Efficient Stuttering Event Detection Using Siamese Networks
ICASSP 2023 - 2023 IEEE International Conference on Acoustics, Speech and Signal Processing (ICASSP)(2023)
摘要
Speech disfluency research is pivotal to accommodating atypical speakers in mainstream conversational technology. However, the lack of publicly available labeled and unlabeled datasets is a significant bottleneck to such research. While many works use pseudo dysfluency data with proxy labels and formulate a self-supervised task, we see merit in using real-world data. In this work, we consolidate the corpora of publicly available speech disfluency datasets with and without labels and propose DisfluentSiam – an efficient siamese network-based small-scale pretraining pipeline using task-specific data from multiple domains with only 10M trainable parameters. We show that with DisfluentSiam, we achieve an average of 15% boost in performance across five types of dysfluency event detection compared to direct wav2vec 2.0 embeddings. In particular, with only 4-5 mins of labeled data for fine-tuning, the DisfluentSiam demonstrates the advantage of task-specific pretraining with up to 25% higher accuracy.
更多查看译文
关键词
Dysfluency,Self-supervised Learning
AI 理解论文
溯源树
样例
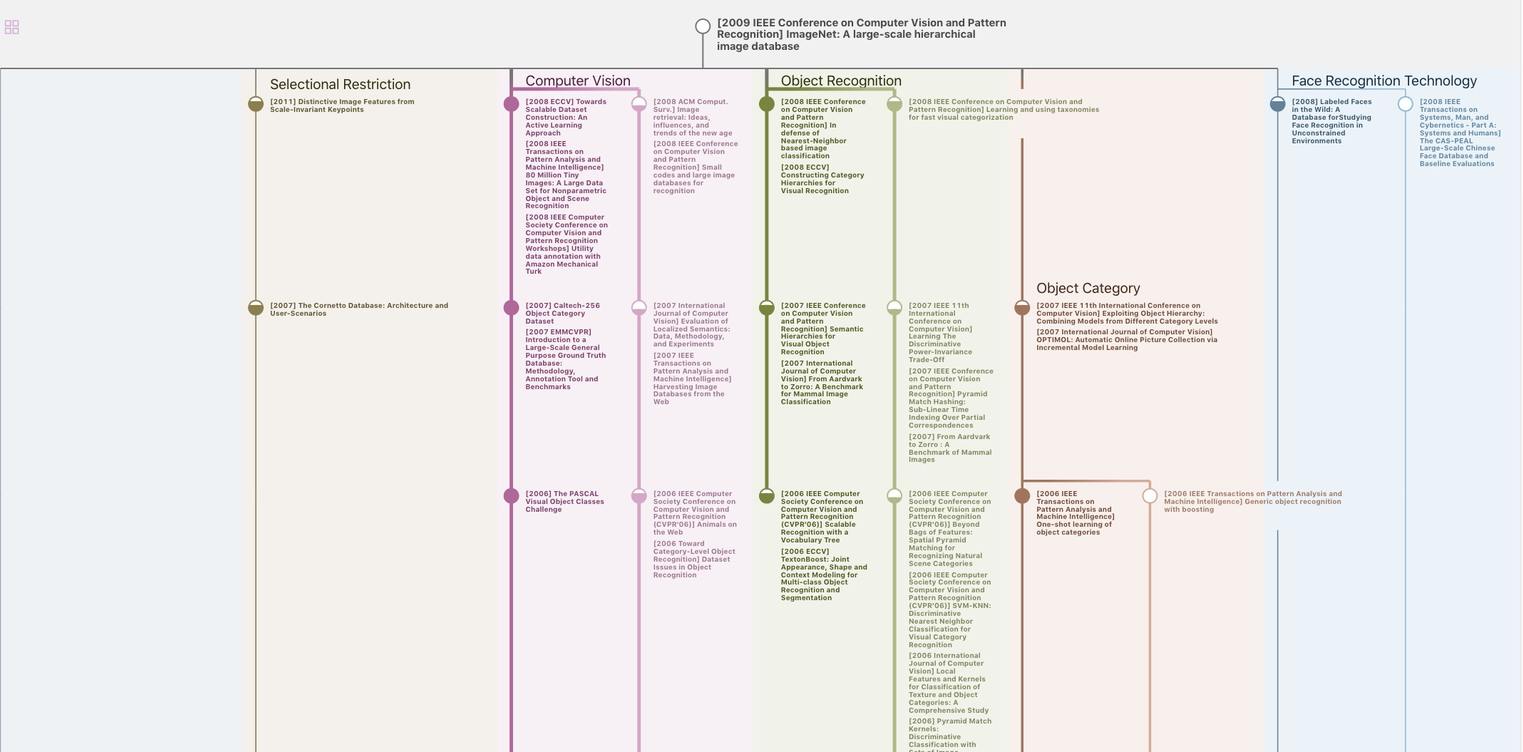
生成溯源树,研究论文发展脉络
Chat Paper
正在生成论文摘要