A Hybrid Enhancing Aspect-Based Sentiment Analysis with BERT for Aspect Extraction and Diverse ML Classifiers
2023 International Conference on Network, Multimedia and Information Technology (NMITCON)(2023)
摘要
Social media involvement in human life is becoming huge where people can express their feelings and opinions. Sentiment analysis (SA) is a method of analysing, handling, concluding, and inferencing subjective texts with the sentiment. Aspect-Based Sentiment Analysis (ABSA) acts an important part in understanding people's opinions and sentiments towards various aspects of products or services. The main key challenges faced in ABSA are product dependent, target dependent, aspect dependent. In this work, a hybrid approach that combines the power of BERT for aspect extraction and diverse ML classifiers for SA. First, a set of diverse ML classifiers used to analyze the sentiment associated with each extracted aspect. By utilizing multiple classifiers, we aim to enhance the robustness and generalization of sentiment classification. Each classifier is trained on a different feature representation, such as PMI, bag-of-words and TF-IDF to capture various aspects of the textual data. Later, BERT leveraged to identify and extract relevant aspects from text. BERT's contextualized embeddings extracts the meaning of words in the context of the sentence, enabling accurate aspect extraction. To evaluate the proposed hybrid approach, trained model is experimented on three benchmark datasets which are based on Amazon reviews, Twitter and TripAdvisor datasets. Our results demonstrate that the combination of BERT for aspect extraction and SVM classifier significantly improves the accuracy of 96.91%, 95.63%, 95.66% on three datasets compared to traditional approaches.
更多查看译文
关键词
ABSA,BERT,Hybrid model,Transformers,ML,BoW,Term Frequency,TFIDF,SVM
AI 理解论文
溯源树
样例
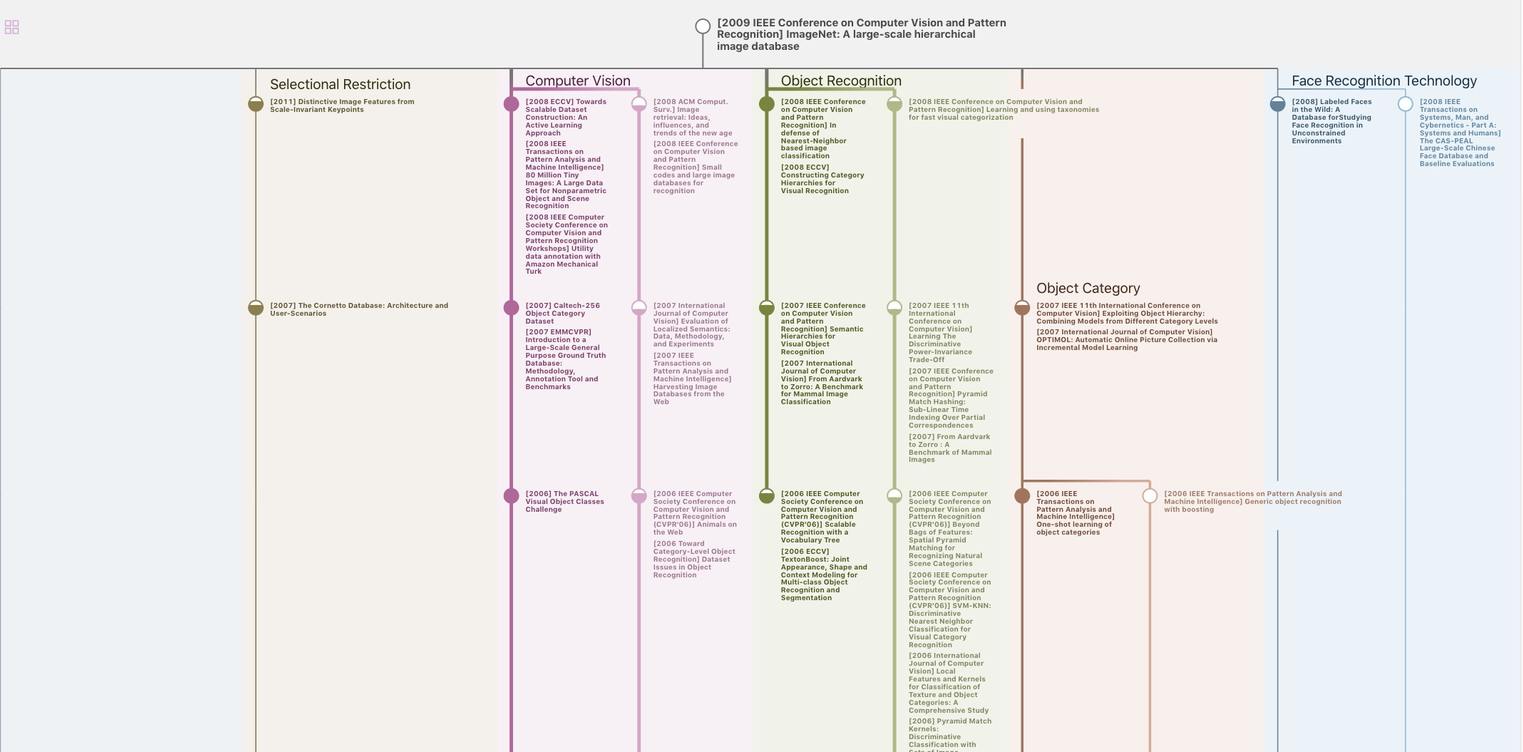
生成溯源树,研究论文发展脉络
Chat Paper
正在生成论文摘要