Short-term Forecasting of Non-Conforming Net Load Using a Fusion Model with Machine Learning and Deep Learning Methods
2023 9th International Conference on Control, Decision and Information Technologies (CoDIT)(2023)
Key words
Net load forecast,machine learning,deep learning,non-conforming net loads,distributed energy resources,behind the meter supply resources
AI Read Science
Must-Reading Tree
Example
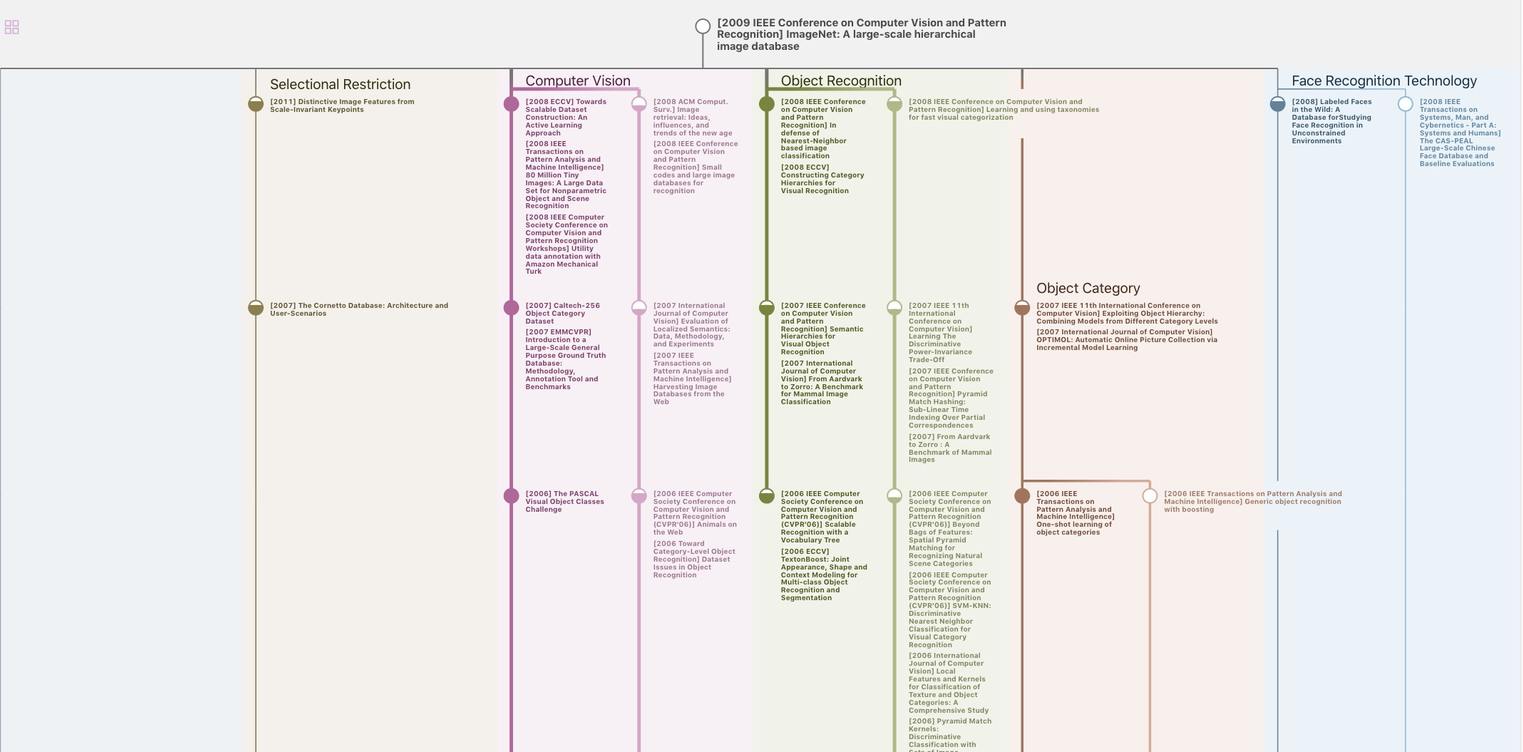
Generate MRT to find the research sequence of this paper
Chat Paper
Summary is being generated by the instructions you defined