Collective Driver Attention: Towards a Comprehensive Visual Understanding of Traffic Scenes
2023 9th International Conference on Control, Decision and Information Technologies (CoDIT)(2023)
摘要
Thanks to state-of-the-art deep learning-based methods for driver's attention prediction, it becomes possible to estimate where drivers look at in different traffic scenes. However, such estimation only takes into account visual information of the front view from a single vehicle. To remedy the lack of comprehensiveness of this approach, modern advanced driver-assistance systems (ADAS) further incorporate individual-specific features, including blood pressure and heart rate, to provide more precise safety advice. Nonetheless, there is still room for the improvement of safety-related recommendations by means of predicting collective drivers' attention. Specifically, the conceptual idea presented in this work is based on integrating visual understanding of the surrounding environment of a vehicle, driver-specific information and estimated attention in order to create a collective knowledge of the road, obstacles, distraction points, pedestrians and drivers' consciousness level from viewpoints of several drivers to predict a holistic attention map. Then, such a 360-degree attention map enables drivers to being aware not only of their front view, but also of back view and around (left and right) sides to help them prevent accidents and keep away from obstacles. The proposed framework takes advantages of edge and cloud computing for processing real-time information and large-scale computing, respectively. This work is intended to open a broader window towards the development of next generation of networked ADAS systems by employing several heterogeneous sources of information, implicit participation of drivers and their visual understanding and reasoning.
更多查看译文
关键词
Driver attention prediction,ADAS,intelligent transportation,intelligent vehicle,saliency estimation
AI 理解论文
溯源树
样例
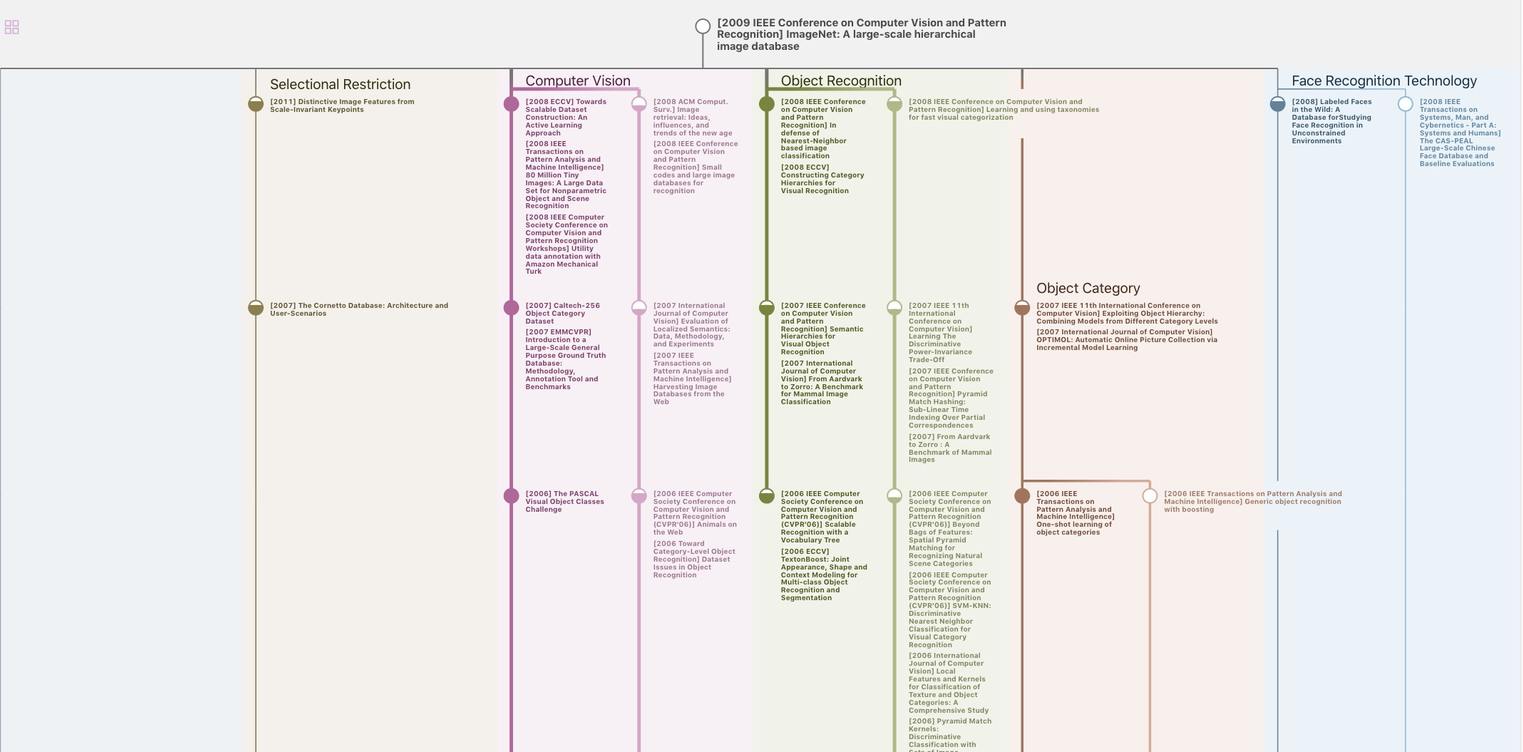
生成溯源树,研究论文发展脉络
Chat Paper
正在生成论文摘要