Exploring Subgroup Performance in End-to-End Speech Models
ICASSP 2023 - 2023 IEEE International Conference on Acoustics, Speech and Signal Processing (ICASSP)(2023)
摘要
End-to-End Spoken Language Understanding models are generally evaluated according to their overall accuracy, or separately on (a priori defined) data subgroups of interest. We propose a technique for analyzing model performance at the subgroup level, which considers all subgroups that can be defined via a given set of metadata and are above a specified minimum size. The metadata can represent user characteristics, recording conditions, and speech targets. Our technique is based on advances in model bias analysis, enabling efficient exploration of resulting subgroups. A fine-grained analysis reveals how model performance varies across sub-groups, identifying modeling issues or bias towards specific subgroups.We compare the subgroup-level performance of models based on wav2vec 2.0 and HuBERT on the Fluent Speech Commands dataset. The experimental results illustrate how subgroup-level analysis reveals a finer and more complete picture of performance changes when models are replaced, automatically identifying the subgroups that most benefit or fail to benefit from the change.
更多查看译文
关键词
End-to-End Speech Representation,Model Bias,Divergence,Subgroup detection
AI 理解论文
溯源树
样例
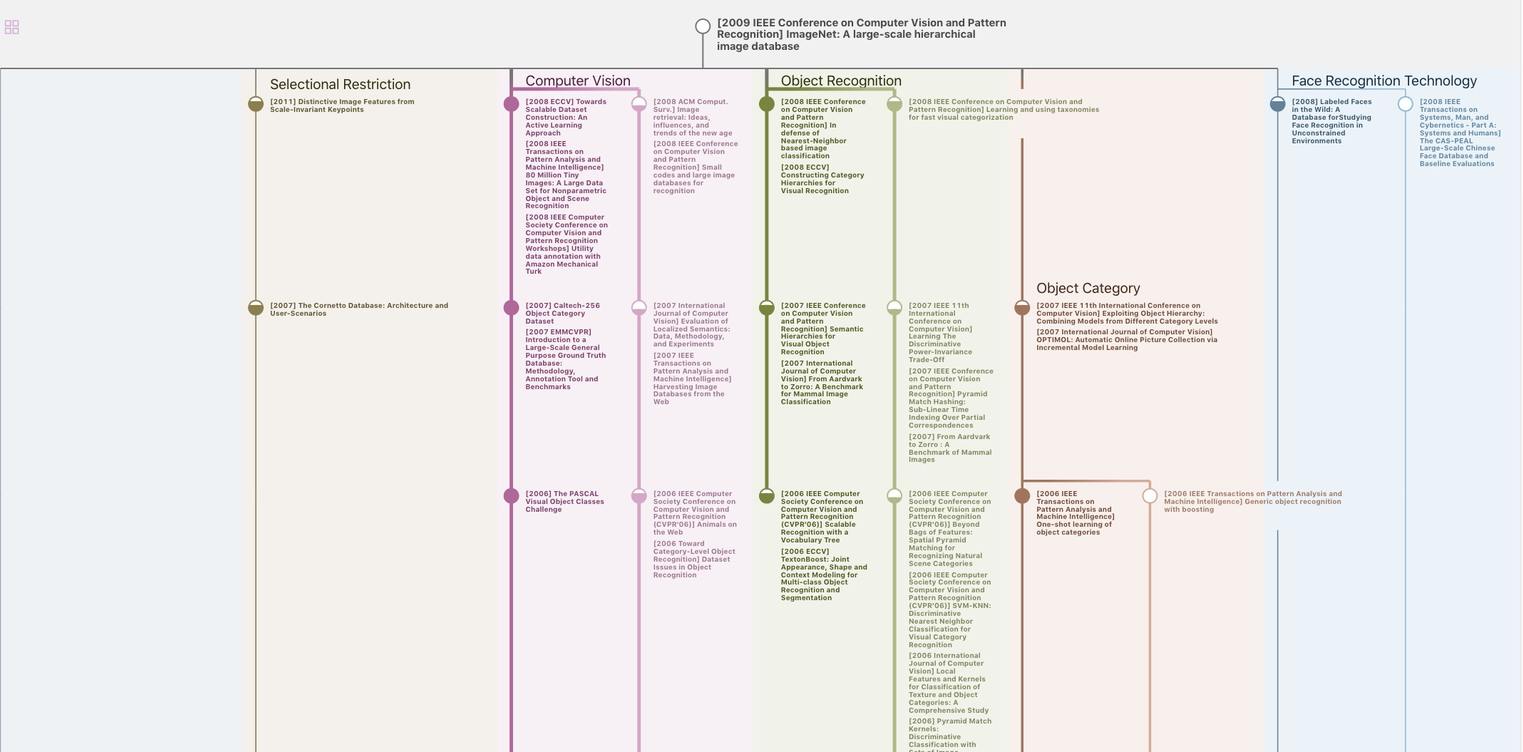
生成溯源树,研究论文发展脉络
Chat Paper
正在生成论文摘要