Onboard cloud detection and atmospheric correction with deep learning emulators
IGARSS 2023 - 2023 IEEE INTERNATIONAL GEOSCIENCE AND REMOTE SENSING SYMPOSIUM(2023)
摘要
This paper introduces DTACSNet, a Convolutional Neural Network (CNN) model specifically developed for efficient onboard atmospheric correction and cloud detection in optical Earth observation satellites. The model is developed with Sentinel-2 data. Through a comparative analysis with the operational Sen2Cor processor, DTACSNet demonstrates a significantly better performance in cloud scene classification (F2 score of 0.89 for DTACSNet compared to 0.51 for Sen2Cor v2.8) and a surface reflectance estimation with average absolute error below 2% in reflectance units. Moreover, we tested DTACSNet on hardware-constrained systems similar to recent deployed missions and show that DTACSNet is 11 times faster than Sen2Cor with a significantly lower memory consumption footprint. These preliminary results highlight the potential of DTACSNet to provide enhanced efficiency, autonomy, and responsiveness in onboard data processing for Earth observation satellite missions.
更多查看译文
关键词
Sentinel-2,Sen2Cor,onboard processing,atmospheric correction,cloud detection,deep learning,CNN
AI 理解论文
溯源树
样例
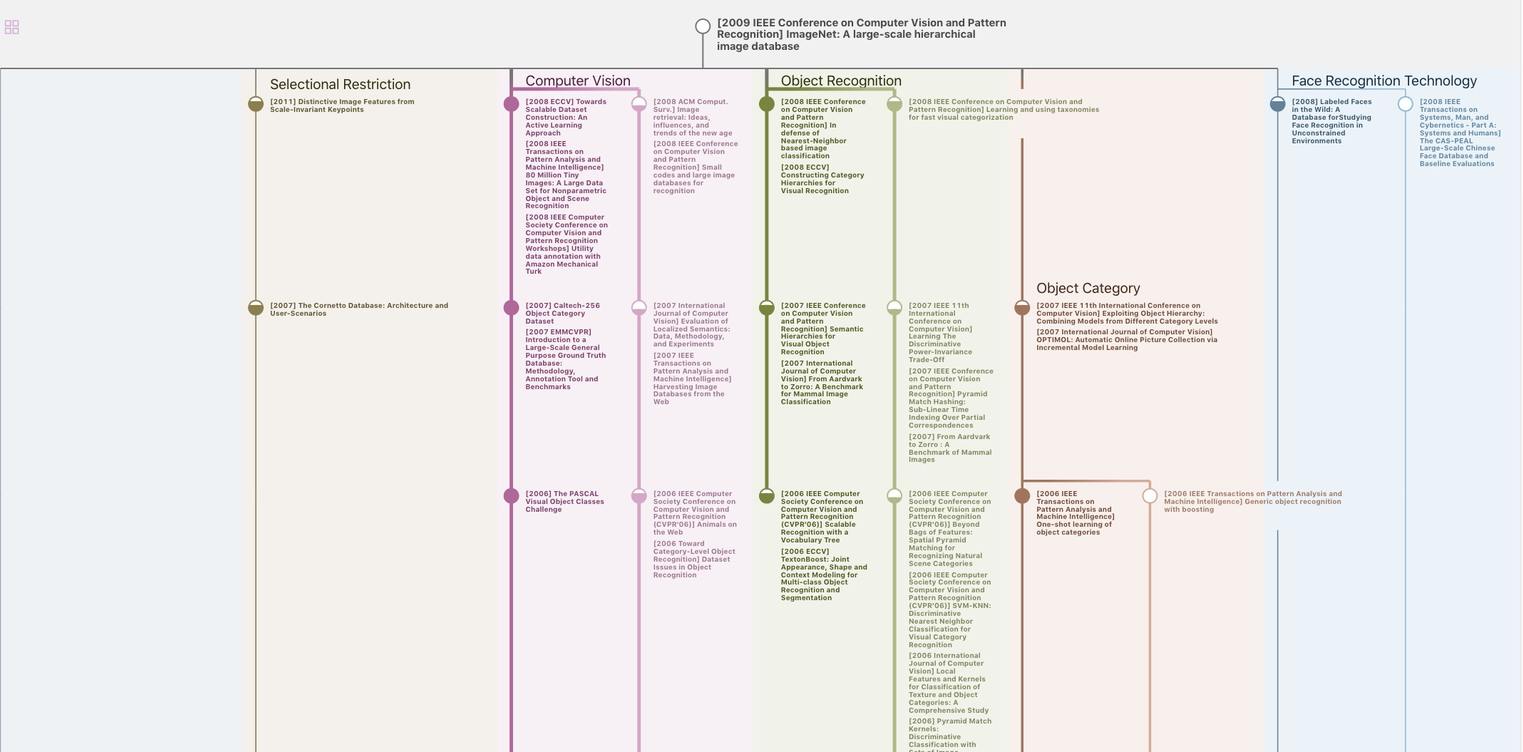
生成溯源树,研究论文发展脉络
Chat Paper
正在生成论文摘要