Hyperspectral data scarcity problem from a super resolution perspective: data augmentation analysis and scheme
IGARSS 2023 - 2023 IEEE INTERNATIONAL GEOSCIENCE AND REMOTE SENSING SYMPOSIUM(2023)
摘要
Hyperspectral Single Image Super Resolution is an important field of research due to the low spatial resolution of Hyperspectral Images (HSI) that limits their usability. Deep Convolutional Neural Networks (DCNNs) have been commonly used for SISR tasks, however, a large dataset is typically needed for training. Because of HSI data scarcity, training DCNNs for HSI-SISR becomes a challenging task. In this study, HSI data scarcity problem is tackled from an SISR perspective via Data Augmentation (DA). Several DA techniques are reviewed in this context, and a new DA technique called CutMixBlur is introduced. The best techniques are decided based on maximizing Peak Signal-to-Noise Ratio (PSNR) and Structure Similarity Index Measurement (SSIM) while minimizing Spectral Angle Mapper (SAM). The aim is to enhance HSI spatially without distorting their unique spectral signature. Experiments on Pavia University and Indian Pines datasets show that CutMixBlur boosts all quality metrics. Additionally, applying DA techniques by randomly stacking their effect significantly enhances the performance of SISR DCNNs, particularly the 3D-SRCNN.
更多查看译文
关键词
Single Image Super Resolution,Augmentation,Hyperspectral,3D-CNN,SRCNN
AI 理解论文
溯源树
样例
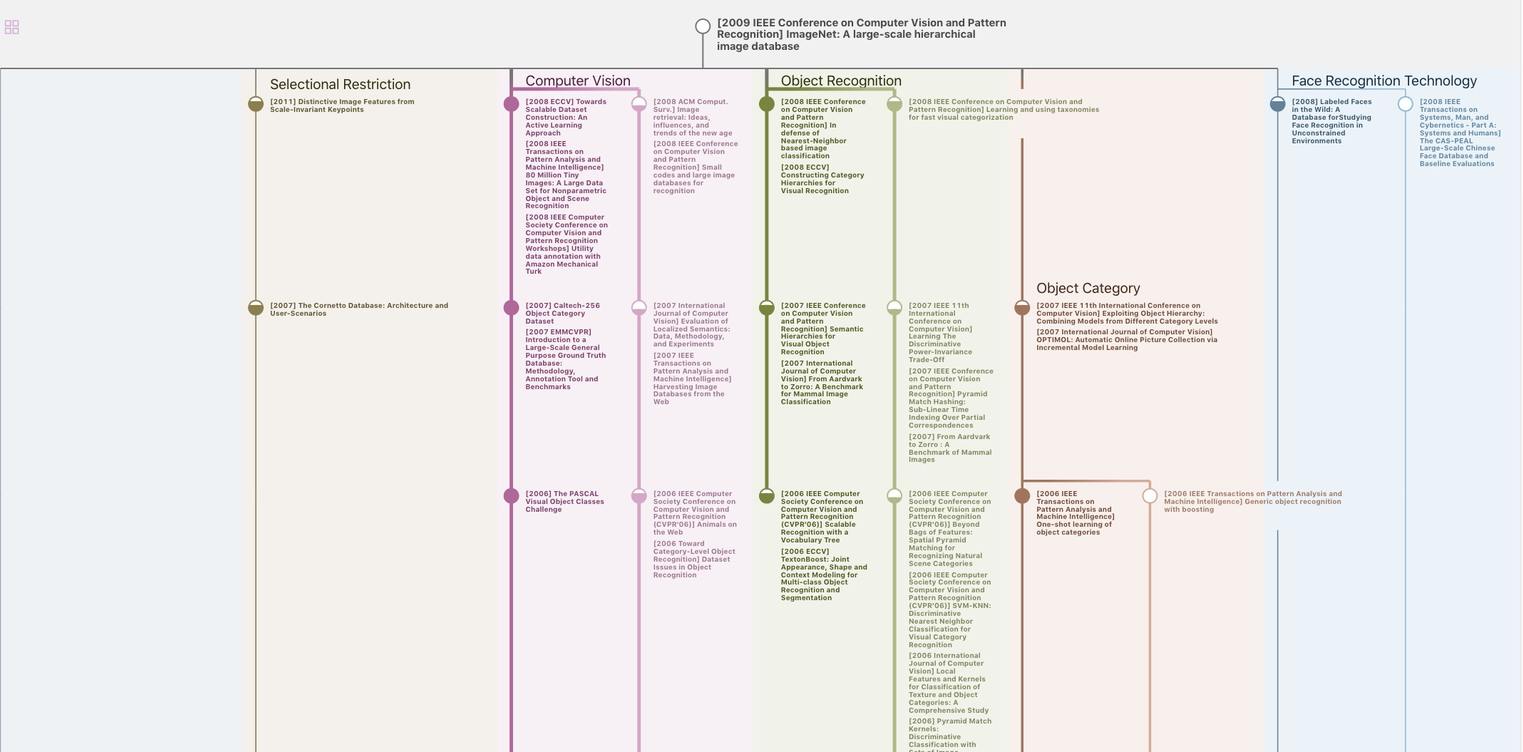
生成溯源树,研究论文发展脉络
Chat Paper
正在生成论文摘要