Developing a framework for rapid collapsed building mapping using satellite imagery and deep learning models
IGARSS 2023 - 2023 IEEE INTERNATIONAL GEOSCIENCE AND REMOTE SENSING SYMPOSIUM(2023)
Abstract
After a major disaster, a rapid assessment of building damage is highly required for emergency response and prompt recovery. Remote sensing technologies have been widely applied for building damage mapping. Combining machine-learning algorithms (e.g., deep learning) and satellite images has recently demonstrated success in boosting damage recognition methods. Although previous techniques have shown great success, they primarily adopt supervised settings, often requiring a minimum number of training samples to achieve acceptable accuracy. Moreover, previous methods also are developed for specific target areas, which makes it challenging to apply them to other regions in case of future disasters. This paper presents a novel unsupervised approach for building damage mapping, focusing on collapsed structures, using modern convolutional neural network (CNN) models and high-resolution remote sensing imagery. We apply our mapping framework to revise the building damage following the 2007 Peru-Pisco Earthquake and the recent 2023 Turkey and Syria Earthquakes.
MoreTranslated text
Key words
Building damage mapping,deep learning models,building footprint segmentation,2007 Peru-Pisco Earthquake,2023 Turkey and Syria Earthquakes
AI Read Science
Must-Reading Tree
Example
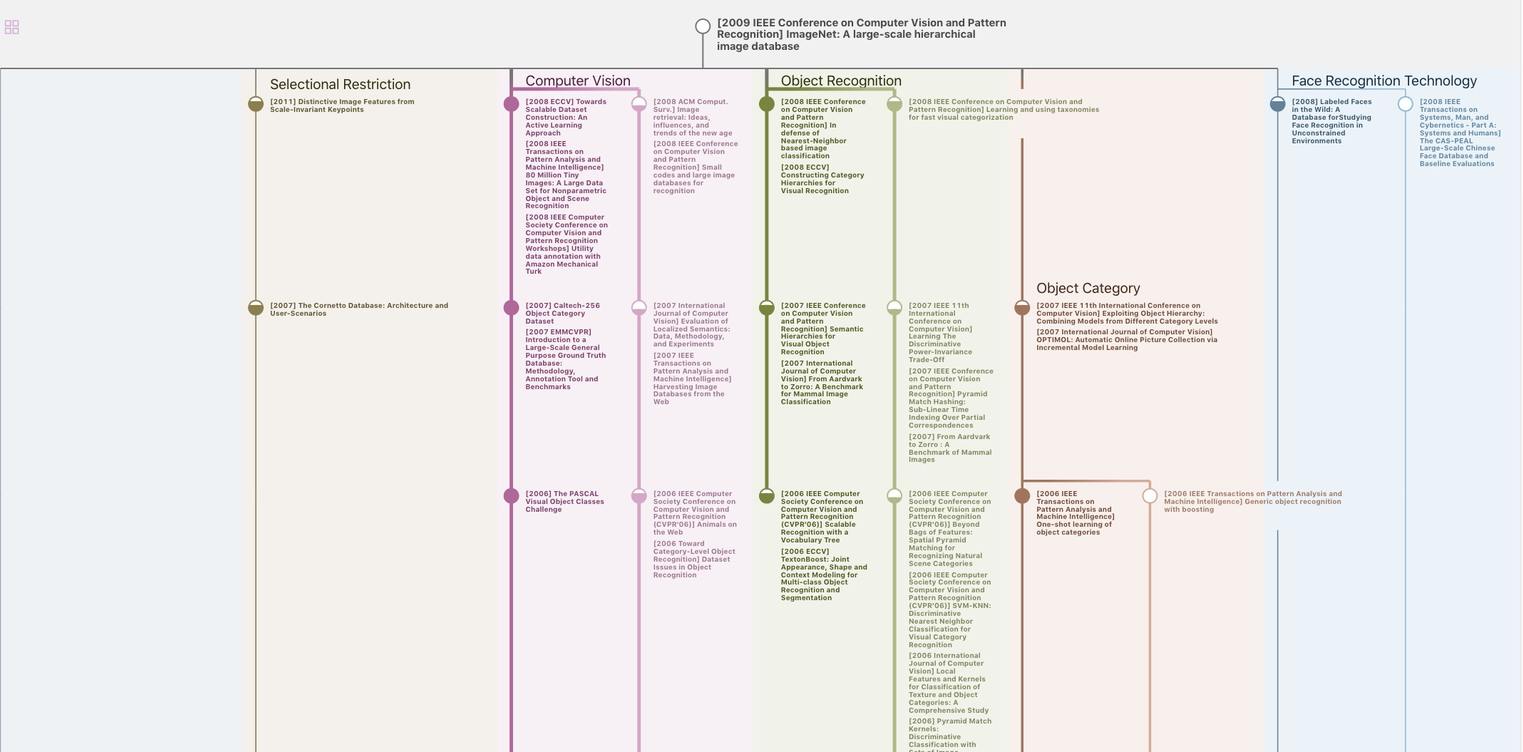
Generate MRT to find the research sequence of this paper
Chat Paper
Summary is being generated by the instructions you defined