The prediction of regional wildfire risk using high-resolution remotely sensed soil moisture content estimation, case study: sidi douma forest, saida, algeria
IGARSS 2023 - 2023 IEEE INTERNATIONAL GEOSCIENCE AND REMOTE SENSING SYMPOSIUM(2023)
Abstract
Wildfires are increasing in frequency due to anthropogenic climate change. Live Fuel Moisture Content (LFMC) is a key parameter for wildfire behavior prediction. However, it is very difficult to measure via Earth observation data. This study suggests the use of Soil Moisture Content (SMC) as a substitute for LFMC for such a purpose. The chosen study cases are wildfires that took place in the Sidi Douma forest, Saida, Algeria on August 2-3, 2017. A pre-fire Landsat-8 product is used to generate SMC-related models and indices, namely Temperature Vegetation Dryness Index, Perpendicular Drought Index, Optical Trapezoidal Model, and the Modified Normalized Difference Water Index. The outputs of those models are input to a Gaussian Naive Bayes classifier, which produced 0.70 accuracy, 0.70 precision, 0.69 F1 score, and 0.69 AUC score. The classification is subsequently used to produce a wildfire risk assessment map that is validated and analyzed via the differences in Normalized Burn Ratio between the pre-fire and post-fire Landsat-8 products.
MoreTranslated text
Key words
Live fuel moisture content,soilmoisture content,wildfires,Gaussian naive Bayes,temperature vegetation dryness index,perpendicular drought index,optical trapezoidal model
AI Read Science
Must-Reading Tree
Example
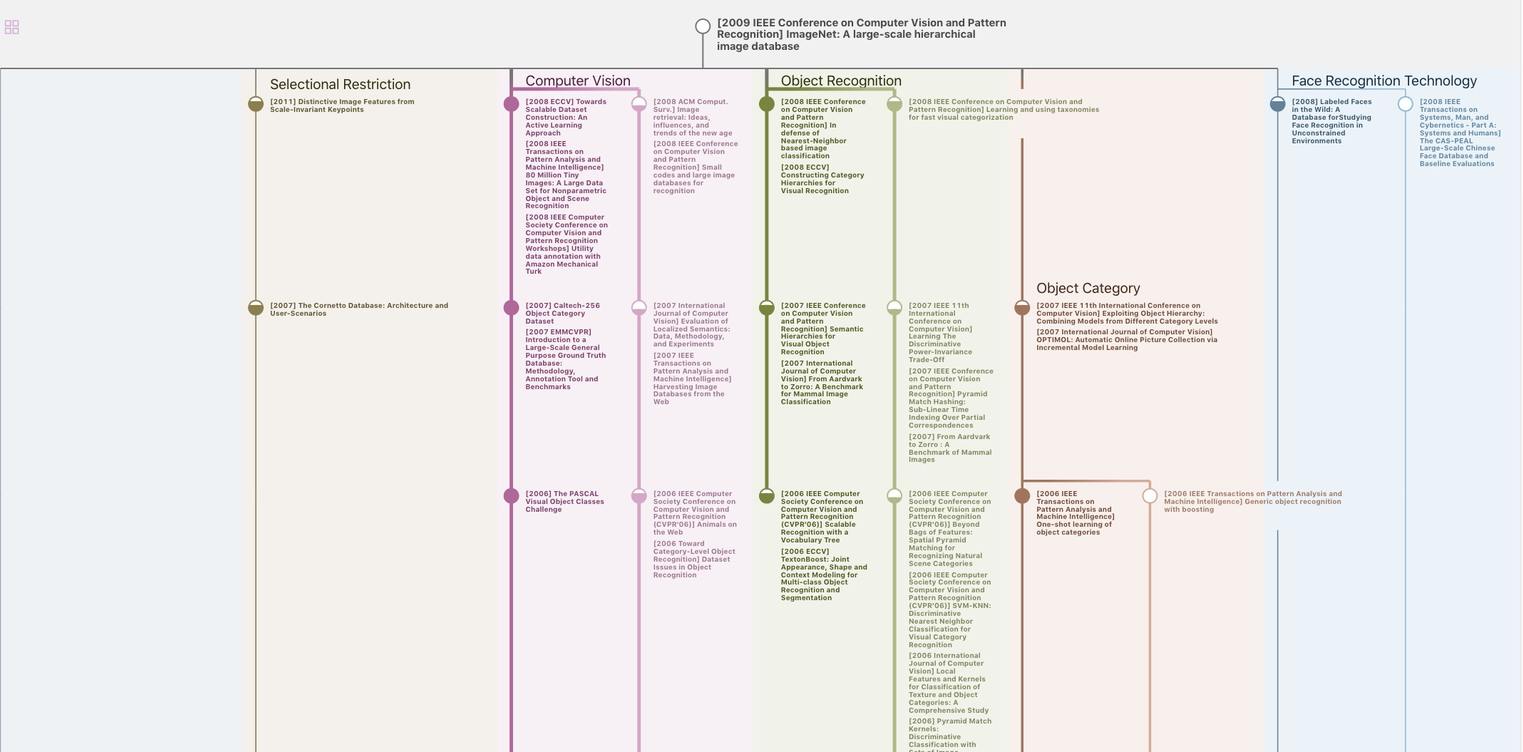
Generate MRT to find the research sequence of this paper
Chat Paper
Summary is being generated by the instructions you defined