Building Keyword Search System from End-To-End Asr Systems
ICASSP 2023 - 2023 IEEE International Conference on Acoustics, Speech and Signal Processing (ICASSP)(2023)
摘要
Keyword search (KWS) systems are commonly built on top of existing automatic speech recognition (ASR) systems. However, end-to-end (E2E) ASR models are not naturally equipped with word-level timing information or confidence. Existing methods for re-purposing E2E ASR systems for KWS are largely heuristic or model-specific. In this paper, we describe a general KWS pipeline, applicable to any ASR model that generates N-best lists. We extract timing information using either external word-aligners, or time-preserving weighted finite-state transducer-based decoders. We show that our light-weight, ASR-agnostic approach for confidence estimation based on N-best lists outperforms other commonly used heuristics, such as using the decoder’s softmax probability, and even a more complicated dedicated confidence estimation model (CEM). Finally, we compare our performance to hybrid ASR models, extensively evaluating the impact of word-level timing, confidence, and recall on KWS performance. Our KWS pipeline is available online
1
, suitable for evaluating the aforementioned ASR components as downstream tasks.
更多查看译文
关键词
speech recognition,end-to-end,keyword search,information retrieval,confidence,forced alignment
AI 理解论文
溯源树
样例
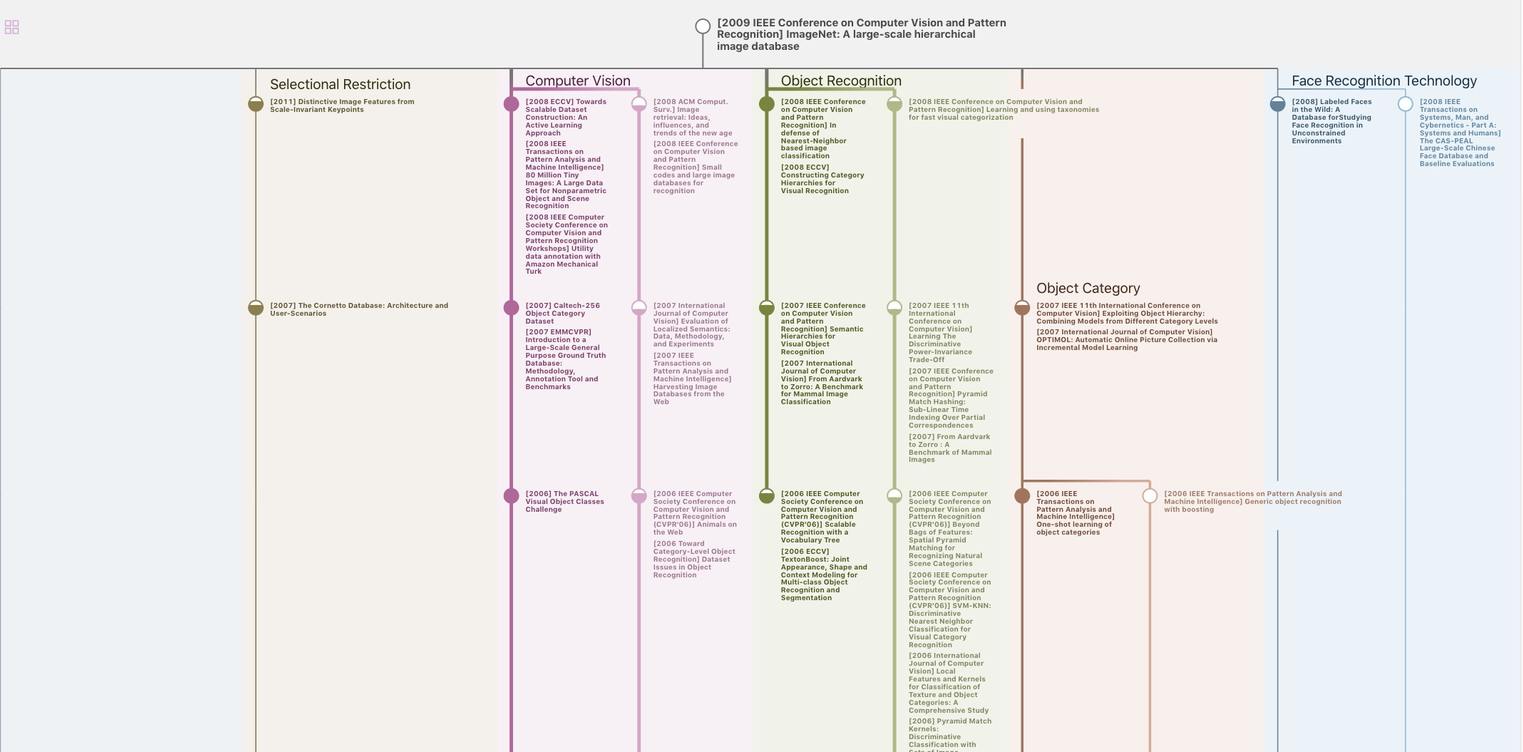
生成溯源树,研究论文发展脉络
Chat Paper
正在生成论文摘要