Manifold Preserving Guided Diffusion
ICLR 2024(2023)
摘要
Despite the recent advancements, conditional image generation still faces
challenges of cost, generalizability, and the need for task-specific training.
In this paper, we propose Manifold Preserving Guided Diffusion (MPGD), a
training-free conditional generation framework that leverages pretrained
diffusion models and off-the-shelf neural networks with minimal additional
inference cost for a broad range of tasks. Specifically, we leverage the
manifold hypothesis to refine the guided diffusion steps and introduce a
shortcut algorithm in the process. We then propose two methods for on-manifold
training-free guidance using pre-trained autoencoders and demonstrate that our
shortcut inherently preserves the manifolds when applied to latent diffusion
models. Our experiments show that MPGD is efficient and effective for solving a
variety of conditional generation applications in low-compute settings, and can
consistently offer up to 3.8x speed-ups with the same number of diffusion steps
while maintaining high sample quality compared to the baselines.
更多查看译文
关键词
generative model,diffusion model,controllable generation
AI 理解论文
溯源树
样例
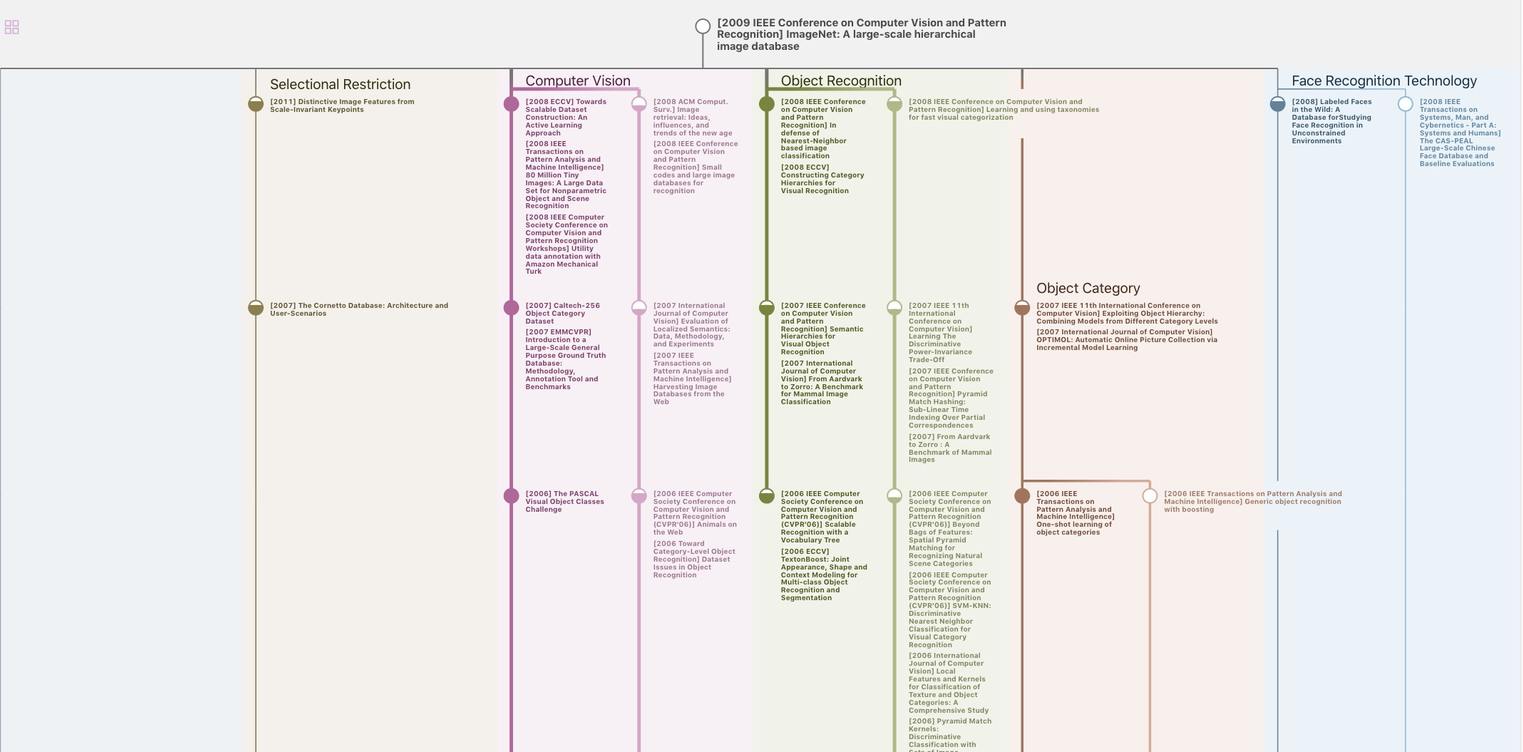
生成溯源树,研究论文发展脉络
Chat Paper
正在生成论文摘要