Low-Dose CT Reconstruction Via Optimization-Inspired GAN
ICASSP 2023 - 2023 IEEE International Conference on Acoustics, Speech and Signal Processing (ICASSP)(2023)
摘要
Most research on Low-dose Computed Tomography (LDCT) reconstruction is designed as a black box, lacking controllability and interpretability. In this paper, a Proximal Linear ADMM framework-based Generative Adversarial Network (PLA-GAN) is proposed. Specifically, without loss of interpretability, channel attention blocks and NonLocal Sparse Attention (NLSA) modules are embedded into two regularizers respectively and iterated alternately, driving the network to cope with real and complex CT image degradation through a multi-scale and adaptive way. To further promote the visual quality, a discriminator containing NLSA module is also introduced. The comparisons with state-of-the-arts on the Mayo dataset validate the superiority of our proposed algorithm both numerically and visually. The advantages of generalizability and interpretability are also evident.
更多查看译文
关键词
Low-dose CT,unfolding,proximal linear ADMM,patch-GAN,sparse attention
AI 理解论文
溯源树
样例
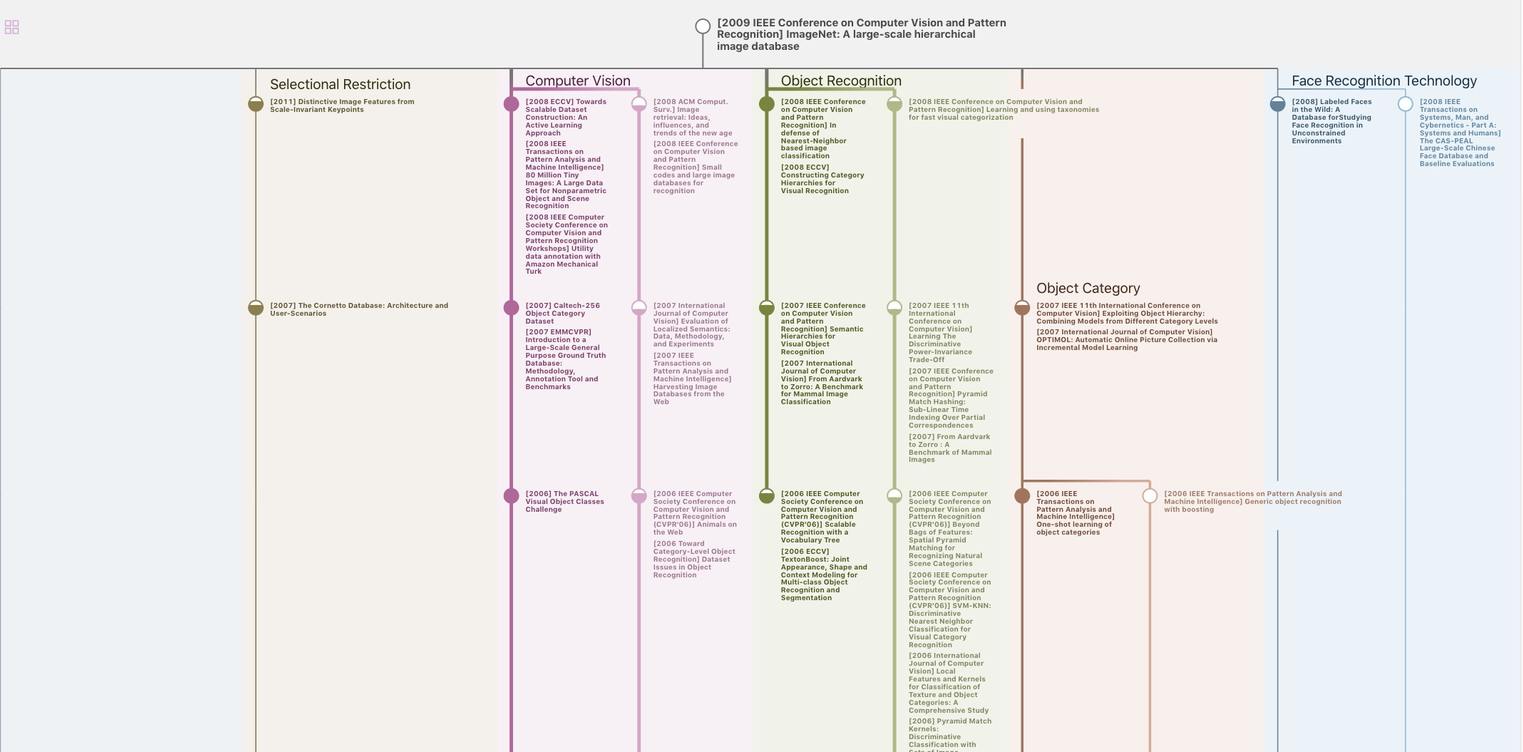
生成溯源树,研究论文发展脉络
Chat Paper
正在生成论文摘要