Distributed few-shot learning with prototype distribution correction
Applied Intelligence(2023)
摘要
Few-shot learning aims to learn a classifier that can perform well even if a few labeled samples are used for training. Many methods based on prototype models have recently been proposed and show good performance in few-shot learning. However, in the few-shot scenario, data is scarce. There is a deviation between the prototype calculated employing only a small amount of data in the support set and the actual prototype. Moreover, the features of the novel class extracted from the pre-trained model trained using base class data exhibit a bias. Additionally, the pre-trained model demonstrates high complexity and poor training efficiency. Therefore, we propose the Distributed Few-shot Learning with Prototype Distribution Correction to this end. Specifically, we employ pseudo-labels to fuse the sample features of the query set and modify the prototype to reduce the bias between the support and the query set. Then we utilize the features of the Gaussian distribution to transfer the base class features to the novel class to reduce the bias between novel classes and base classes. Finally, we combine distributed learning to improve the efficiency of the pre-trained model. We evaluate our method on Mini-ImageNet, Tiered-ImageNet, and CUB, three few-shot learning public datasets. The classification accuracy of our method improves in every case on every dataset and achieves state-of-the-art performance. In particular, the accuracy improves by 10.12% on 1-shot Mini-ImageNet.
更多查看译文
关键词
Few-shot learning,Prototype correction,Distribution correction,Distributed learning
AI 理解论文
溯源树
样例
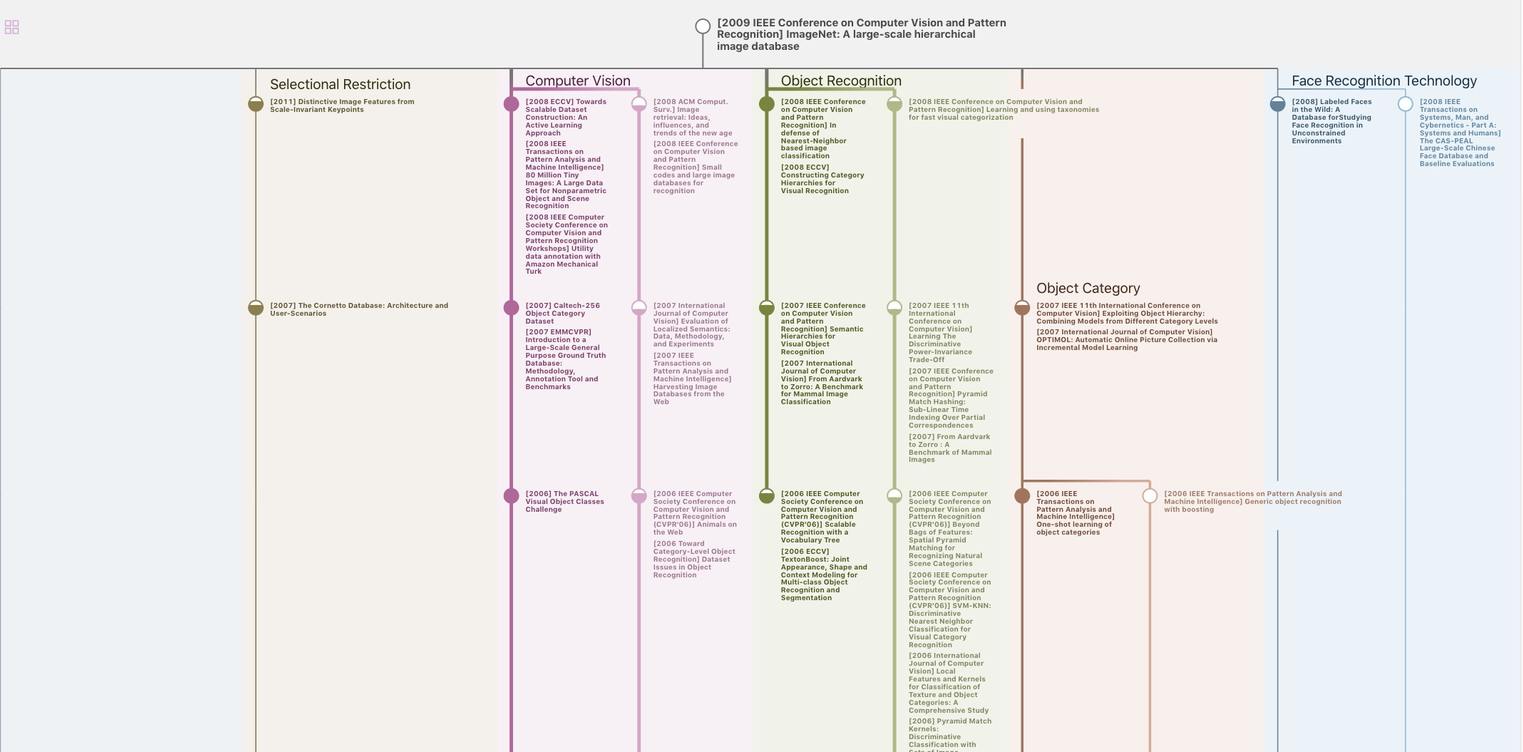
生成溯源树,研究论文发展脉络
Chat Paper
正在生成论文摘要