Truncation of Perfectly Matched Layers Based on Recurrent Neural Networks in FDTD
2023 International Conference on Microwave and Millimeter Wave Technology (ICMMT)(2023)
摘要
To improve the efficiency of finite-difference method in time-domain (FDTD) for unbounded problems and to keep the stability of the late-time electromagnetic response, a method to truncate the interior of perfectly matched layers (PMLs) is proposed in this work. The method uses a recurrent neural network (RNN) to capture the time-marching electromagnetic field information in the FDTD and to complete the offline training of field components at the truncated interfaces of PMLs. During the computation in FDTD, the RNN simultaneously predicts the field components on the cells of the truncated interfaces at each time step online until the end. Since the outer region of the PML is truncated, the computational domain of the FDTD and the total number of unknowns are well reduced. Therefore, the proposed method can effectively improve the efficiency of FDTD. In addition, benefitting from the absorbing of the remaining PMLs to incident waves, the output electromagnetic fields are still stable in the late-time iterations and no error accumulation occurs. Numerical examples validate the proposed method, and the results show that there is an optimal truncation position for the traditional PMLs while balancing the accuracy and the efficiency.
更多查看译文
关键词
Absorbing boundary condition (ABC),finite-difference time-domain (FDTD),neural network,perfectly matched layer (PML)
AI 理解论文
溯源树
样例
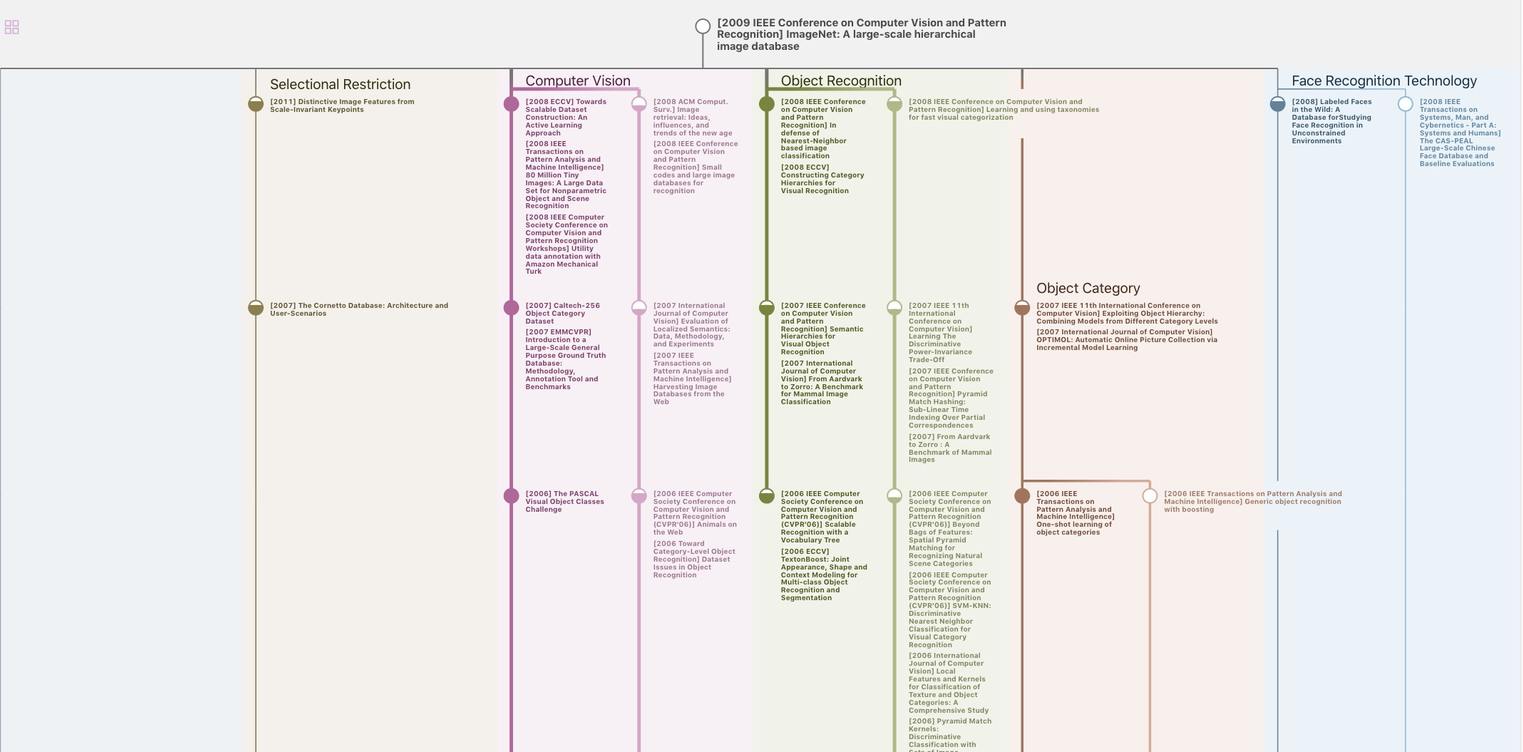
生成溯源树,研究论文发展脉络
Chat Paper
正在生成论文摘要