Water physics aware semantic segmentation through texture-biased u-net architectures
IGARSS 2023 - 2023 IEEE INTERNATIONAL GEOSCIENCE AND REMOTE SENSING SYMPOSIUM(2023)
摘要
Reliably identifying water bodies is an important step in automating the identification of potable water. This work therefore investigates water scene segmentation, with a focus on water's physical properties which give it features that distinguish it from other elements. We propose a physics-aware water segmentation method, in which we adapt both a U-Net and MACUNet model so that they are biased towards texture information by using a Gabor convolutional layer as the first layer, combined with a mixture of average and maximum pooling layers in the encoder. To train the networks, a dataset of annotated water images was created, comprising water bodies from diverse light, atmospheric, geographic, and environmental conditions. We show that the physics-aware, texture-biased models result in effective water segmentation. We then test the texture-biased models using 3 standard aerial scene segmentation benchmarks and show that in all cases they outperform the standard U-Net or MACUNet models. We suggest this is because the new models are sensitive to small variations in texture, meaning they can extract information from scenes affected by light, canopy or shadows.
更多查看译文
关键词
Semantic Segmentation,U-Net,Gabor,Aerial Images,Remote Sensing,Water
AI 理解论文
溯源树
样例
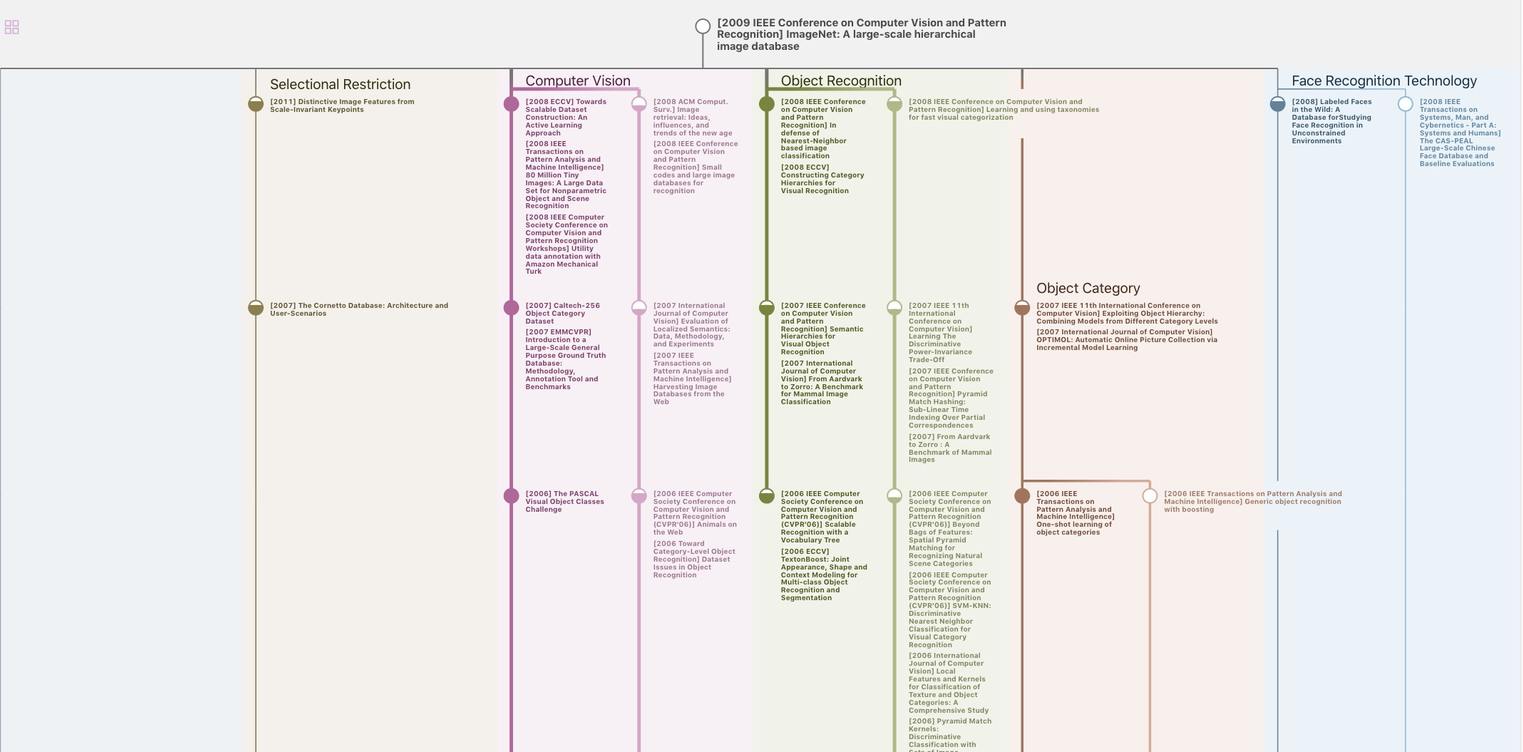
生成溯源树,研究论文发展脉络
Chat Paper
正在生成论文摘要