Low Precision Representations for High Dimensional Models
ICASSP 2023 - 2023 IEEE International Conference on Acoustics, Speech and Signal Processing (ICASSP)(2023)
摘要
The large memory footprint of high dimensional models require quantization to a lower precision for deployment on resource constrained edge devices. With this motivation, we consider the problems of learning a (i) linear regressor, and a (ii) linear classifier from a given training dataset, and quantizing the learned model parameters subject to a pre-specified bit-budget. The error metric is the prediction risk of the quantized model, and our proposed randomized embedding-based quantization methods attain near-optimal error while being computationally efficient. We provide fundamental bounds on the bit-budget constrained minimax risk that, together with our proposed algorithms, characterize the minimum threshold budget required to achieve a risk comparable to the unquantized setting. We also show the efficacy of our strategy by quantizing a two-layer ReLU neural network for non-linear regression. Numerical simulations show the improved performance of our proposed scheme as well as its closeness to the lower bound.
更多查看译文
关键词
Quantization,Randomized Hadamard,Minimax lower bounds,Regression,Classification
AI 理解论文
溯源树
样例
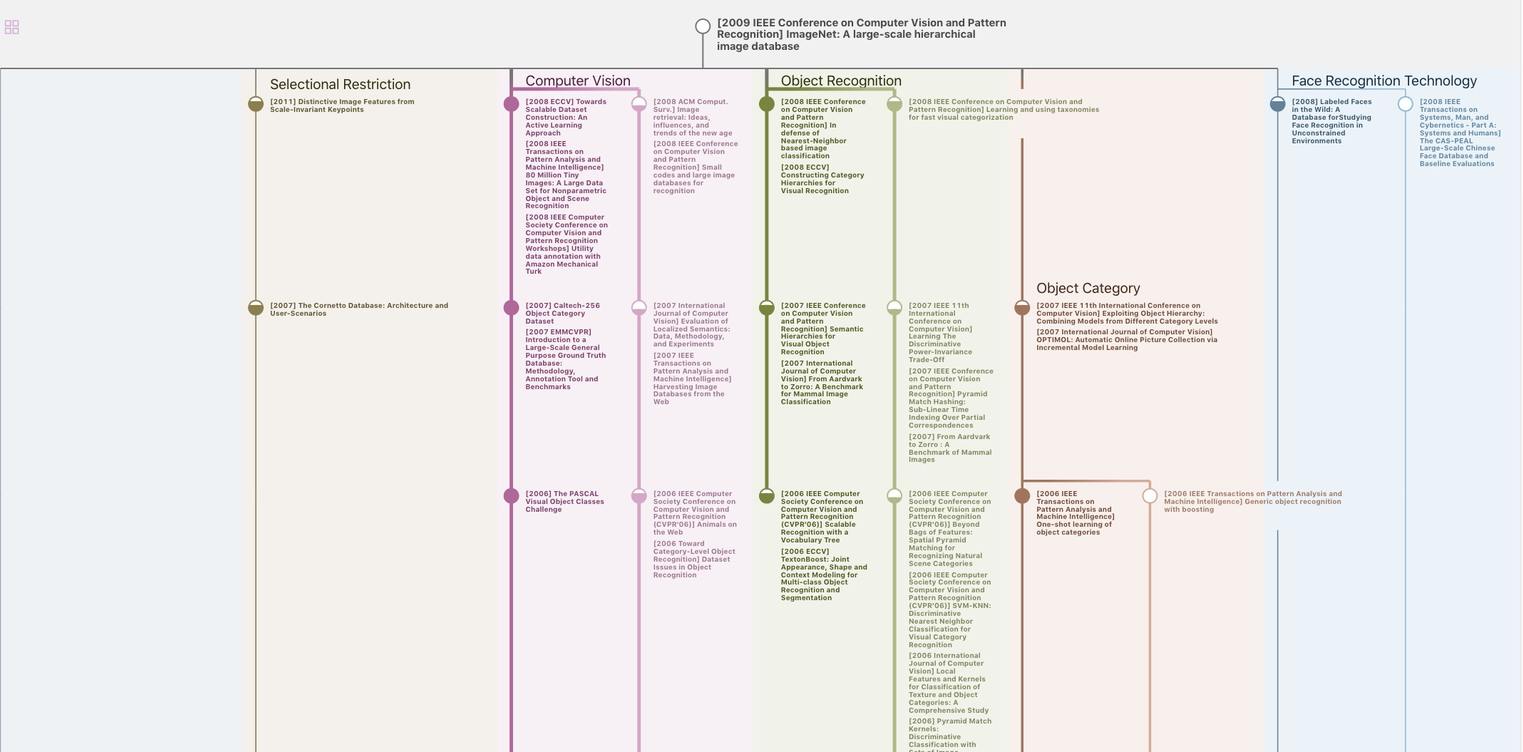
生成溯源树,研究论文发展脉络
Chat Paper
正在生成论文摘要