High-resolution fine-grained wetland mapping based on class-balanced deep semantic segmentation networks
IGARSS 2023 - 2023 IEEE INTERNATIONAL GEOSCIENCE AND REMOTE SENSING SYMPOSIUM(2023)
摘要
Wetlands are among the most valuable environmental resources and are crucial to achieving sustainable development strategies. However, the number, distribution, and types of wetlands are poorly understood. Widely used medium-resolution wetland products do not provide enough surface feature for fine-grained wetland mapping. To fill this gap, a high-resolution wetland remote sensing mapping dataset covering the contiguous United States was constructed. On this dataset, the performance of deep semantic segmentation networks with various backbones and architecture for wetland mapping was evaluated. Several loss functions were implemented to address the issue of class imbalance of this dataset, resulting in an improvement in classification accuracy. High spatial resolution images offer an abundance of surface texture, shape, structure, and neighborhood relationship data that can be applied to the classification of large-scale wetlands. The combination of high-resolution imagery and deep semantic segmentation models enables the automatic classification of wetlands to be refined.
更多查看译文
关键词
Wetland mapping,high-resolution remote sensing,deep learning,semantic segmentation
AI 理解论文
溯源树
样例
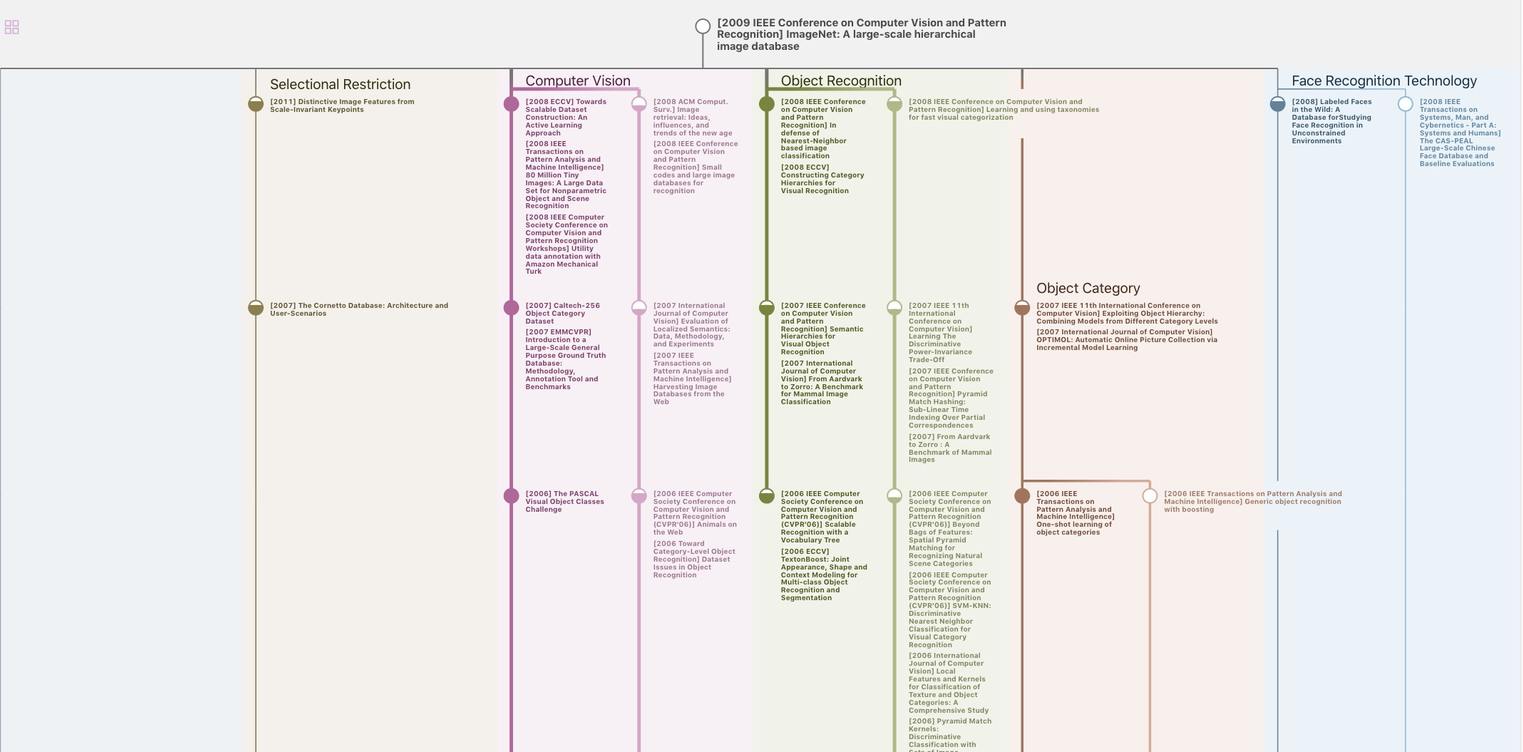
生成溯源树,研究论文发展脉络
Chat Paper
正在生成论文摘要