Adaptive Edge-Level Personalization on Hierarchical Federated Learning
2023 IEEE International Performance, Computing, and Communications Conference (IPCCC)(2023)
摘要
Federated learning faces the challenge of non-IID data distribution while the global model doesn’t achieve well for individual clients. To address this challenge, we propose hierarchical personalized federated learning (HPFL) and achieve edge-level personalization, which overcomes non-IID data distribution and alleviates the overfitting of the pesonalization process. The three-layer framework makes the learning and personalization process more feasible than traditional two-layer federated learning since real-world edge servers usually have sufficient computing power than local clients and have efficient communication with the cloud server. In our approach, the personalized model on each edge server is generated by mixing the global model and edge model, which can be updated based on adaptive mixing parameter and optimized after multiple iterations. Experiments on MNIST and FMNIST show that the proposed HPFL overcomes non-IID data distribution, achieves comparable performance to traditional two-layer personalization APFL and outperforms HierFAVG under non-IID data setting.
更多查看译文
关键词
federated learning,personalized federated learning,hierarchical federated learning,non-IID data
AI 理解论文
溯源树
样例
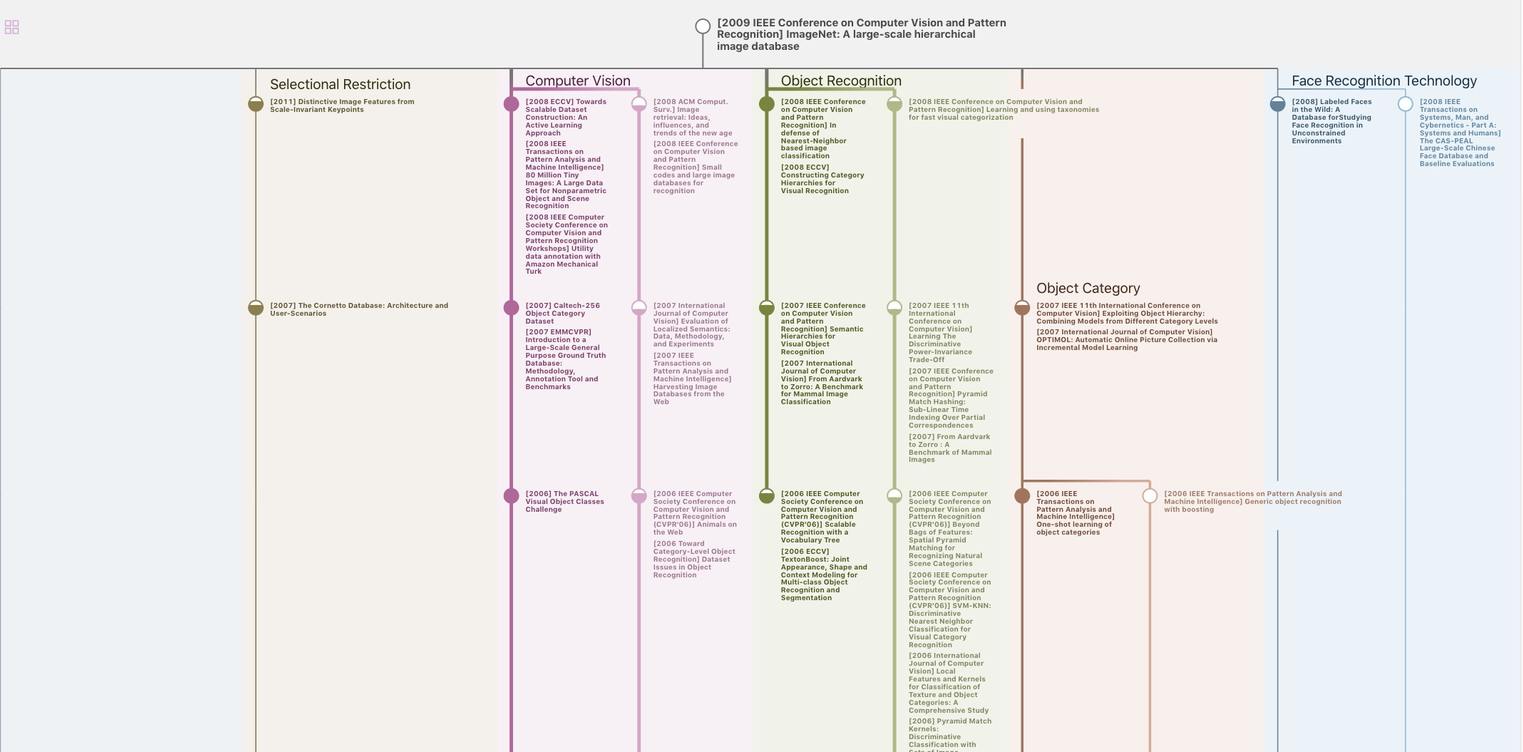
生成溯源树,研究论文发展脉络
Chat Paper
正在生成论文摘要