Novel quadratic time-frequency features in EEG signals for robust detection of epileptic seizure
Research on Biomedical Engineering(2023)
Abstract
Purpose Epilepsy is a chronic neurological disorder characterized by recurrent convulsions. Therapists seek to recognize epilepsy patterns in Electroencephalogram (EEG) signals through visual inspection. However, this procedure is costly, time-consuming, and sensitive to bias. Therefore, in this proof-of-concept investigation, we developed an automatic method of epileptic seizure detection. The deployed approach includes novel time-frequency features of EEG data. Methods We evaluated the performance of the developed algorithm using the CHB-MIT scalp EEG database and the TUH EEG Corpus database. We compute time-frequency representations of EEG data through Quadratic Time-Frequency Distributions (QTFDs), namely, the Smoothed Pseudo Wigner-Ville distribution (SPWVD), the Choi-Williams distribution (CWD), and the spectrogram (SP). We developed an approach for detecting epileptical brain activity by extracting relevant features from the obtained time-frequency representation. We analyzed EEG signals in four frequency bands in accordance to brainwaves bandwidths, namely, Delta (0.4 up to 4 Hz), Theta (4 up to 8 Hz), Alpha (8 up to 12 Hz), Beta (12 up to 30 Hz), and Gamma (above 30 Hz). Thus, we located the contour of the time-frequency area to define features within the time-frequency plane. We provided the extracted time-frequency features as inputs for two classifiers: the support vector machine (SVM) with RBF kernel function and the k-nearest neighbors (kNN) for comparison purposes. We defined seven statistical parameters, sensitivity (Se), specificity (Sp), the overall classification accuracy (Acc), precision (Prec), False alarm rate (FPR), F-measure score (F1-score), and Area under the curve (AUC). Results The experimental results obtained from the both databases show that the proposed approach provides very encouraging results. Indeed, we can reach precision rates of 99.29% when using the Smoothed Pseudo Wigner-Ville distribution (SPWVD) combined with the kNN classifier and a precision rate of 97.67% for the Choi-Williams distribution (CWD) combined with a SVM classifier, with an average area under the curve (AUCs) of 0.9911 and 0.9701 respectively. The spectrogram (SP) yields fewer performance results for both classifiers at a rate not exceeding 95.88% with an area under the curve of 0.9542. Conclusion We developed an efficient approach for recognizing epileptic seizures. This approach localizes the contour area at five levels on time-frequency representations of EEG signals using three quadratic time-frequency distributions. Compared to the Spectrogram (SP) and the Smoothed Pseudo Wigner-Ville distribution (SPWVD), the Choi-Williams distribution (CWD) has proved its effectiveness with high precision and complete sensitivity, indicating a powerful method for identifying epileptic seizures from EEG signals.
MoreTranslated text
Key words
Electroencephalogram, Epilepsy, Seizure, Spectrogram, Smoothed pseudo Wigner-Ville distribution, Choi-Williams distribution, Support vector machine, K-nearest neighbors, Classification
AI Read Science
Must-Reading Tree
Example
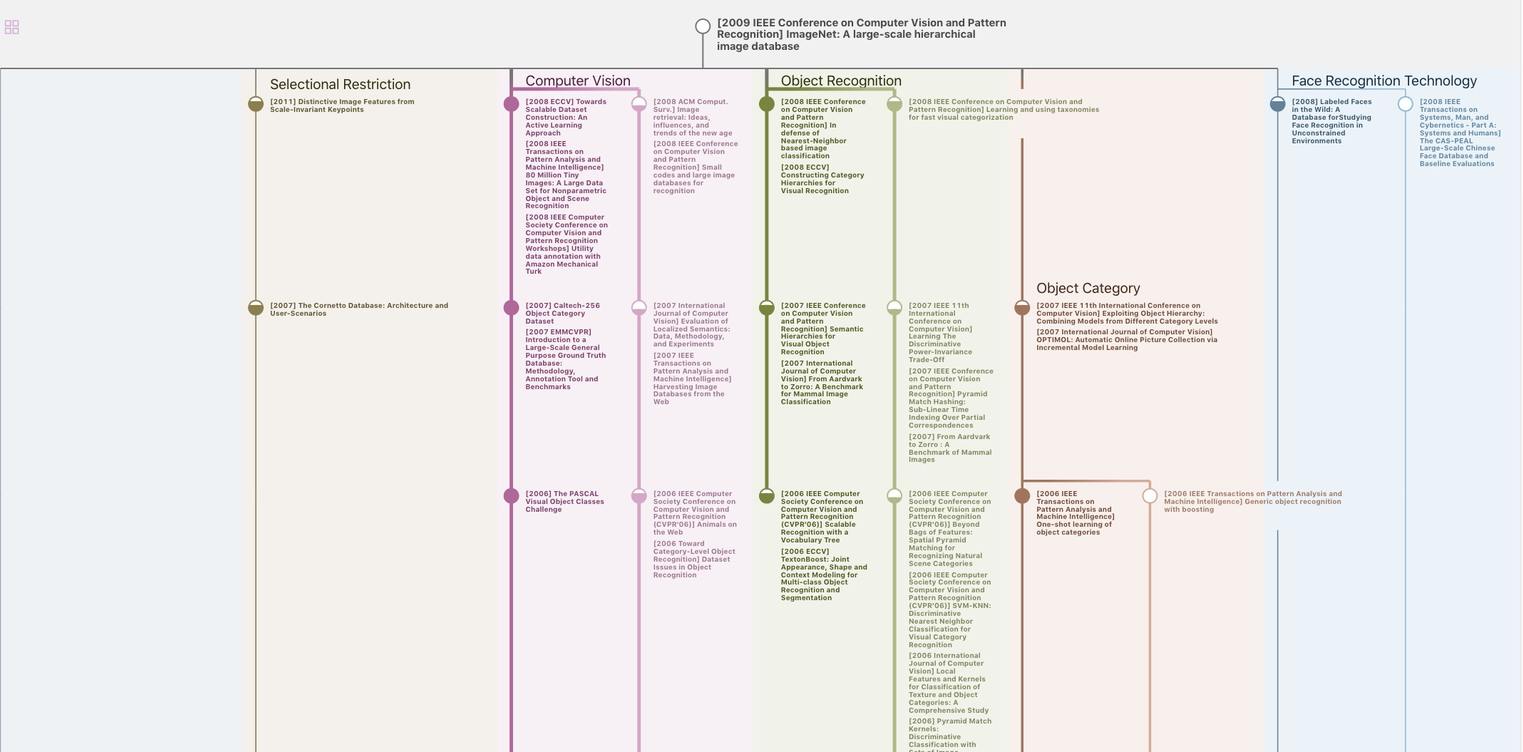
Generate MRT to find the research sequence of this paper
Chat Paper
Summary is being generated by the instructions you defined