Lai time series reconstruction from sentinel-2 imagery using vegetation growing phenology feature
IGARSS 2023 - 2023 IEEE INTERNATIONAL GEOSCIENCE AND REMOTE SENSING SYMPOSIUM(2023)
摘要
As an essential input parameter, Leaf Area Index (LAI) plays significant value in global climate models.. Acquisition of LAI products with extensive long-term series is crucial for various applications. Presently, numerous remote sensing inversion algorithms have been proposed for the generation of LAI products. However, the low temporal resolution is a huge challenge for producing LAI at a medium to high spatial resolution scale. In addition, the cloud has resulted in a significant reduction of accessible data. In this study, a LAI reconstruction method considering vegetation phenological period was developed. A total of 241 Sentinel-2 images from Saihanba in northern China and cloud probability data provided by Sentinel Hub were collected. The results indicate that the proposed method can effectively reconstruct LAI value in the data-missing period and areas. This study evaluated the performance of LAI reconstruction by implementing the "leave-one-out" method. The results demonstrate that the RMSE of LAI reconstruction is 0.5509 when using July 31st data as the validation dataset, and 0.3933 when using August 7th data as the validation dataset. In summary, this study demonstrates the potential for the LAI time series reconstruction from high-resolution data.
更多查看译文
关键词
Leaf area index (LAI),Reconstruction,Sentinel-2,Saihanba
AI 理解论文
溯源树
样例
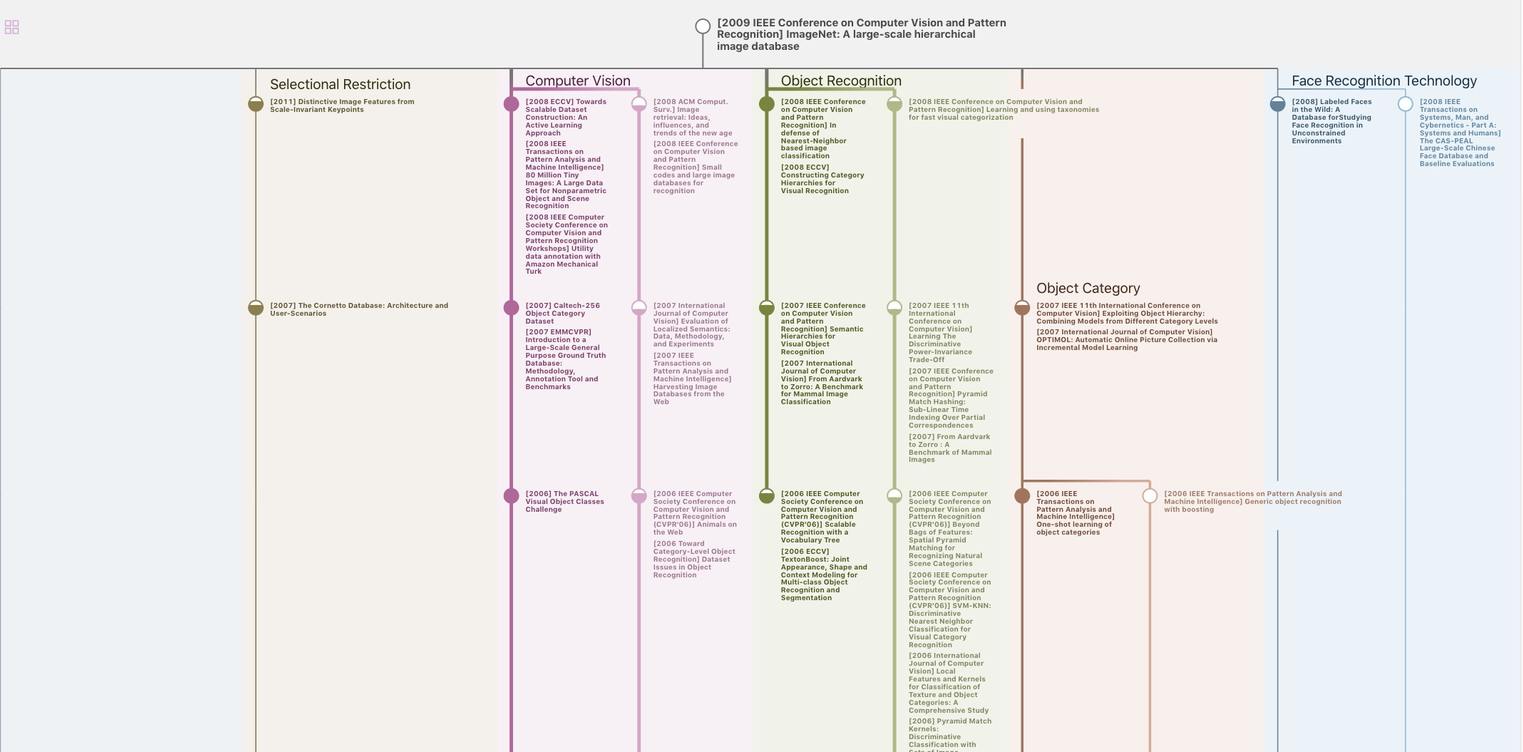
生成溯源树,研究论文发展脉络
Chat Paper
正在生成论文摘要