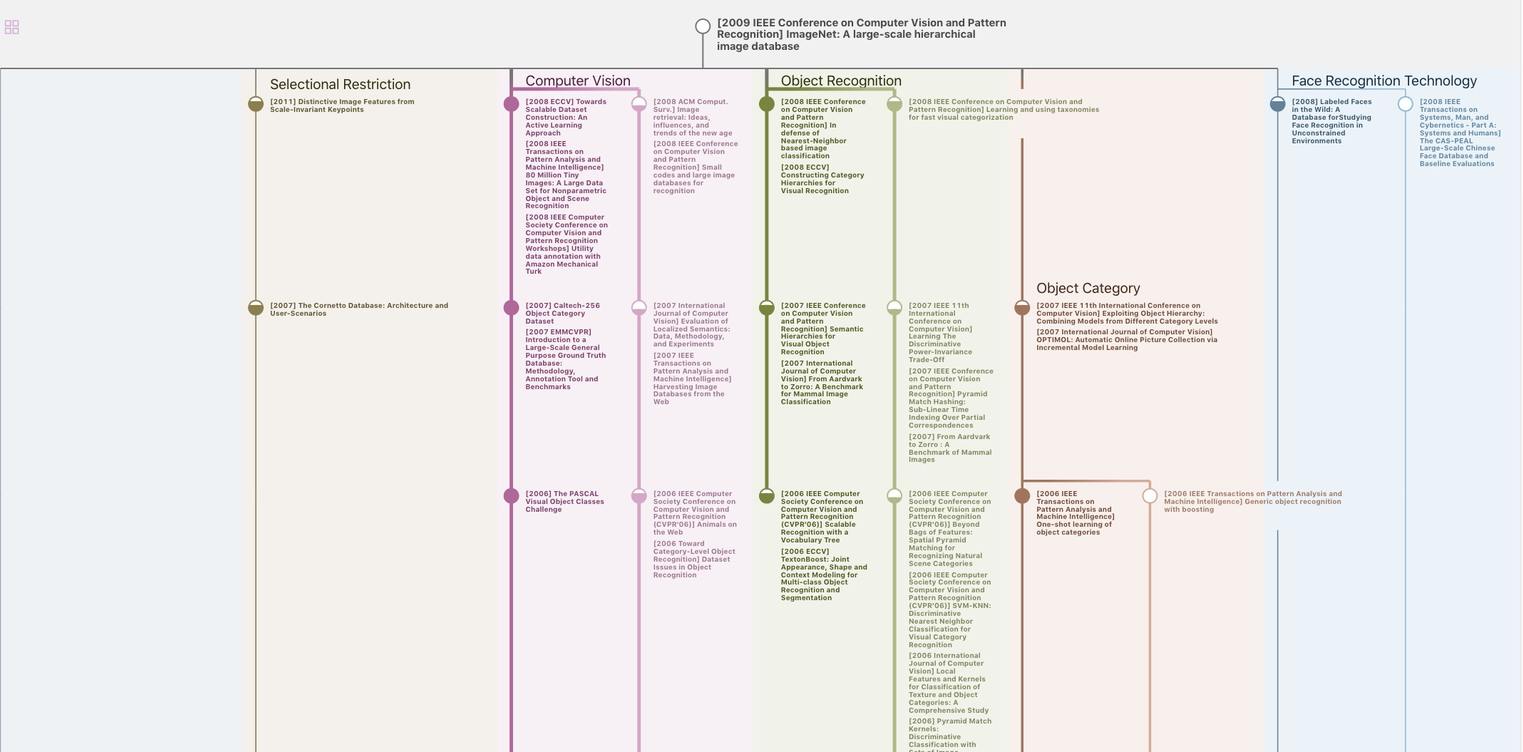
Generate MRT to find the research sequence of this paper
Chat Paper
Summary is being generated by the instructions you defined