Cov Loss: Covariance-Based Loss for Deep Face Recognition
ICASSP 2023 - 2023 IEEE International Conference on Acoustics, Speech and Signal Processing (ICASSP)(2023)
摘要
Recently, deep neural networks (DNNs) have emerged as state-of-the-art approaches for various computer vision areas. In this paper, we propose an optimized approach for large-scale face recognition. Our work is motivated through the recent development of deep convolutional neural networks (CNNs) that use different loss functions to learn deep features from face images to perform face recognition. As opposed to previous works, we model Cov loss to optimize deep features along the covariance matrix to enhance discriminative power. We formulate Cov loss to maximize inter-class variance and minimize intra-class variance by optimizing the distance between the deep features and their corresponding class covariances in the Euclidean space. The proposed Cov loss is evaluated on large-scale face recognition problems and present results on LFW, IJB-A Janus, IJB-C Janus, and Celebrity Frontal-Profile (CFP). By optimizing features along both Euclidean and angular spaces, our novel loss function learns more robust feature representations of faces, and improves general performance results comparable to the state-of-the-art results.
更多查看译文
关键词
Face Recognition,Loss Function,Deep Learning
AI 理解论文
溯源树
样例
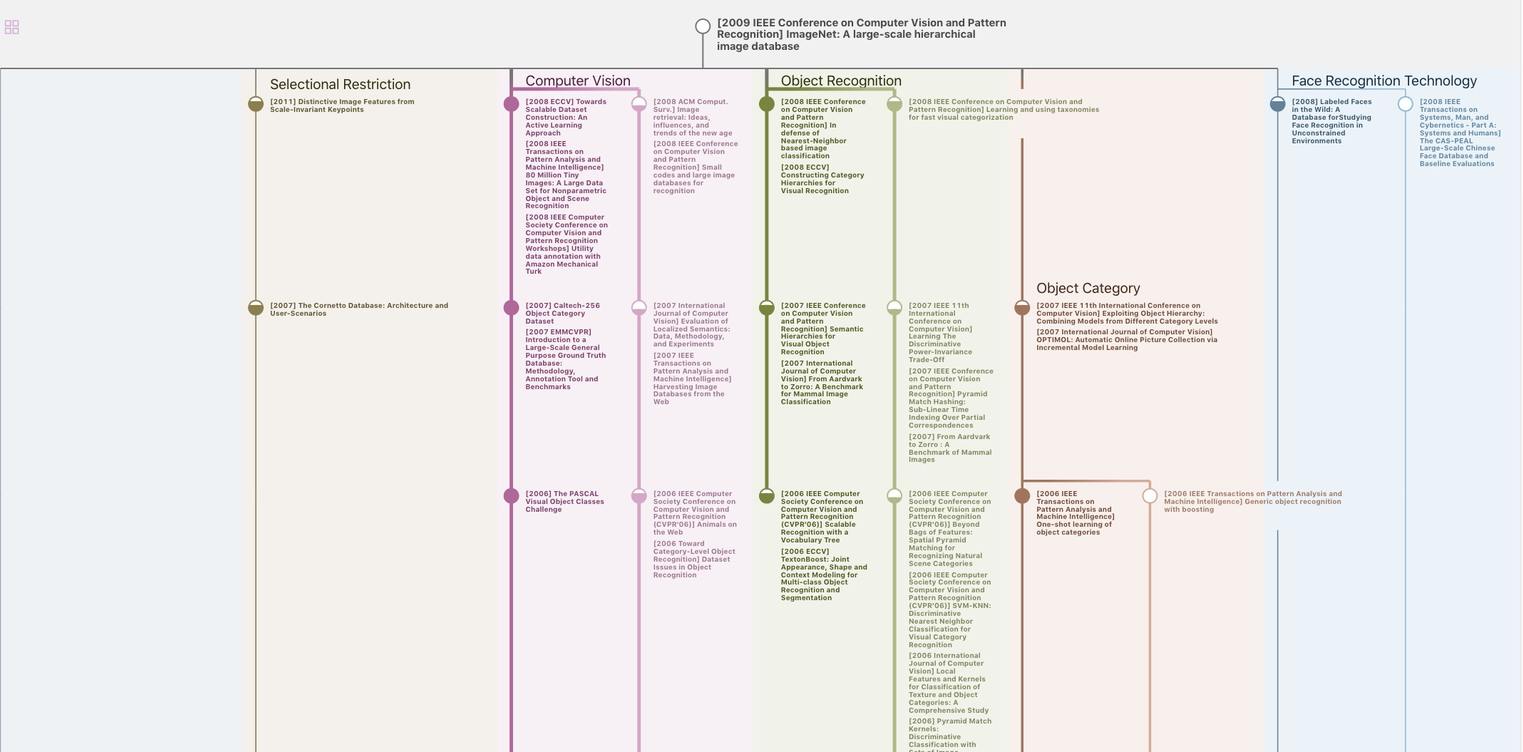
生成溯源树,研究论文发展脉络
Chat Paper
正在生成论文摘要