A Graph Attention Network-Based Model for Anomaly Detection in Multivariate Time Series
JOURNAL OF SUPERCOMPUTING(2024)
关键词
Anomaly detection,Multivariate time series,Graph neural network,Similarity constraint
AI 理解论文
溯源树
样例
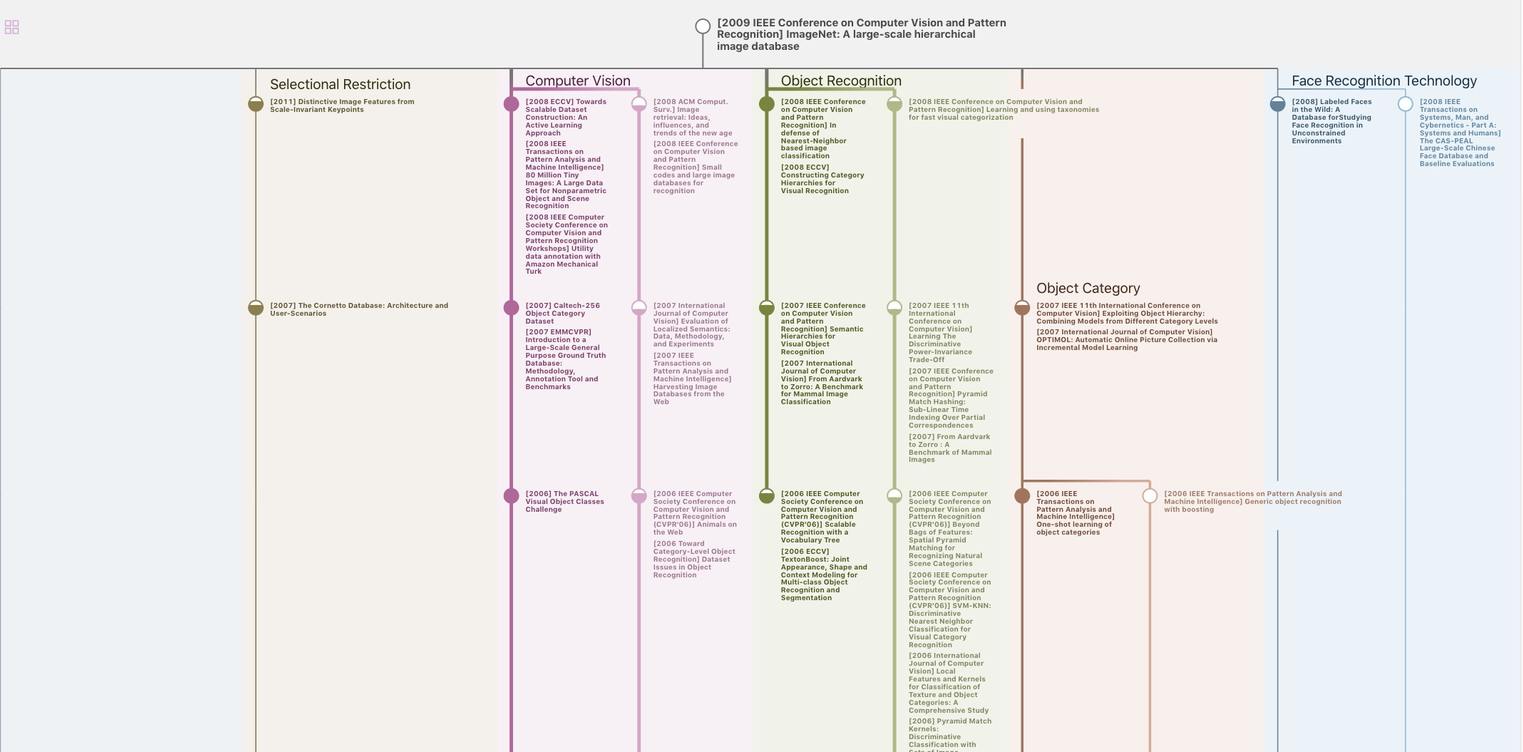
生成溯源树,研究论文发展脉络
Chat Paper
正在生成论文摘要