Correction to: An optimized boosting framework for skin lesion segmentation and classification
Multimedia Tools and Applications(2024)
摘要
Skin Lesion (SL) prediction and segmentation is the trending topic in the medical imaging industry for finding different disease severity. But the un-cleared pixels in the image data might reduce the image features analyzing exactness that has resulted from average or poor segmentation accuracy. Thus the traditional method is not succeeding in this specific domain. Hence, proper preprocessing is required to filter noise features at a high level, which has led to attaining the expected SL segmentation classification exactness score. To address these issues, the current study intended to implement a novel Sailfish-based Gradient Boosting Framework (SbGBF) for recognizing and segmenting the SL region with a high exactness rate. The boosting mechanism is rich with noise removal features; hence, this study considers the optimized boosting mechanism. The boosting parameters were initially activated to eliminate the noise variables in the trained SL data. Then the sailfish fitness function is processed for tracing the region features in the preprocessed SL images, and the segmentation is performed. Finally, the SL types were classified, and the processing outcomes were measured. The recorded SL segmentation and classification accuracy by a novel SbGBF is 98%; a 0.02% error score was also reported. In addition, the recorded minimum computational complexity score is 3 s, which is quite less than the compared associated models.
更多查看译文
关键词
Skin lesion (SL),Segmentation and classification,SailFish optimizer (SFO),Gradient boosting (GB),Feature analysis,Machine learning (ML)
AI 理解论文
溯源树
样例
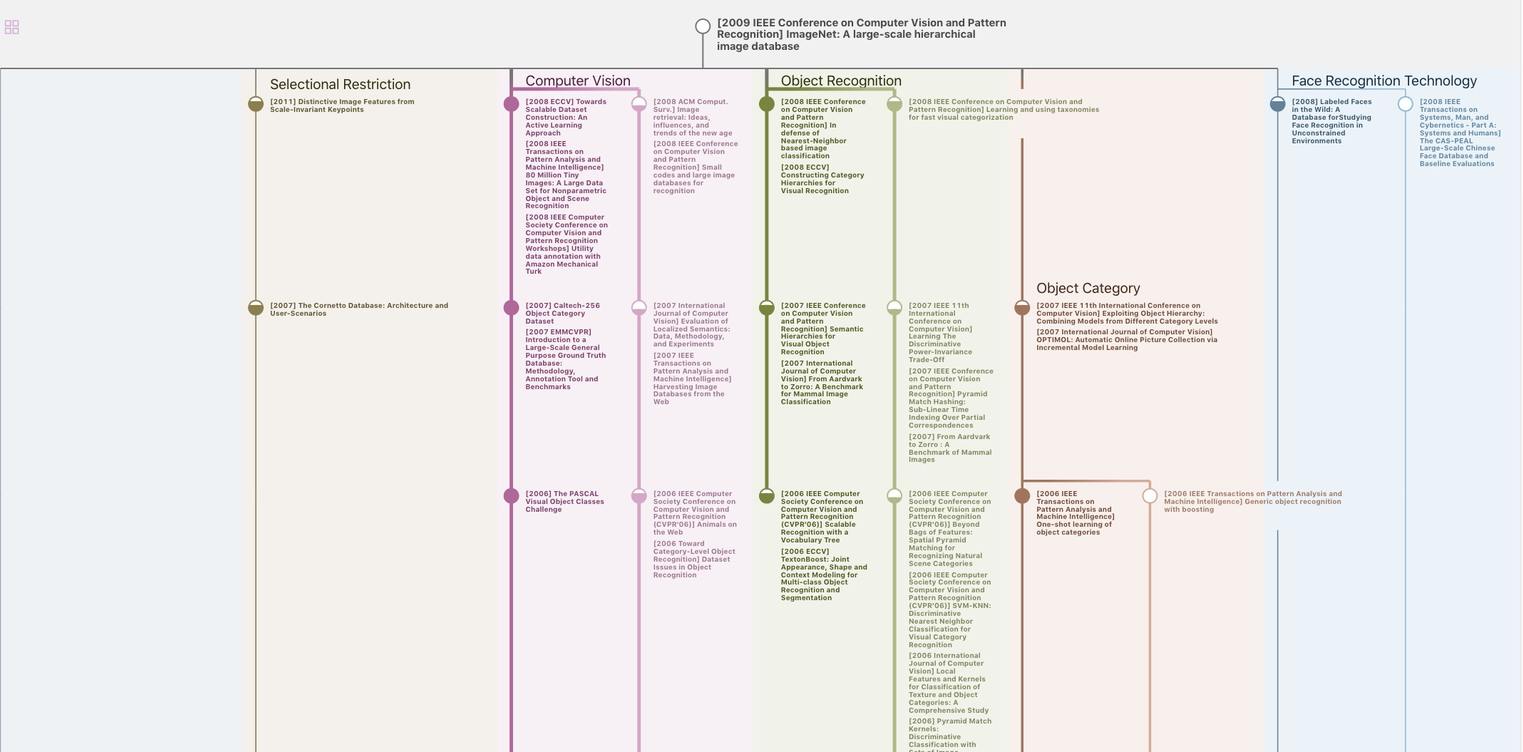
生成溯源树,研究论文发展脉络
Chat Paper
正在生成论文摘要