An Adaptive Sampling Strategy for Federal Graph Neural Networks in Internet of Things
2023 IEEE International Performance, Computing, and Communications Conference (IPCCC)(2023)
摘要
The Internet of Thing systems have contributed a considerable scale of graphs via numerous devices, like the network topology. These graph data are used in wireless communication and mobile computing for a variety of tasks such as traffic prediction and vehicle communication. Therefore, the analysis of these graph data has shown great significance towards the management of IoTs. Compared to other distributed computing scenarios, IoTs require a wider range and more frequent transmission of data. Considering the constrained communication resources and concerns about data privacy, IoT devices tend to keep the graph data locally. Therefore, it is meaningful to adopt the idea of Federal Graph Neural Network for IoTs, where the fancy Graph Neural Network can be implemented in a distributed manner over graphs in IoTs. This paper proposes a sampling-based framework towards the purpose. It assumes the graphs are vertically partitioned over devices, which means all devices hold an identical set of vertices and edges, and each owns a subset of features. It fits the fact that different devices own heterogeneous sensing capabilities. Then a sampling-based method is proposed for multi-round training of Federal Graph Neural Network, and only partial devices join in the training in each round. The sampling strategy applies the local accuracy of validation of each device to adjust the sampling probabilities accordingly, so as to accelerate the convergence of the global model. Finally, extensive analysis and numerical evaluation verify the advancement of the framework.
更多查看译文
关键词
Federated Learning,Graph Neural Network,Sampling Strategy
AI 理解论文
溯源树
样例
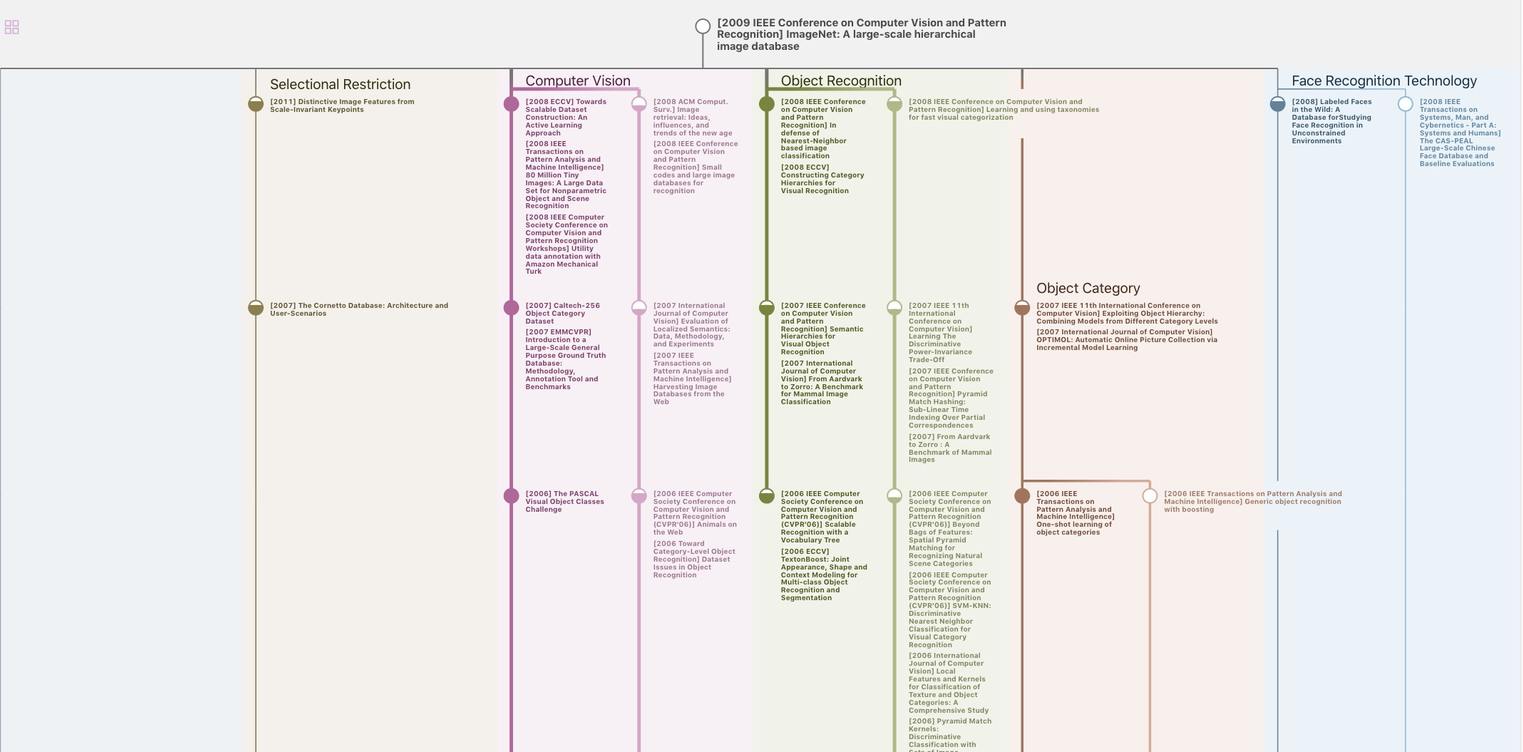
生成溯源树,研究论文发展脉络
Chat Paper
正在生成论文摘要