Geometric Matrix Completion with Collaborative Routing Between Capsules
ICASSP 2023 - 2023 IEEE International Conference on Acoustics, Speech and Signal Processing (ICASSP)(2023)
Abstract
Collaborative filtering (CF) is the most common application of matrix completion, where the user-item rating matrix can be formulated as a bipartite graph. Due to the power of learning on graphs, graph neural networks (GNNs) have been widely adapted to the user-item graph. However, existing graph-based models mainly focus on modeling implicit feedback, while explicit feedback is not fully exploited where the geometric relationships between users and items are latent. Inspired by routing between capsules, we devise a routing algorithm Collaborative Routing for CF to capture these relationships. A novel graph-based matrix completion model based on Collaborative Routing (CRMC) is proposed, which can exploit the user-item graph from both implicit and explicit perspectives to learn better representations, and preserve the relationships between users and items latent in the user-item graph. Experimental results on three real-world datasets demonstrate the effectiveness of our model.
MoreTranslated text
Key words
Matrix Completion,Collaborative Filtering,Capsule Networks
AI Read Science
Must-Reading Tree
Example
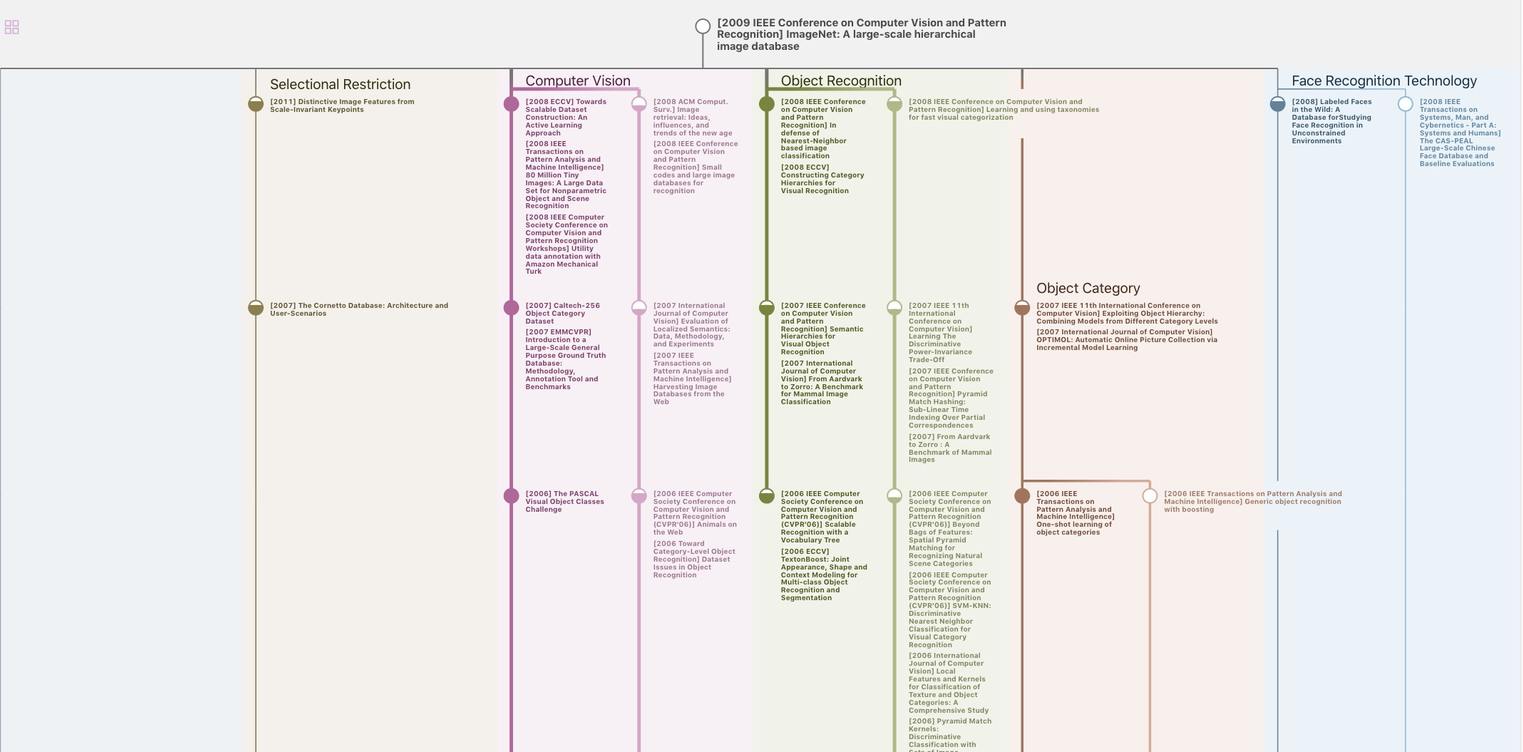
Generate MRT to find the research sequence of this paper
Chat Paper
Summary is being generated by the instructions you defined