Classifying Rock Fragments Produced by Tunnel Boring Machine Using Optimized Convolutional Neural Network
Rock Mechanics and Rock Engineering(2024)
摘要
Grain size and uniformity index of fragments produced by tunnel boring machine (TBM) reflect tunneling states. Traditional manual fragment identification methods will reduce tunneling safety and efficiency. Therefore, an automatic fragment classification method is significant. However, the fragments classification accuracy of existing deep learning methods will decrease under different tunnel projects with various kinds of fragments. In this paper, a rock fragment classification method is proposed based on convolutional neural network (CNN). To distinguish fragment and background with very similar grey level in the image, contrast limited adaptive histogram equalization is used to enhance fragment edge features. In addition, image regeneration is used in data preparation process to balance data set. During hyperparameter tuning in model training, this research applies Bayesian optimization to acquire optimum model. Experiments show that proposed CNN model can acquire an accuracy of 91.88% in classifying various types of fragments, improving 39.21%, 11.64%, 13.64% and 9.45%, respectively, compared with LeNet, ResNet, VGG and AlexNet. Batch normalization, DropBlock and global average pooling skills are used to alleviate overfitting of CNN model. Based on proposed model pre-trained on a single project data set and a small amount of new data, the migrated model can achieve 89.86% accuracy on a new tunnel data set. The experiment results demonstrate a great generalization ability of proposed model dealing with various kinds of fragments.
更多查看译文
关键词
Tunnel boring machine,Rock fragment classification,Convolutional neural network,Deep learning,Bayesian optimization
AI 理解论文
溯源树
样例
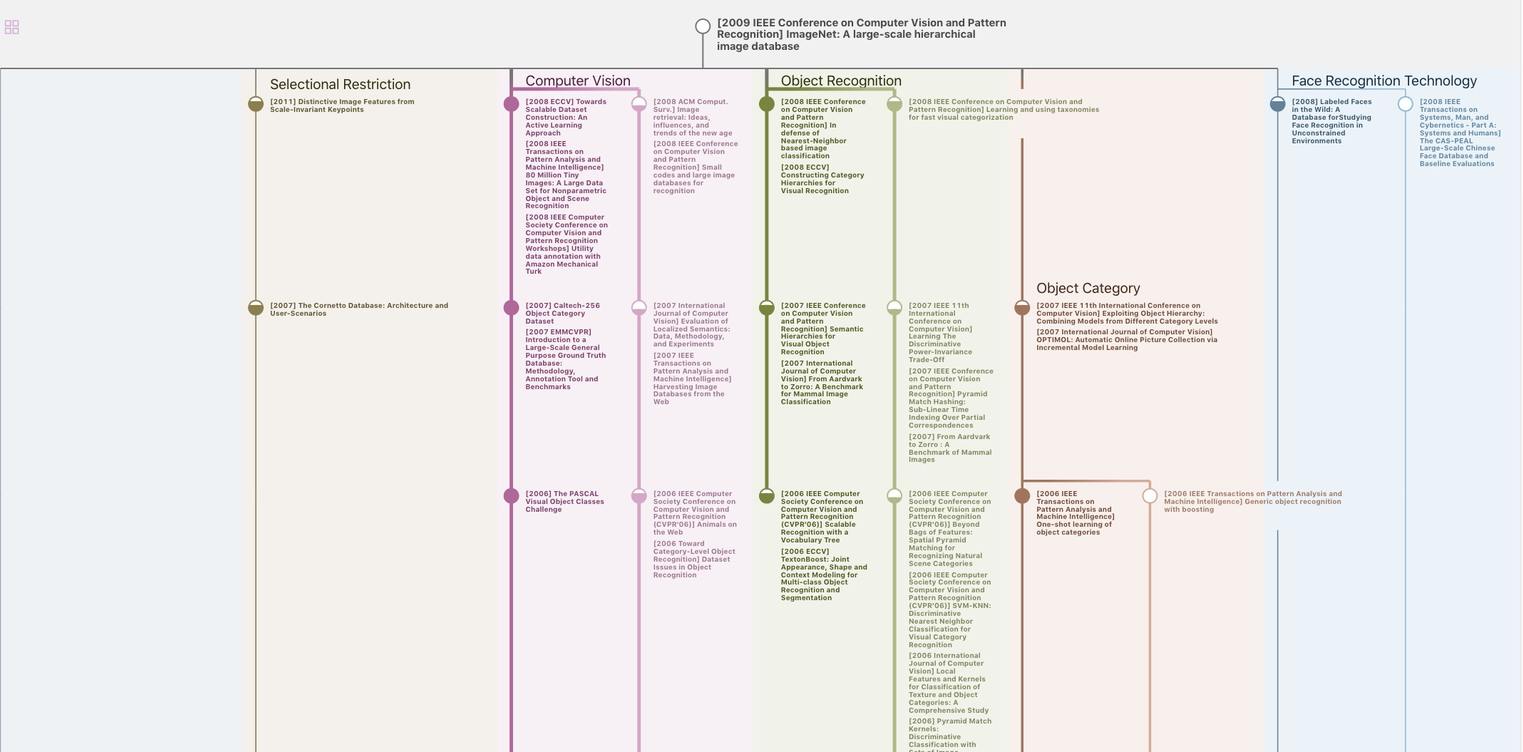
生成溯源树,研究论文发展脉络
Chat Paper
正在生成论文摘要