Reinforcement Learning for the Just-in-Time Job-Shop Scheduling Problem
2023 9th International Conference on Control, Decision and Information Technologies (CoDIT)(2023)
摘要
This paper focuses on the optimization of job-shop scheduling in industrial production systems in the just-in-time configuration. The scheduling function plays a crucial role in determining the performance and competitiveness of a company, especially with the increasing demand for timely product delivery. The main challenge in job-shop scheduling is to find the optimal scheduling of multiple jobs on several machines, taking into account their due dates and processing times. The earliness-tardiness model adds another layer of complexity to the problem by considering the impact of delivering jobs early or late, incurring inventory costs or tardiness penalties, respectively. Due to the multiple constraints and objectives involved, finding the optimal solution is a challenging task. To tackle this problem, we in this paper investigate the use of recent advances in deep reinforcement learning techniques to optimize the earliness-tardiness cost in the job-shop. The experimental results showed that the proposed method performed at least as good as the state of the art in 65% of the studied instances, while strictly improving 10% of the same benchmark.
更多查看译文
关键词
earliness-tardiness model,industrial production systems,just-in-time job-shop scheduling problem,optimization,reinforcement learning
AI 理解论文
溯源树
样例
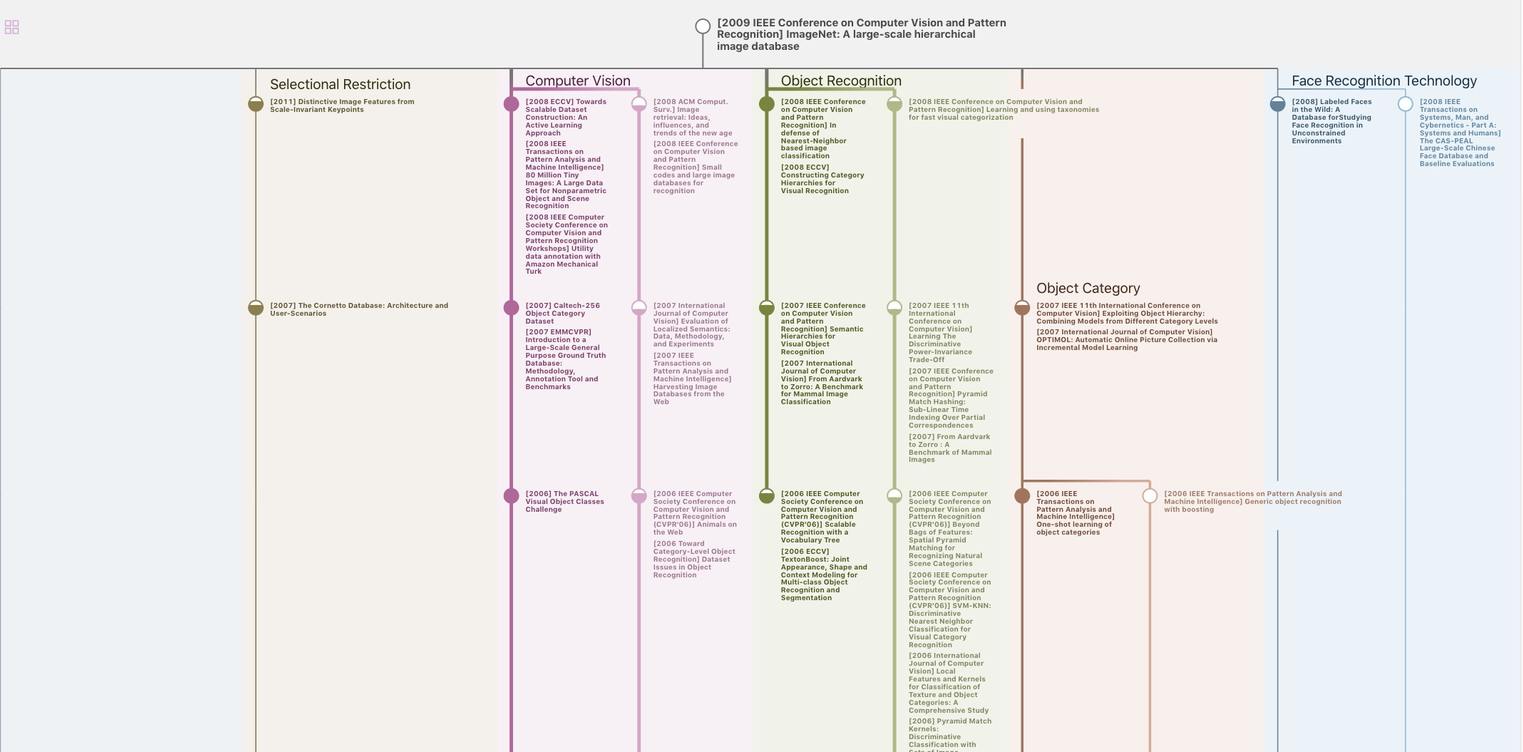
生成溯源树,研究论文发展脉络
Chat Paper
正在生成论文摘要