Estimating landslide hazard distribution based on machine learning and bivariate statistics in Utmah Region, Yemen
Natural Hazards(2024)
摘要
Landslides represent significant risks to human activity, leading to infrastructure damage and loss of life. This study focuses on assessing landslide hazards in Utmah Region, Yemen. The evaluation involves comparing the effectiveness of the relative frequency ratio model with five machine learning algorithms (MLAs) for hazard mapping. Field surveys, high-resolution satellite imagery, and aerial photography were utilized in the study. The inventory map was generated after identifying and mapping 100 landslides. The inventory was then divided randomly into a training dataset (70 landslides) and a validation dataset (30 landslides), with an equal number of non-landslide pixels. Eleven additional landslide conditioning factors were collected from various sources, and the frequency ratio (FR) approach was employed to identify the most crucial variables for modeling. The models were rigorously tested and assessed using statistical metrics, including the Friedman and Wilcoxon signed-rank tests, as well as the area under the receiver operating characteristics (AUROC) curve. The findings based on the training and validation datasets revealed that the RF algorithm (AUROC, 0.992) outperformed the other models in generating hazard maps. The XGBoost model (AUROC, 0.991), NB model (AUROC, 0.970), ANN model (AUROC, 0.922), KNN model (AUROC, 0.877), and FR (AUROC, 0.674) were found to be less effective. Consequently, the study highlights that the Random forest (RF) model shows promise as an effective approach for predicting landslides spatially on a global scale.
更多查看译文
关键词
Predictive modeling,Remote sensing,Geographic information system (GIS),Environmental factors,Landslide inventory
AI 理解论文
溯源树
样例
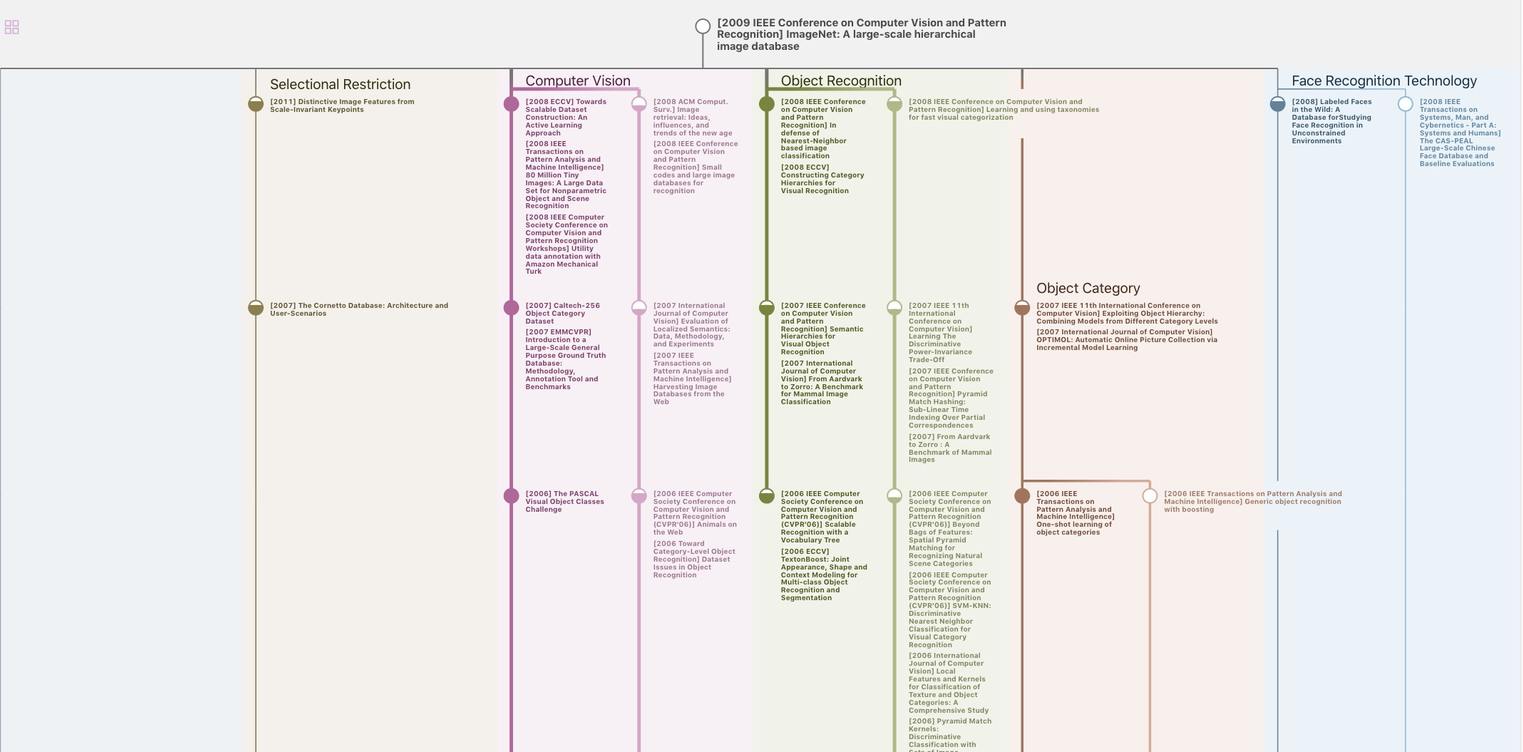
生成溯源树,研究论文发展脉络
Chat Paper
正在生成论文摘要