Satellite image augmentation via seamless instance blending for building damage segmentation
IGARSS 2023 - 2023 IEEE INTERNATIONAL GEOSCIENCE AND REMOTE SENSING SYMPOSIUM(2023)
摘要
When a natural disaster strikes, humanitarian organizations require a rapid and precise localization of damaged buildings to coordinate rescue missions and allocate relief goods. Current approaches rely on a manual comparison of pre- and post-disaster satellite imagery to generate a reliable damage map for selected areas. The main obstacle to using machine learning methods to automate this time-consuming analysis is the small amount of labeled training data. To generate more diverse datasets, we propose an augmentation procedure to seamlessly insert buildings into optical satellite imagery. We analyzed how different compositions of inserted buildings affect the segmentation of building damage and showed how the proposed augmentation technique can be used to significantly improve the performance of building damage segmentation networks.
更多查看译文
关键词
Seamless Augmentation,Building damage segmentation,Satellite Imagery,Deep Learning
AI 理解论文
溯源树
样例
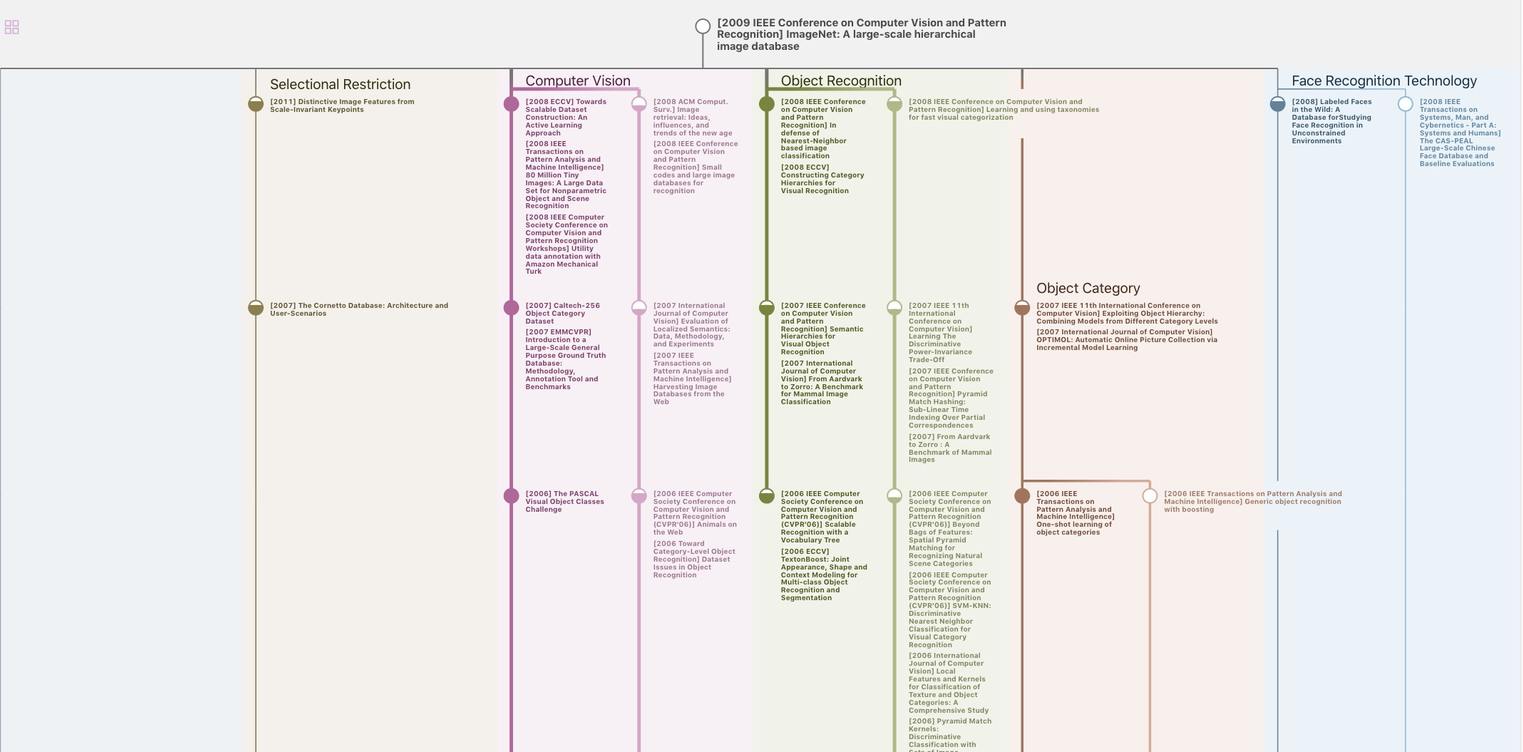
生成溯源树,研究论文发展脉络
Chat Paper
正在生成论文摘要