Day-Ahead Forecasting of Solar Irradiance & PV Power Output Through Statistical Machine Learning Methods
2022 Saudi Arabia Smart Grid (SASG)(2022)
摘要
Energy production from solar photovoltaic (PV) plants is unpredictable, mainly due to the stochastic formation and movement of clouds or aerosol - dust particles which scatter or disperse solar radiation. Accurate forecasts of PV output are essential to Distribution and Transportation System Operators as they assist efficient solar energy trading and management of electricity grids. This work evaluates an autoregressive, computationally-light KNN-regression scheme (TSFKNN) for hourly, day-ahead forecasts of solar irradiance and energy yield of various PV technologies. The model is being tested and validated using data measured in Thuwal, Saudi Arabia. The available measured records span a 60-month period. The developed forecasting models are designed for online systems and provide increased levels of accuracy and low computational cost. Several parametric and nonparametric specifications, coupled with conventional versus outlier-robust estimation procedures are tested, in order to derive an optimal month-specific daily profile (MDP). Current results demonstrate that including intraday variability to the monthly-based irradiance models achieve improved predictive accuracy between 10% and 25% on average.
更多查看译文
关键词
Solar energy output forecasting,Time series KNN,Ensemble modeling,Statistical Machine Learning
AI 理解论文
溯源树
样例
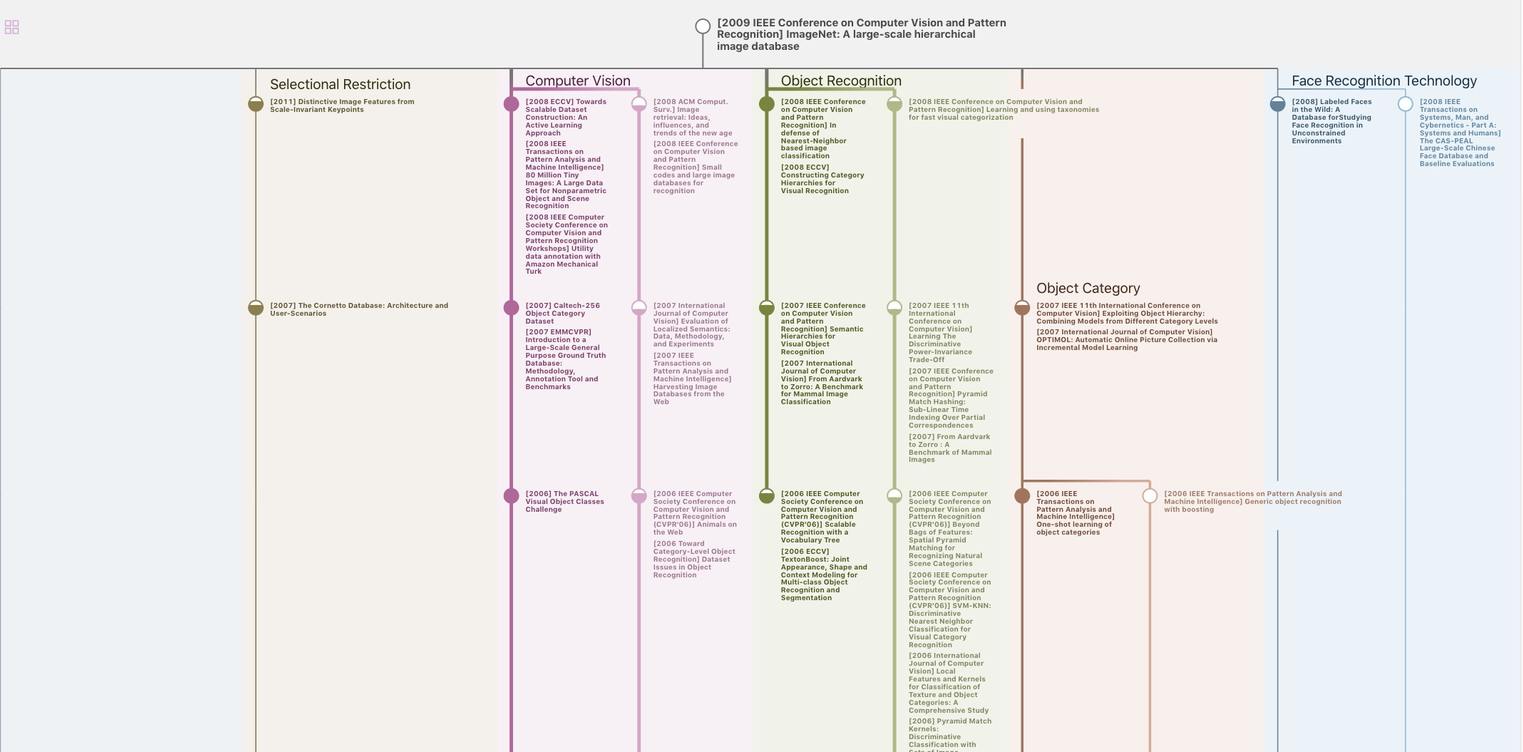
生成溯源树,研究论文发展脉络
Chat Paper
正在生成论文摘要