Unsupervised geospatial domain adaptation for large-scale wildfire burned area mapping using sentinel-2 msi and sentinel-1 sar data
IGARSS 2023 - 2023 IEEE INTERNATIONAL GEOSCIENCE AND REMOTE SENSING SYMPOSIUM(2023)
摘要
Satellite remote sensing provides a cost-effective way for monitoring wildfires on a large scale, and the continuous observations and measurements have made remote sensing a primary source of unlabelled big data. Supervised deep learning has shown great success in various remote sensing applications, but it heavily relies on high-quality labels. However, burned area labels are only available for a small part of the world, supervised deep learning from limited labelled data has poor generalization performance across geographical regions and climate zones. Different satellite sensors represent the same physical objects in various ways, while multi-source satellite data often exhibits a combination of common and complementary information, such as optical and radar data. The common information makes it possible to exploit huge amounts of unlabelled multi-source data in model training through consistency regularization between multi-source predictions. In this work, we adopted an unsupervised geospatial domain adaptation (GDA) framework based Dual Stream U-Net model, which combines the supervised loss and unsupervised multi-modal consistency regularization to exploit both labelled and unlabelled multi-model data for model training in a semi-supervised learning manner. The experimental results demonstrate that unsupervised GDA has better generalization performance across geographical regions than fully supervised learning.
更多查看译文
关键词
Wildfire,burned area,domain adaptation,Sentinel-2,Sentinel-1,change detection,segmentation
AI 理解论文
溯源树
样例
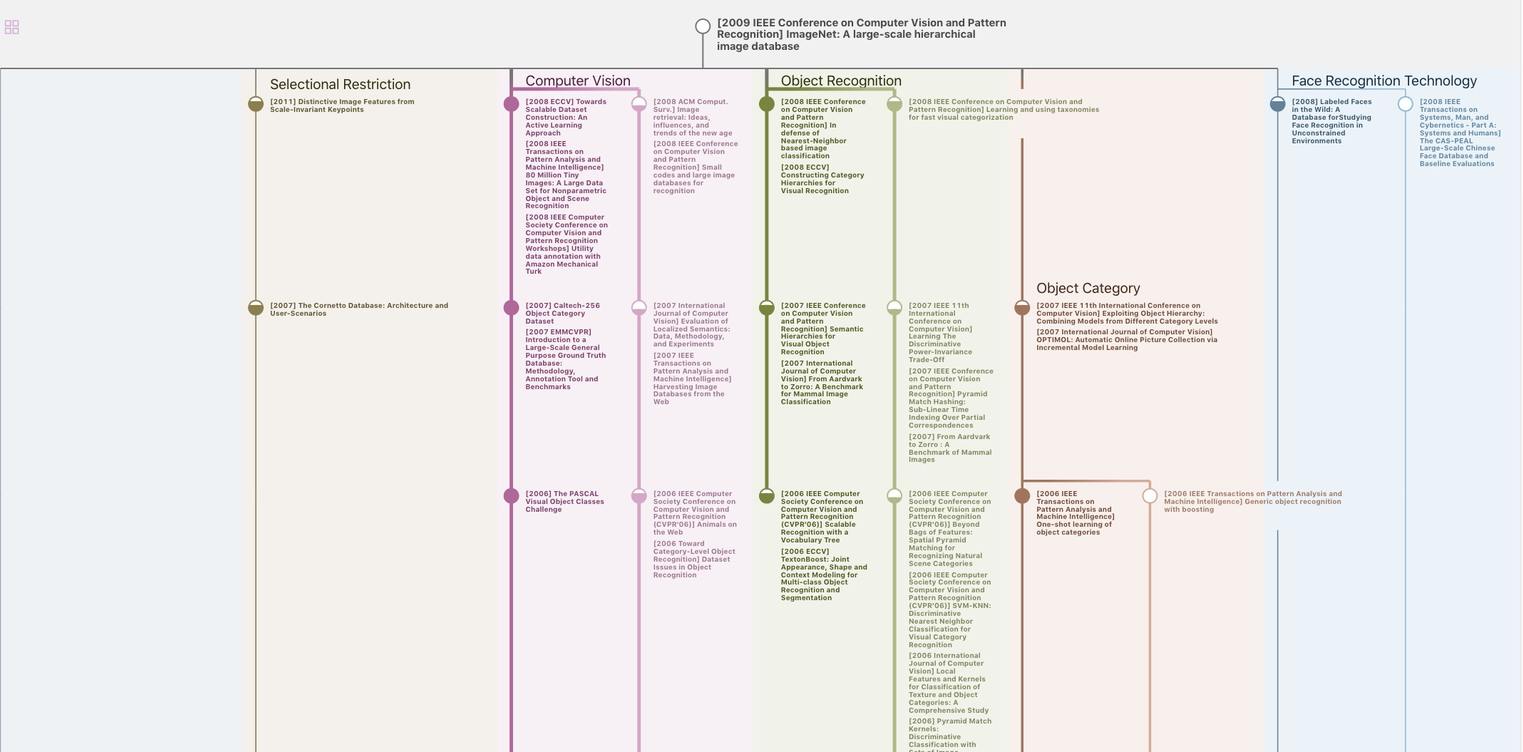
生成溯源树,研究论文发展脉络
Chat Paper
正在生成论文摘要