Agile Radio Map Prediction Using Deep Learning
ICASSP 2023 - 2023 IEEE International Conference on Acoustics, Speech and Signal Processing (ICASSP)(2023)
摘要
In this paper, we introduce a runtime-efficient radio frequency (RF) map prediction method based on UNet convolutional neural networks (CNNs), trained on a large-scale 3D maps dataset. The proposed method calculates the line-of-sight maps and feeds them as input for the UNet CNN. A special Kullback–Leibler divergence loss function is adopted, enabling the proposed method to minimize both error’s mean and variance. The performance of our model is evaluated in the context of the 2023 IEEE ICASSP Signal Processing Grand Challenge, namely, the First Pathloss Radio Map Prediction Challenge. The evaluation results demonstrate that the proposed method achieves an average normalized root-mean-square error (RMSE) of 0.045 with an average of 14 milliseconds (ms) runtime.
更多查看译文
关键词
Radio Map Prediction,UNet,RSS
AI 理解论文
溯源树
样例
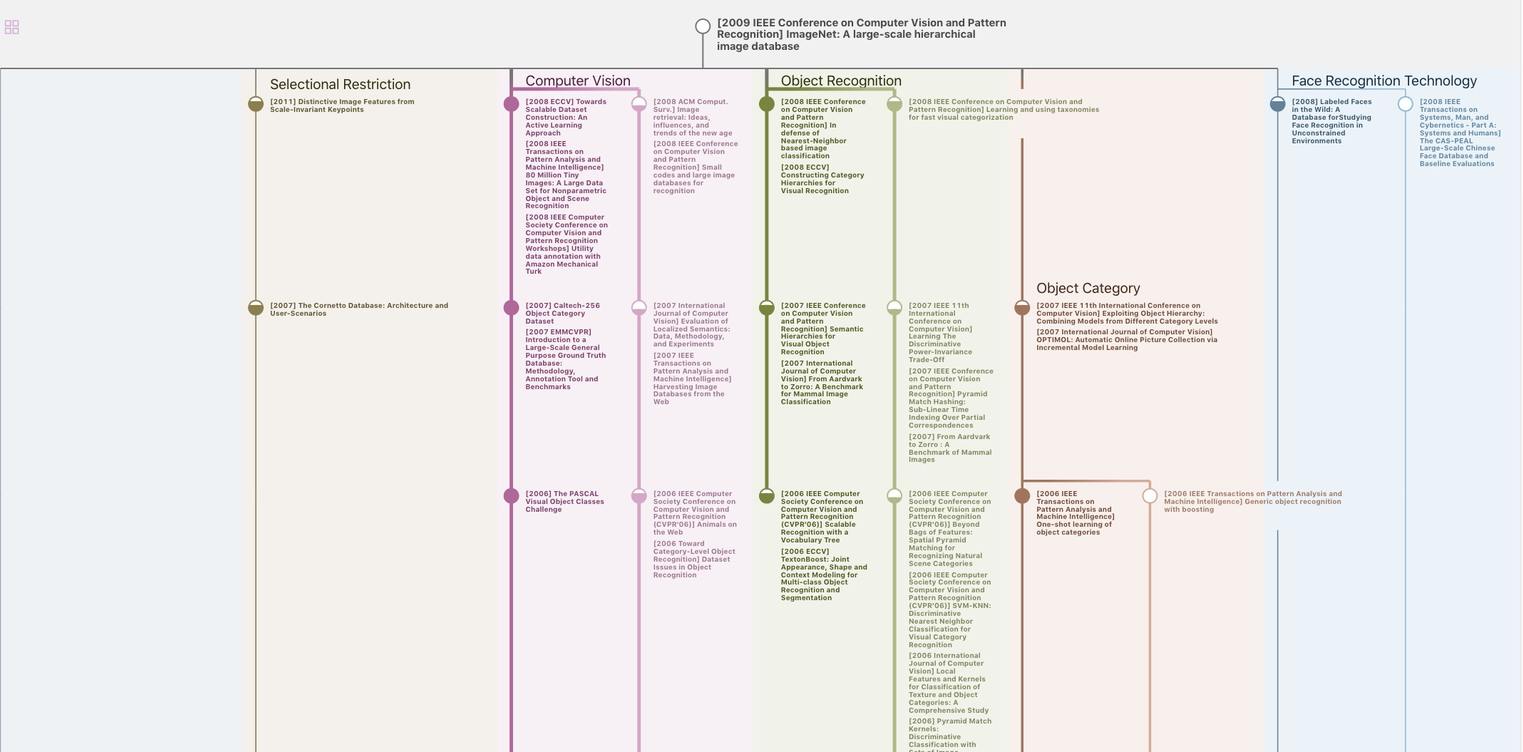
生成溯源树,研究论文发展脉络
Chat Paper
正在生成论文摘要