WAVELET2VEC: A Filter Bank Masked Autoencoder for EEG-Based Seizure Subtype Classification
ICASSP 2023 - 2023 IEEE International Conference on Acoustics, Speech and Signal Processing (ICASSP)(2023)
摘要
Electroencephalogram (EEG) based seizure subtype classification plays an important role in clinical diagnostics. However, existing deep learning approaches face two challenges in such applications: 1) convolutional or recurrent neural network based models have difficulty learning long-term dependencies; and, 2) there are not enough labeled seizure sub-type data for training such models. This paper proposes a Transformer-based self-supervised learning model for EEG-based seizure subtype classification, which copes well with these two challenges. Filter bank analysis is first employed to improve Vision Transformer as a Wavelet Transformer (WaT) encoder, which generates multi-grained feature representations of EEG signals. Then, self-supervised learning is used to pre-train WaT from unlabeled EEG data. Experiments on two public datasets demonstrated that Wavelet2Vec outperformed several other supervised and self-supervised models in cross-subject seizure subtype classification.
更多查看译文
关键词
EEG,seizure subtype classification,filter bank analysis,self-supervised learning,masked autoencoder
AI 理解论文
溯源树
样例
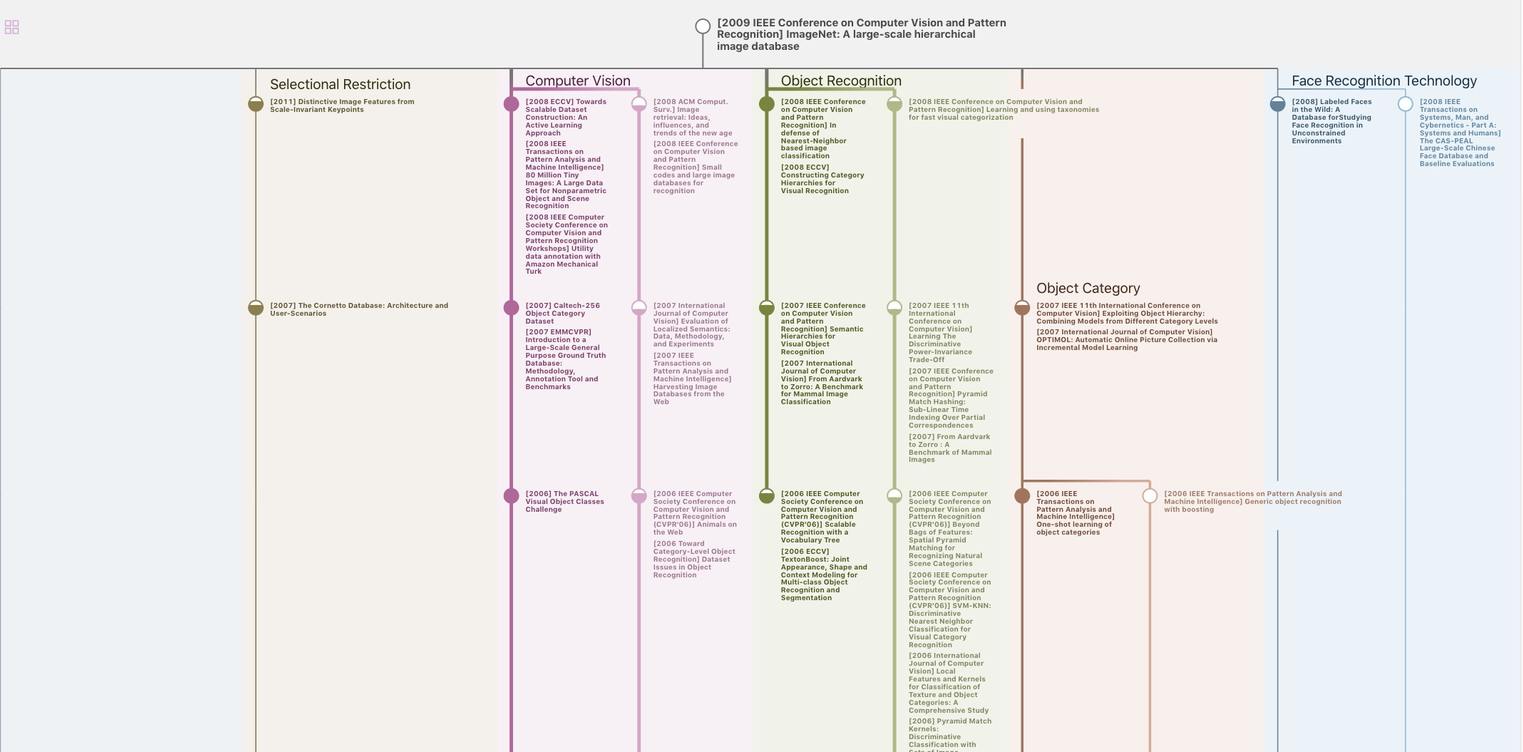
生成溯源树,研究论文发展脉络
Chat Paper
正在生成论文摘要